Sequential Hybridization of Online Surrogate Models for Continuous Optimization
PROCEEDINGS OF THE 2023 GENETIC AND EVOLUTIONARY COMPUTATION CONFERENCE COMPANION, GECCO 2023 COMPANION(2023)
摘要
Surrogate-based optimization is a common practice in different application fields in engineering and sciences, mainly when the objective function is computationally expensive. The selection of the best modeling approach to design the surrogate impacts howthe optimization will perform. This paper explores online surrogates trained during the optimization process and applied to filter nonpromising solutions before considering the evaluation with the actual fitness function. The study analyses two online alternative approaches proposed by the literature to implement surrogates. On the one hand, we consider traditional regression-based approaches that construct the surrogate as a continuous approximation of the fitness function; based on experiments on the same benchmark, this paper takes XGBoost regressor as the representative surrogate for this approach. On the other, we include a pairwise model that predicts whether a candidate solution is better than a reference one as a binary classifier; for this type of surrogate, we take a Decision Tree classifier, which performed the best in the same baseline study. The analysis of the two surrogates shows that the contribution of the surrogate varies during the different optimization phases. This paper demonstrates how combining complementary surrogates improves their behavior and the performance of the optimization as a whole.
更多查看译文
关键词
Evolutionary Algorithms,Machine Learning,Surrogate Models
AI 理解论文
溯源树
样例
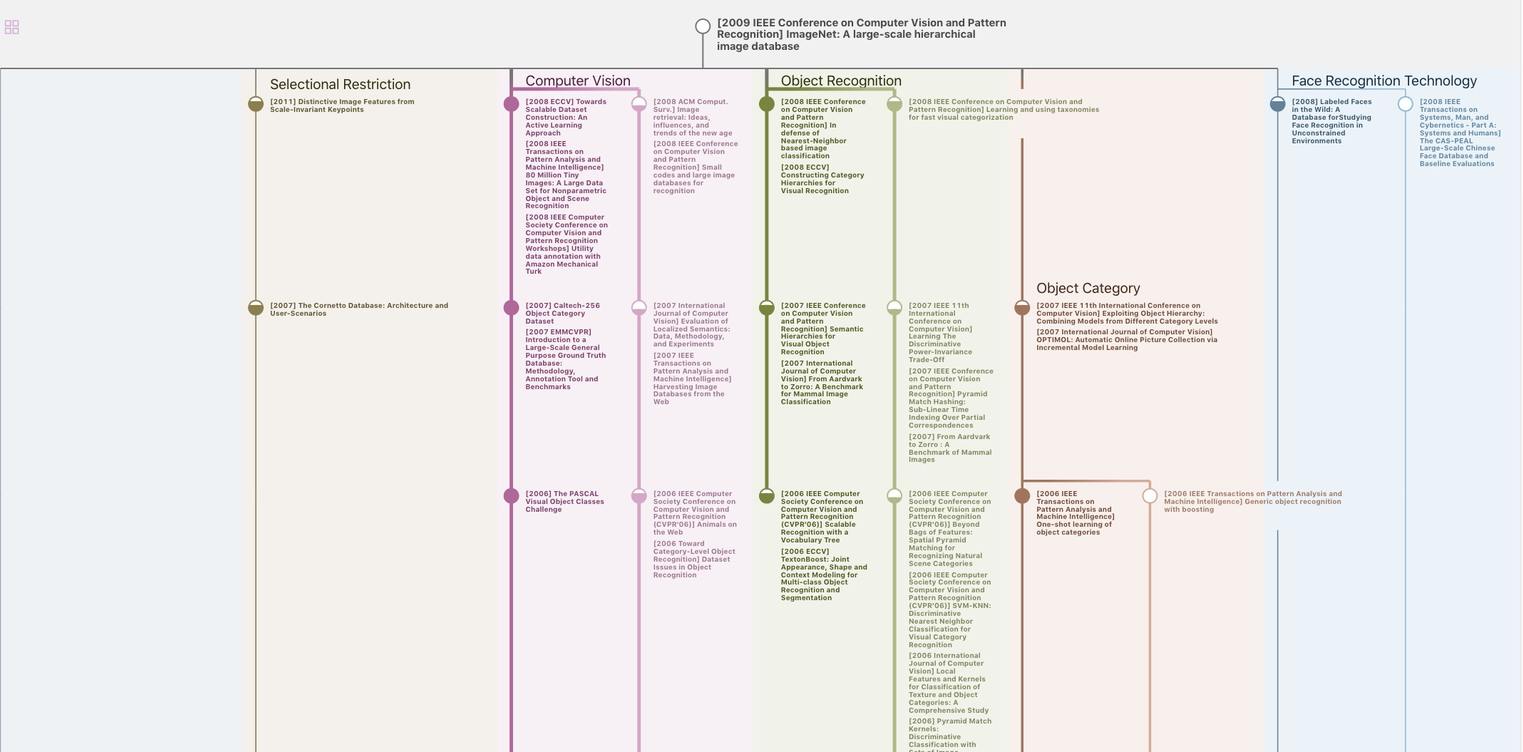
生成溯源树,研究论文发展脉络
Chat Paper
正在生成论文摘要