Comparing Individual Representations in Grammar-Guided Genetic Programming for Glucose Prediction in People with Diabetes
PROCEEDINGS OF THE 2023 GENETIC AND EVOLUTIONARY COMPUTATION CONFERENCE COMPANION, GECCO 2023 COMPANION(2023)
摘要
The representation of individuals in Genetic Programming (GP) has a large impact on the evolutionary process. In this work, we investigate the evolutionary process of three Grammar-Guided GP (GGGP) methods, Context-Free Grammars GP (CFG-GP), Grammatical Evolution (GE) and Structured Grammatical Evolution (SGE), in the context of the complex, real-world problem of predicting the glucose level of people with diabetes two hours ahead of time. Our analysis differs from previous analyses by (1) comparing all three methods on a complex benchmark, (2) implementing the methods in the same framework, allowing a fairer comparison, and (3) analyzing the evolutionary process outside of performance. We conclude that representation choice is more impactful with a higher maximum depth, and that CFG-GP better explores the search space for deeper trees, achieving better results. Furthermore, we find that CFG-GP relies more on feature construction, whereas GE and SGE rely more on feature selection. Finally, we altered the GGGP methods in two ways: using is an element of-lexicase selection, which solved the overfitting problem of CFG-GP; and with a penalization of complex trees, to create more interpretable trees. Combining is an element of-lexicase selection with CFG-GP performed best.
更多查看译文
关键词
Grammar-Guided Genetic Programming,Individual representations,Symbolic Regression
AI 理解论文
溯源树
样例
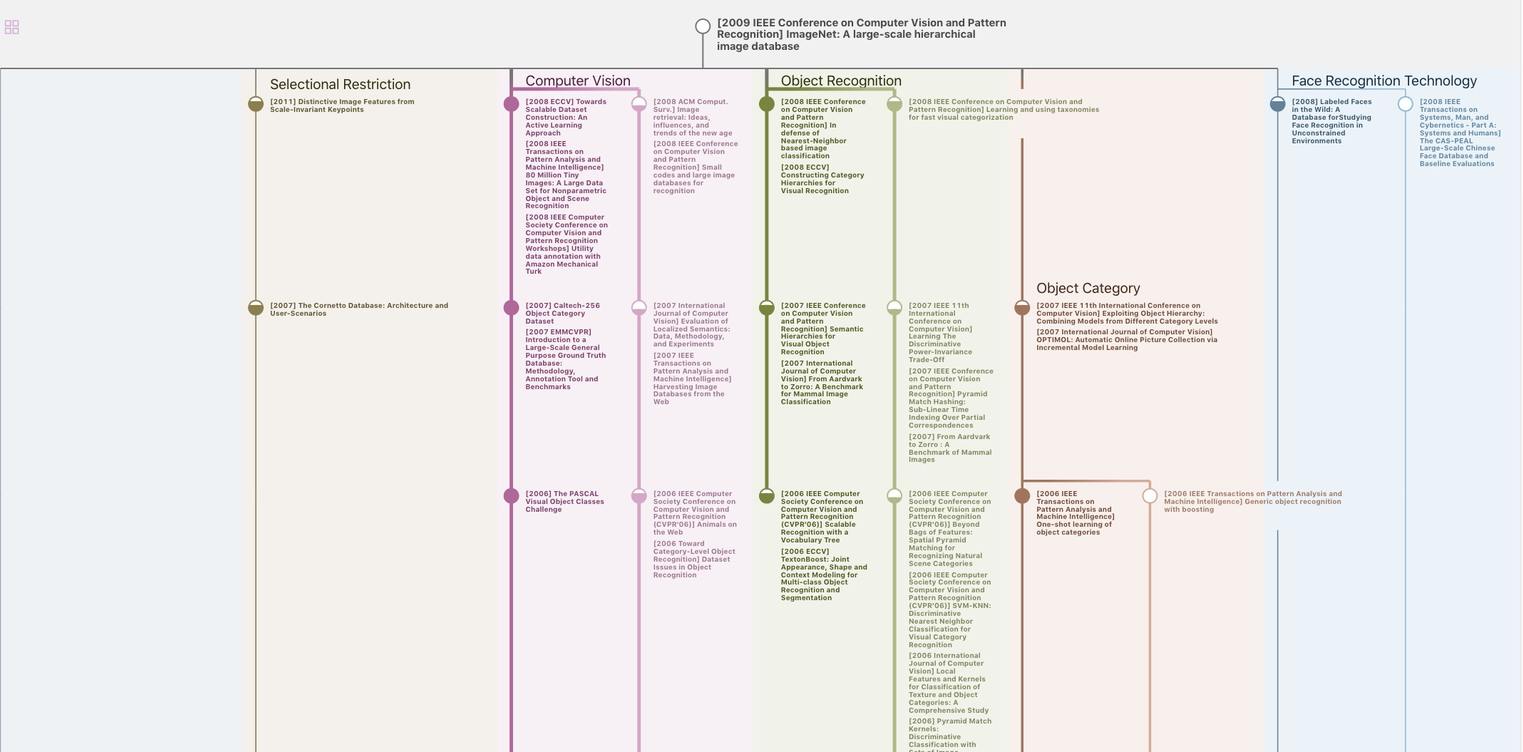
生成溯源树,研究论文发展脉络
Chat Paper
正在生成论文摘要