Towards Principled Synthetic Benchmarks for Explainable Rule Set Learning Algorithms
PROCEEDINGS OF THE 2023 GENETIC AND EVOLUTIONARY COMPUTATION CONFERENCE COMPANION, GECCO 2023 COMPANION(2023)
摘要
A very common and powerful step in the design process of a new learning algorithm or extensions and improvements of existing algorithms is the benchmarking of models produced by said algorithm. We propose a paradigm shift in the benchmarking of explainable if-then-rule-based models like the ones generated by Learning Classifier Systems or Fuzzy Rule-based Systems. The principled method we suggest is based on synthetic data sets being sampled from randomly generated but known processes that have the same overall structure as the models that are being benchmarked (i. e. each process consists of a set of components each of which corresponds to one if-then rule) which is up-to-date not available among the many synthetic data generators. This approach has several benefits over other benchmarks and we expect that it will lead to fresh insights into how algorithms producing such explainable models work and can be improved. We demonstrate its usage by benchmarking the effects of different rule representations in the XCSF classifier system.
更多查看译文
关键词
Rule Set Learning,Learning Classifier Systems,Fuzzy Rule-based Systems,Benchmarking,Evolutionary Machine Learning
AI 理解论文
溯源树
样例
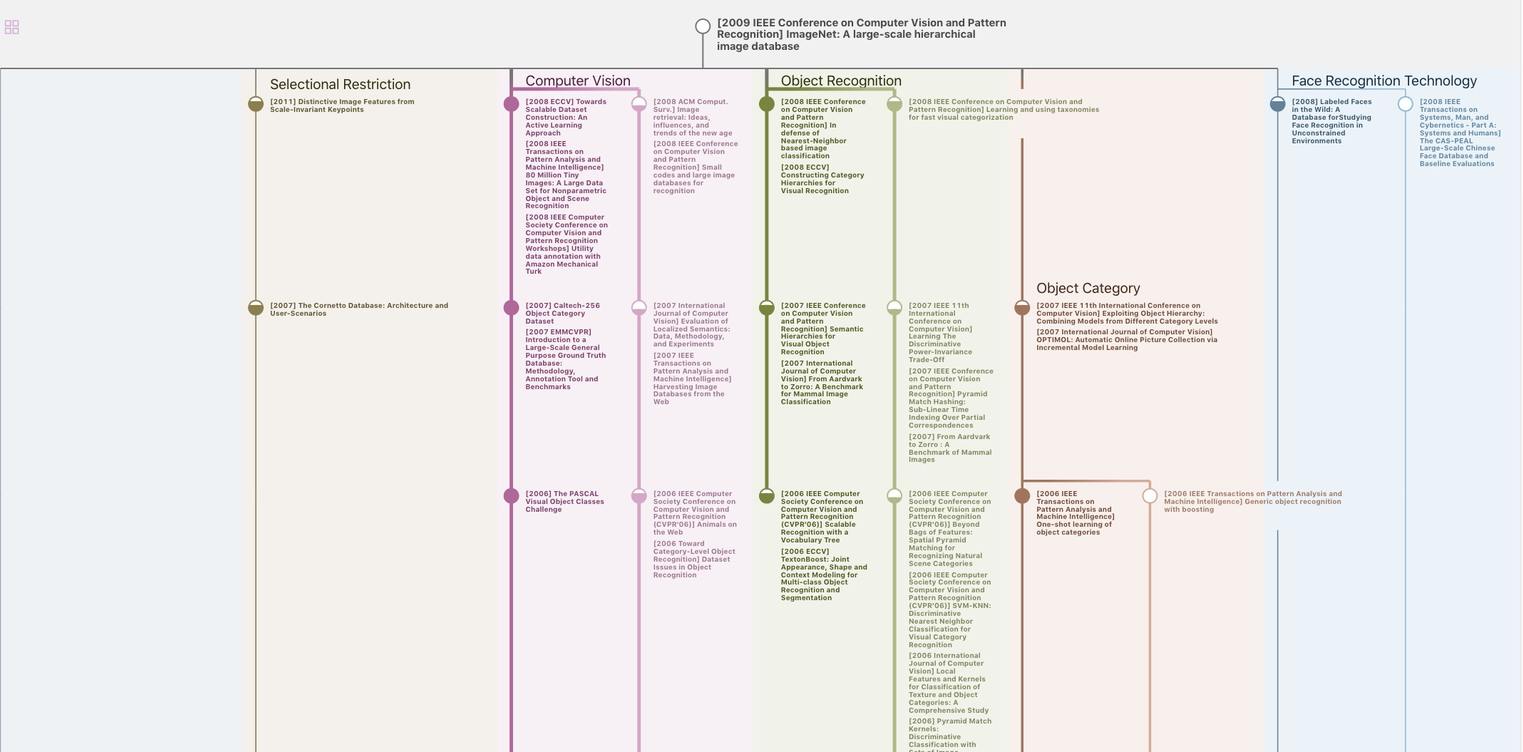
生成溯源树,研究论文发展脉络
Chat Paper
正在生成论文摘要