Stable and Sample-Efficient Policy Search for Continuous Control via Hybridizing Phenotypic Evolutionary Algorithm with the Double Actors Regularized Critics
GECCO(2023)
摘要
Evolutionary Reinforcement Learning arises from hybridizing the sample efficiency of policy gradient with the stability of evolutionary computation. Proximal Distilled Evolutionary Reinforcement Learning (PDERL) implements the hybridization by having information transferred between an RL agent operating alongside a population of candidate policies. PDERL employs two phenotype-based variation operators, behavior distillation crossover and proximal mutation, which exhibit better effectiveness compared to traditional genotype-based operators. We demonstrate that the proximal mutation is sensitive to its mutation magnitude hyperparameter, which yields damaging effects if its value is improperly set. Inspired from Differential Evolution, we propose a novel mutation procedure that operates on action vectors generated by candidate policies. The phenotypic differential mutation (PhDM) shows its stability in diversity maintenance with little disruption. A recently-introduced actor-critic policy gradient algorithm, Double Actors Regularized Critics (DARC), exhibits a superior sample efficiency. DARC alleviates both overestimation and underestimation bias via the usage of two actors for better exploration and a dedicated critic regularization technique. In this paper, we restructure PDERL to incorporate PhDM and the policy gradient mechanism of DARC. Experimental results show that our Phenotypic Evolutionary DARC (PhEDARC) outperforms both PDERL and DARC in four control tasks from OpenAI Gym. Ablation studies support our design choices.
更多查看译文
关键词
evolutionary reinforcement learning,variation operators,policy search,continuous control
AI 理解论文
溯源树
样例
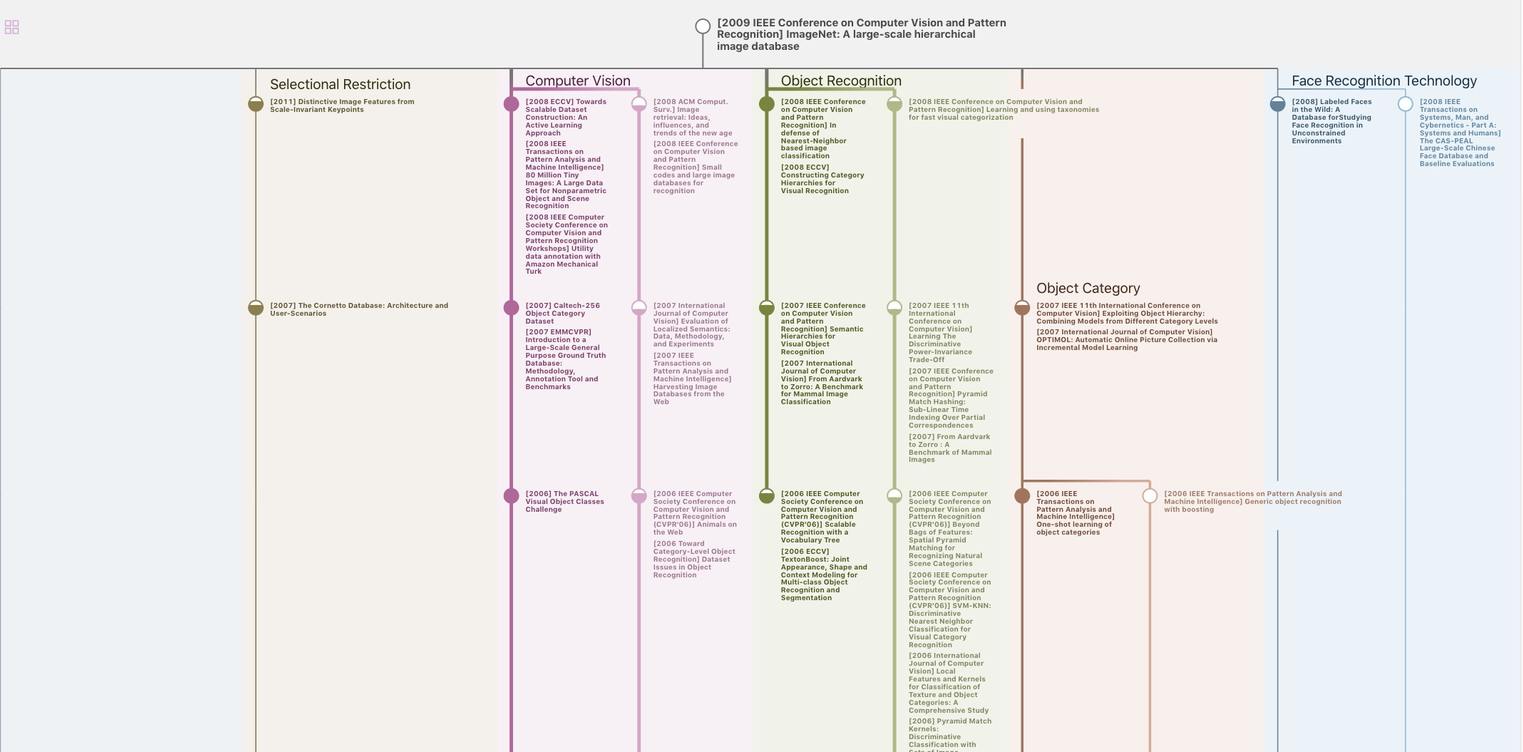
生成溯源树,研究论文发展脉络
Chat Paper
正在生成论文摘要