Aging and rejuvenating strategies for fading windows in multi-label classification on data streams
38TH ANNUAL ACM SYMPOSIUM ON APPLIED COMPUTING, SAC 2023(2023)
摘要
Combining the challenges of streaming data and multi-label learning, the task of mining a drifting, multi-label data stream requires methods that can accurately predict labelsets, adapt to various types of concept drift and run fast enough to process each data point before the next arrives. To achieve greater accuracy, many multi-label algorithms use computationally expensive techniques, such as multiple adaptive windows, with little concern for runtime and memory complexity. We present Aging and Rejuvenating kNN (ARkNN) which uses simple resources and efficient strategies to weight instances based on age, predictive performance, and similarity to the incoming data. We break down ARkNN into its component strategies to show the impact of each and experimentally compare ARkNN to seven state-of-the-art methods for learning from multi-label data streams. We demonstrate that it is possible to achieve competitive performance in multi-label classification on streams without sacrificing runtime and memory use, and without using complex and computationally expensive dual memory strategies.
更多查看译文
关键词
Machine Learning,Data Streams,Multi-label Learning
AI 理解论文
溯源树
样例
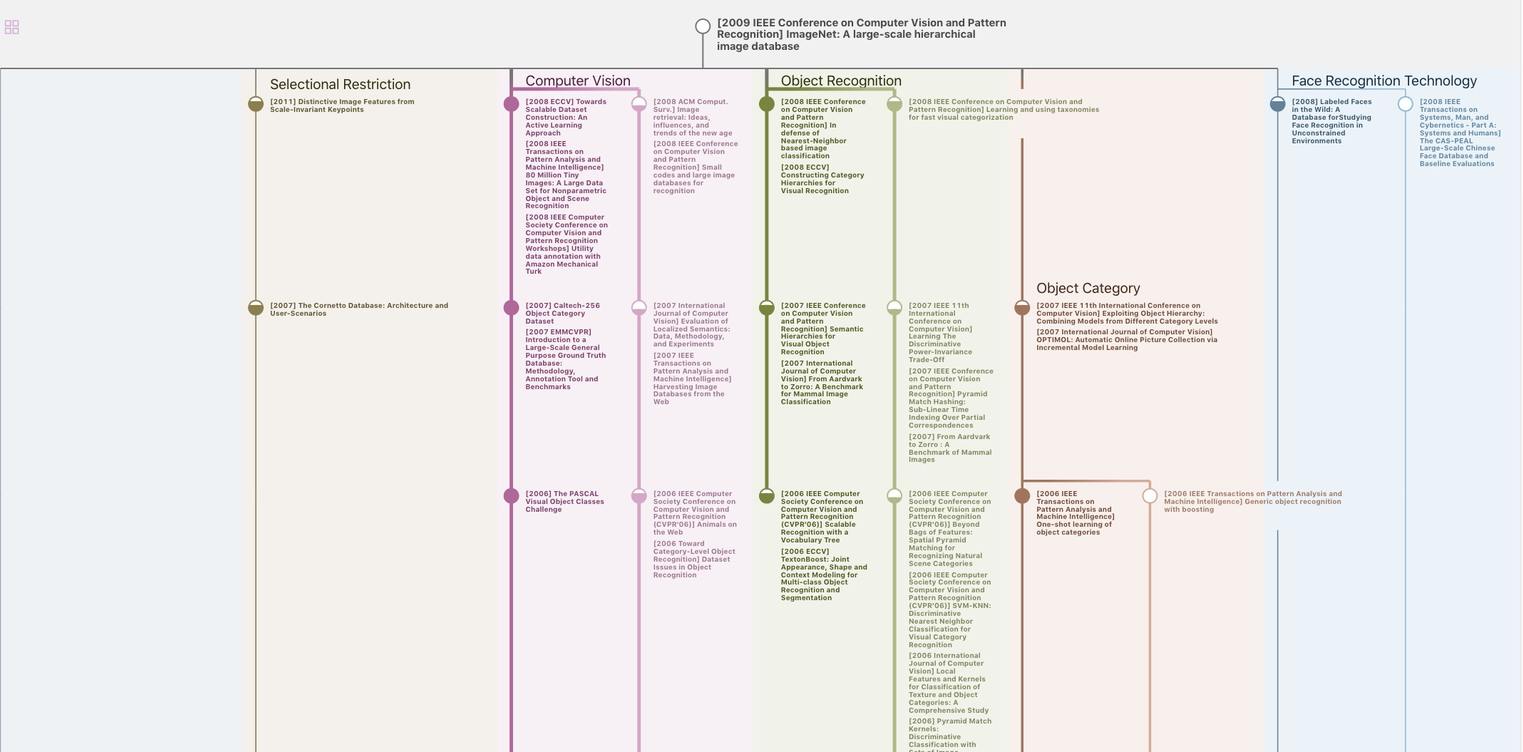
生成溯源树,研究论文发展脉络
Chat Paper
正在生成论文摘要