Designing Convolutional Neural Networks using Surrogate assisted Genetic Algorithm for Medical Image Classification
PROCEEDINGS OF THE 2023 GENETIC AND EVOLUTIONARY COMPUTATION CONFERENCE COMPANION, GECCO 2023 COMPANION(2023)
摘要
Recently, Deep Learning (DL) algorithms have shown state-of-the-art performance in numerous tasks. The design of DL algorithms is time-consuming process that requires expert-level knowledge. To overcome this problem, Neural Architecture Search (NAS) is proposed, which automatically searches for the best neural network architecture for a given task. Indeed, evolutionary NAS has emerged as a widely studied research area, in which evolutionary algorithms are used to design neural networks. In this study, we proposed a novel encoding scheme to represent Convolutional Neural Network (CNN) architectures using continuous representation, which provides a larger search space and potentially finds better solutions compared to discrete representations. Moreover, it allows better exploitation of genetic operators, leading to a quick convergence of individuals. Furthermore, in order to reduce the computational time of the evaluation phase during the search process, surrogate models are used to provide an alternative objective function. To evaluate the effectiveness of the proposal, experiments are performed on CIFAR-10 and multiple datasets from the MedMNIST benchmark. The experimental results demonstrated the effectiveness of the proposed approach compared to several existing state-of-the-art algorithms.
更多查看译文
关键词
Neural Architecture Search,AutoML,AutoDL,Deep Learning
AI 理解论文
溯源树
样例
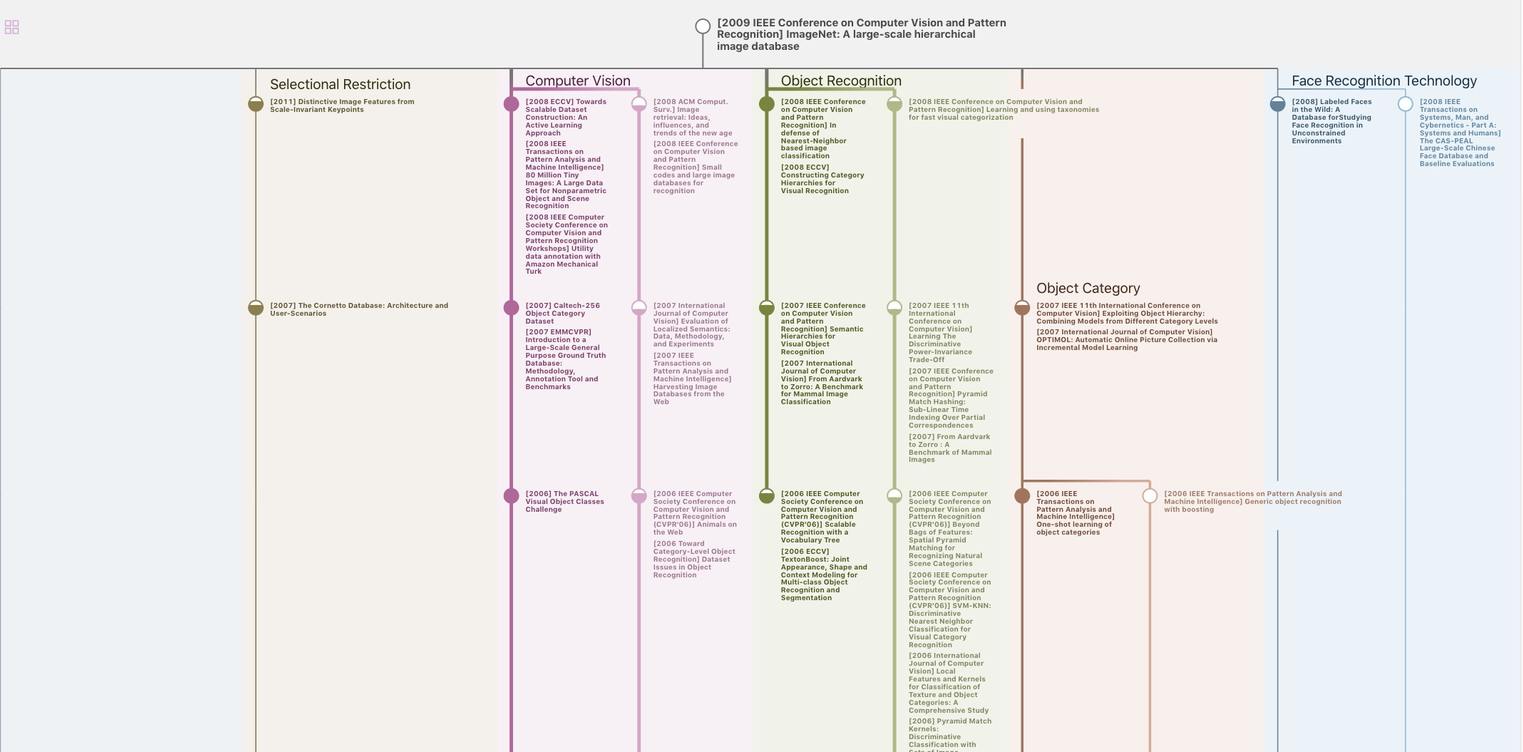
生成溯源树,研究论文发展脉络
Chat Paper
正在生成论文摘要