GP with Ranging-Binding Technique for Symbolic Regression
PROCEEDINGS OF THE 2023 GENETIC AND EVOLUTIONARY COMPUTATION CONFERENCE COMPANION, GECCO 2023 COMPANION(2023)
摘要
This paper proposes a model-based genetic programming algorithm for symbolic regression, called the ranging-binding genetic programming algorithm (RBGP). The goal is to allow offspring to retain the superiority of their promising parents during evolution. Inspired by the concept of model building, RBGP makes use of syntactic information and semantics information in a program to capture the hidden patterns. When compared with GP-GOMEA, ellynGP, and gplearn, RBGP outperformed the others on average in the Penn machine learning benchmarks, RBGP achieving statistically significant improvements over all other methods on 44% of the problems.
更多查看译文
关键词
genetic programming,evolutionary computation
AI 理解论文
溯源树
样例
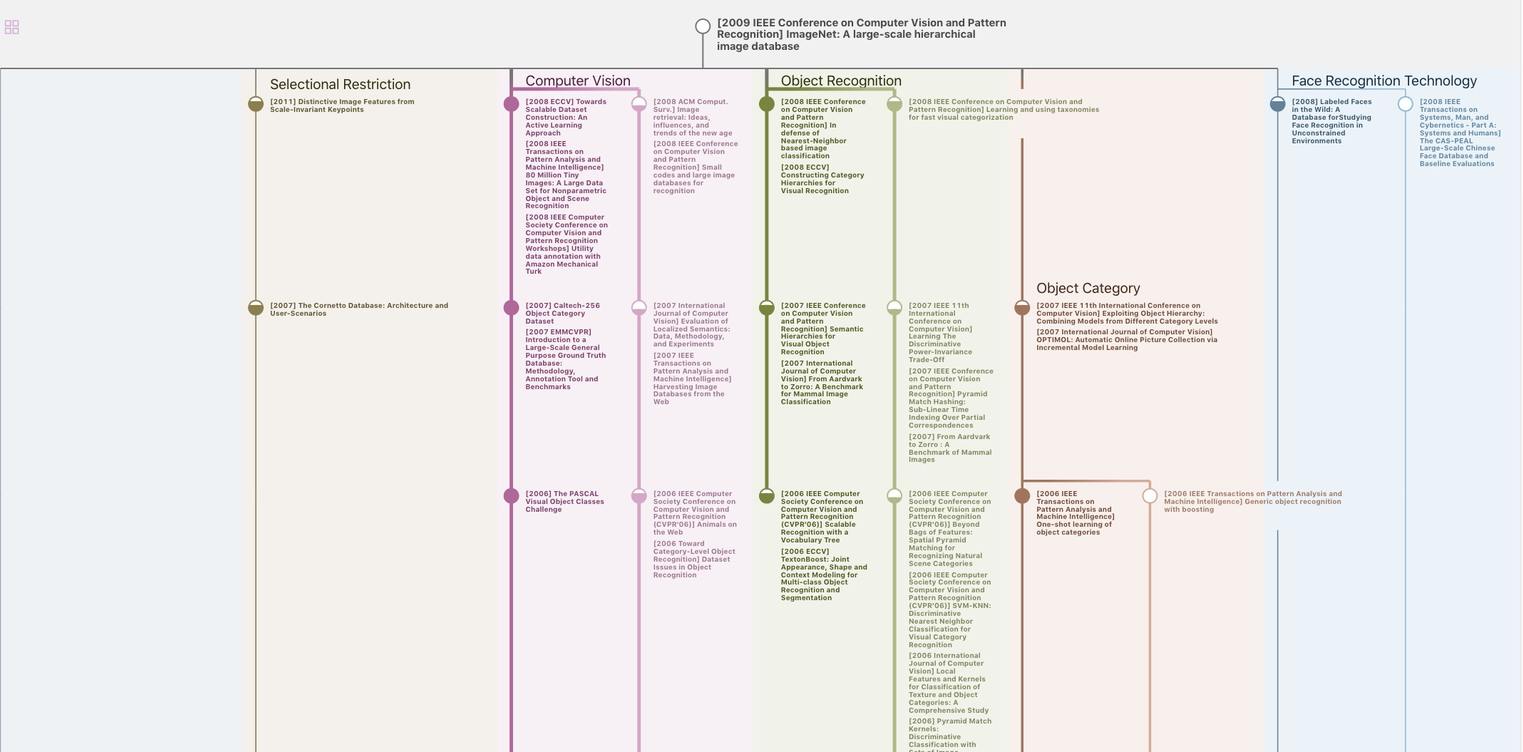
生成溯源树,研究论文发展脉络
Chat Paper
正在生成论文摘要