An Evolutionary Reinforcement Learning Scheme for IoT Robustness.
CSCWD(2023)
摘要
With the rapid scale expansion of the Internet of Things (IoT), the probability of system failure increases. Frequent system failures degrade the quality of service (QoS) of IoT. Existing optimization strategies utilize reinforcement learning (RL) to enhance the robustness of IoT topology. However, due to the increasing scale of the IoT environment, the unbalanced exploration and exploitation of RL agents make it prone to premature convergence at the local optimum. Large-scale action spaces and state spaces lead to a sparse reward problem, which reduces the convergence efficiency of the algorithm. This paper proposes an evolutionary reinforcement learning scheme for IoT robustness to solve the above problems. We design a multi- agent evolution mechanism to provide multiple experiences for RL, which strengthens exploration capability. We present new evolution operators to promote convergence, which combine dis- tillation crossover and Gaussian mutation. Extensive experiments show that our scheme has a strong exploration capability, and the optimization rate of IoT topology robustness reaches 81.15%, which outperforms other robustness optimization algorithms.
更多查看译文
关键词
Internet of Things,network topology,robustness optimization,evolutionary reinforcement learning
AI 理解论文
溯源树
样例
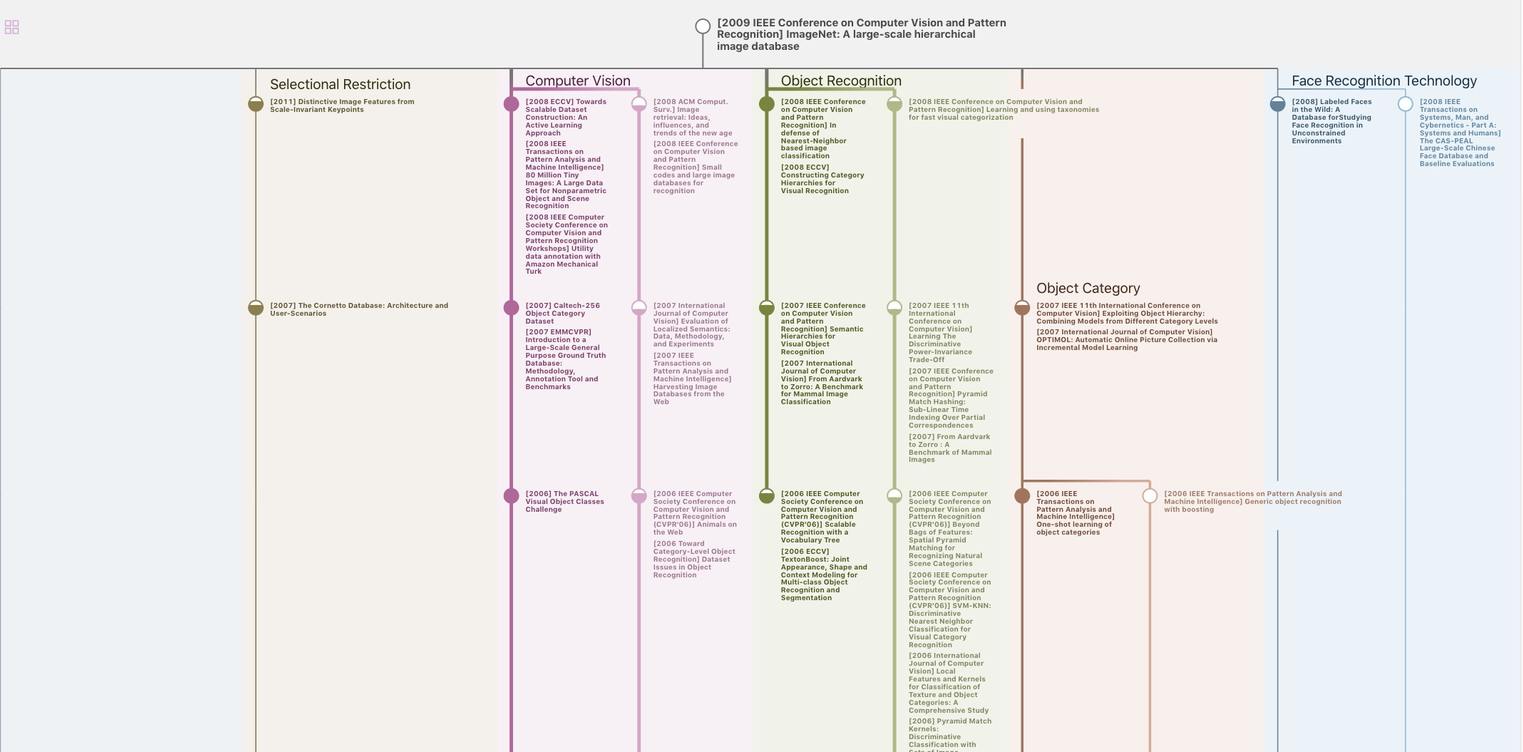
生成溯源树,研究论文发展脉络
Chat Paper
正在生成论文摘要