Pareto Front Upconvert on Multi-objective Building Facility Control Optimization
PROCEEDINGS OF THE 2023 GENETIC AND EVOLUTIONARY COMPUTATION CONFERENCE COMPANION, GECCO 2023 COMPANION(2023)
摘要
This paper verified the effects of a supervised multi-objective optimization algorithm (SMOA) efficiently upconverting the Pareto front representation by utilizing known solutions on a real-world multi-objective building facility control optimization problem. Also, several sampling methods for evaluating promising candidate solutions in SMOA were proposed and compared. Evolutionary variations, such as crossover and mutation involving randomness, are not preferred in practical scenarios, particularly when the objective functions are computationally expensive. In order to suppress obtaining inferior solutions, SMOA constructs the Pareto front and Pareto set estimation models using known solutions, samples promising candidate solutions, and evaluates them. It was reported that SMOA could efficiently generate well-distributed solutions that upconvert the Pareto front representation compared to evolutionary variations with limited solution evaluations in artificial test problems. This paper focuses on the real-world building facility control problem with 15 known solutions, and results show that SMOA can efficiently improve the Pareto front representation compared to evolutionary variations. Also, results show that crowding distance-based one-time sampling considering the distribution of the known solutions achieved the best Pareto front approximation performance in the sampling methods compared in this paper.
更多查看译文
关键词
building facility control,multi-objective optimization,evolutionary optimization,data-driven optimization,supervised multi-objective optimization,Pareto front estimation,Pareto set estimation
AI 理解论文
溯源树
样例
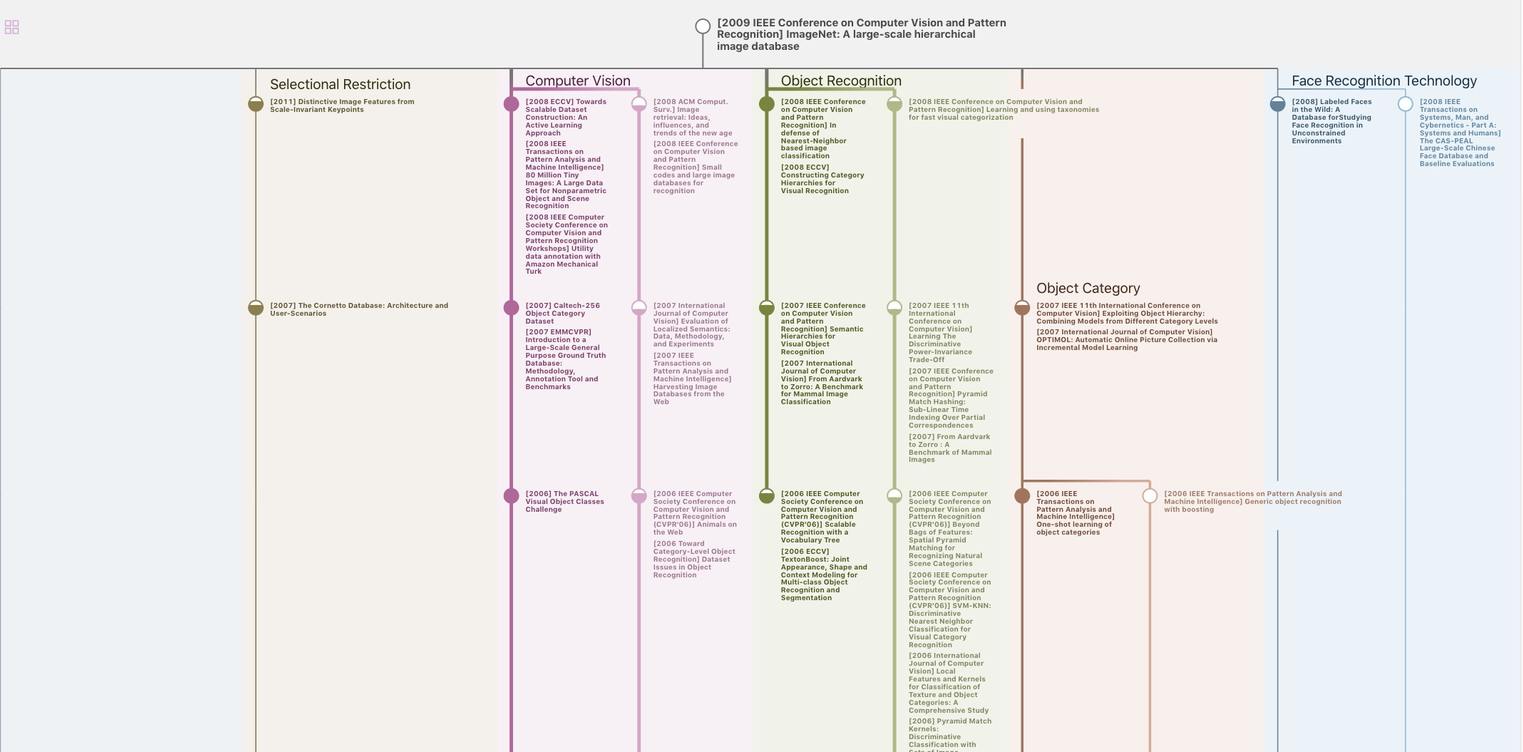
生成溯源树,研究论文发展脉络
Chat Paper
正在生成论文摘要