Security Verification Software Platform of Data-efficient Image Transformer Based on Fast Gradient Sign Method
38TH ANNUAL ACM SYMPOSIUM ON APPLIED COMPUTING, SAC 2023(2023)
摘要
Recently, research using knowledge distillation in artificial intelligence (AI) has been actively conducted. In particular, data-efficient image transformer (DeiT) is a representative transformer model using knowledge distillation in image classification. However, DeiT's safety against the patch unit's adversarial attacks was not verified. Furthermore, existing DeiT research did not prove security robustness against adversarial attacks. In order to verify the vulnerability of adversarial attacks, we conducted an attack using the fast gradient sign method (FGSM) targeting the DeiT model based on knowledge distillation. As a result of the experiment, an accuracy of 93.99% was shown in DeiT verification based on Normal data (Cifar-10). In contrast, when verified with abnormal data based on FGSM (adversarial examples), the accuracy decreased by 83.49% to 10.50%. By analyzing the vulnerability pattern related to adversarial attacks, we confirmed that FGSM showed successful attack performance through weight control of DeiT. Moreover, we verified that DeiT has security limitations for practical application.
更多查看译文
关键词
Deit,Knowledge distillation,Adversarial attacks,FGSM
AI 理解论文
溯源树
样例
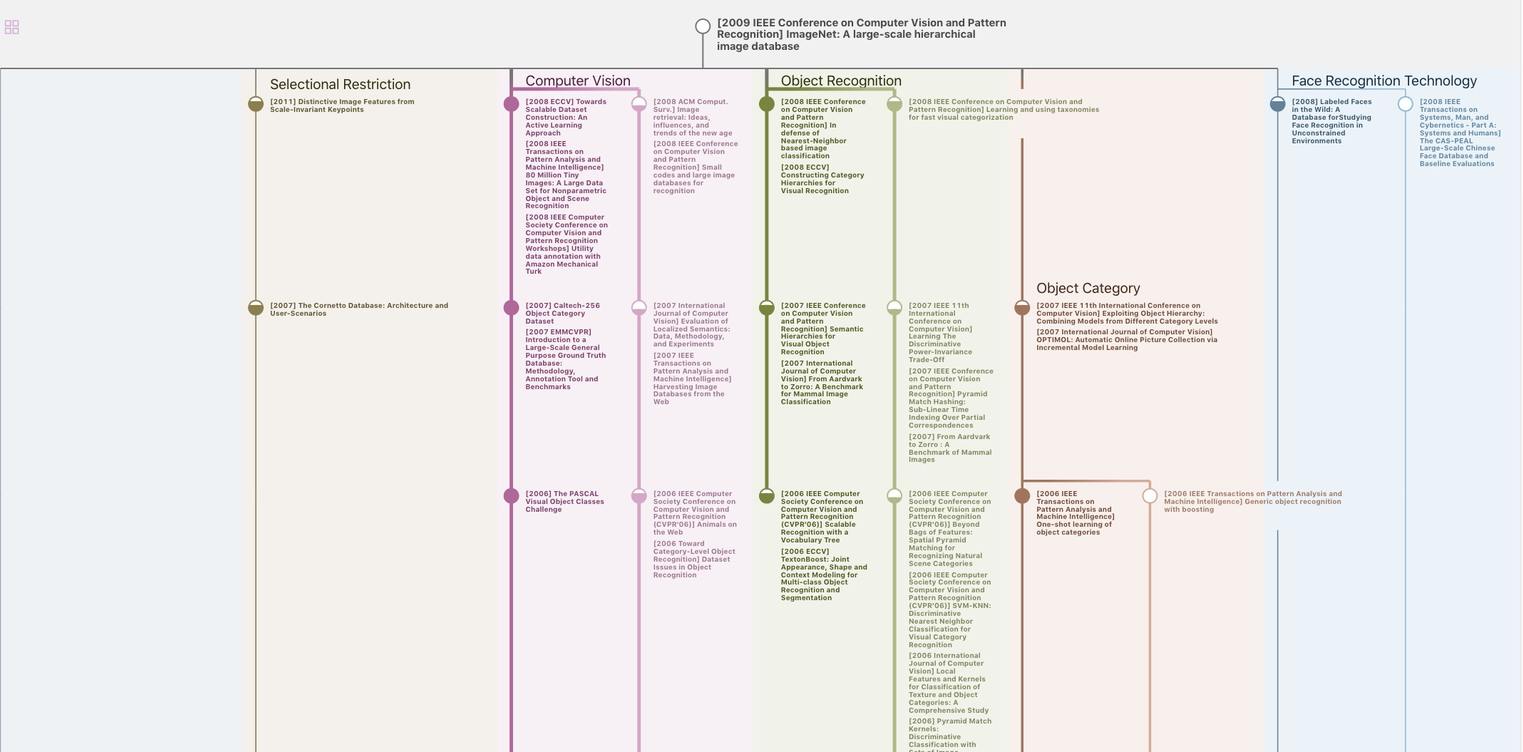
生成溯源树,研究论文发展脉络
Chat Paper
正在生成论文摘要