Performance Analysis of Hybrid Sampling and Meta-heuristics
PROCEEDINGS OF THE 2023 GENETIC AND EVOLUTIONARY COMPUTATION CONFERENCE COMPANION, GECCO 2023 COMPANION(2023)
摘要
This paper investigates the effect of hybridising sampling algorithms with population-based meta-heuristics. Recent literature has shown that alternatives to the traditionally used pseudo-random number generators to generate the initial population of metaheuristics can improve performance. However, most studies focus on sample sizes that are limited to the size of the initial populations. In contrast, this paper studies the effect of extended random initialisation, which uses relatively large samples and then initialises the meta-heuristics from the points in the sample with the best-found fitness values. A portfolio of three meta-heuristics, four sampling algorithms and three different sampling budgets are analysed from the fixed budget perspective on the BBOB benchmark suite. Statistical analysis of the results shows that the hybrid algorithms converge to better solutions than their non-hybrid counterparts. The results further indicate that large sample sizes can be used to generate landscape analysis features, ensuring reliable approximations of the investigated functions' properties without lessening the meta-heuristics' performance.
更多查看译文
关键词
meta-heuristics,evolutionary algorithms,sampling algorithms,initialization,landscape analysis
AI 理解论文
溯源树
样例
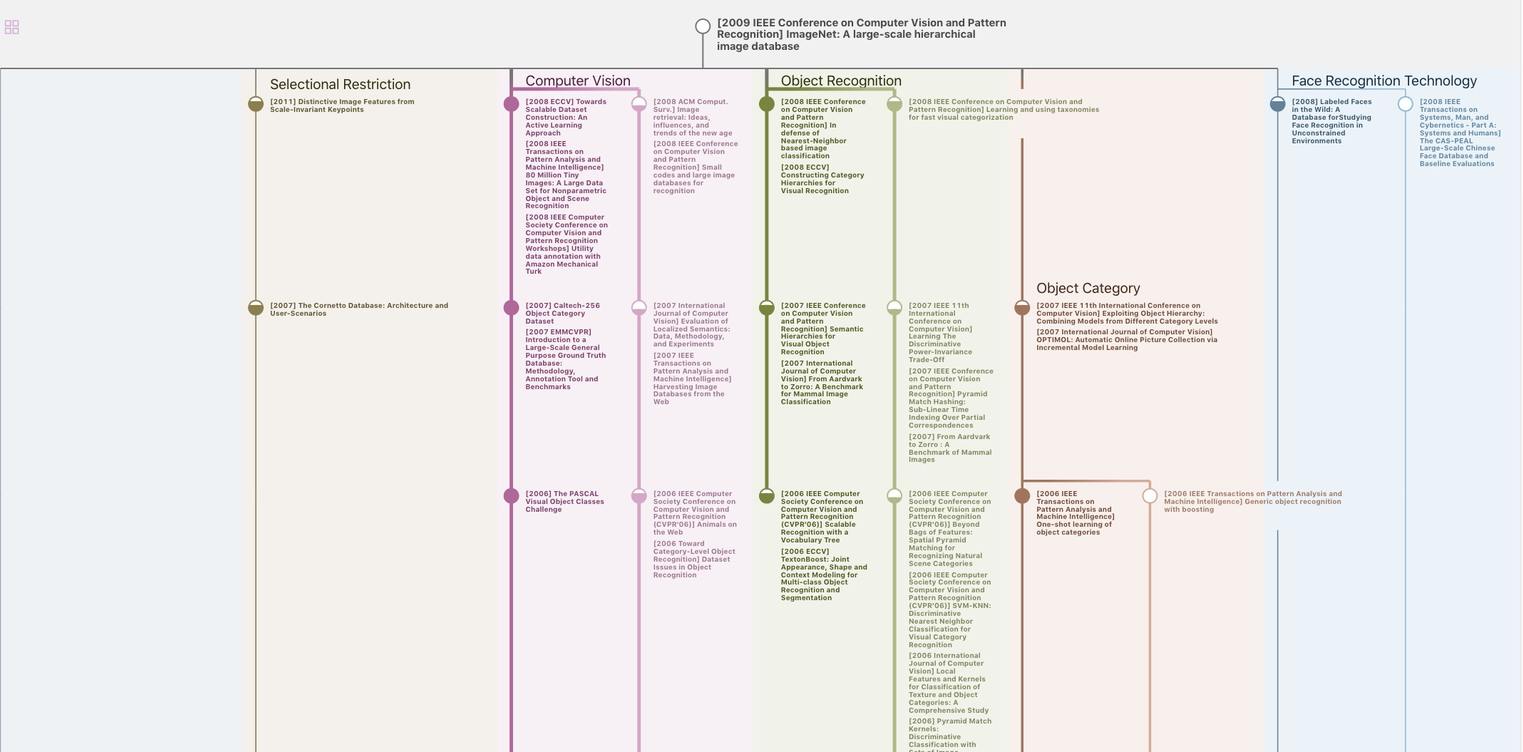
生成溯源树,研究论文发展脉络
Chat Paper
正在生成论文摘要