PrimeNet: Pre-training for Irregular Multivariate Time Series.
AAAI(2023)
摘要
Real-world applications often involve irregular time series, for which the time intervals between successive observations are non-uniform. Irregularity across multiple features in a multi-variate time series further results in a different subset of features at any given time (i.e., asynchronicity) . Existing pre-training schemes for time-series, however, often assume regularity of time series and make no special treatment of irregularity. We argue that such irregularity offers insight about domain property of the data—for example, frequency of hospital visits may signal patient health condition—that can guide representation learning. In this work, we propose PrimeNet to learn a self-supervised representation for irregular multivariate time-series. Specifically, we design a timesensitive contrastive learning and data reconstruction task to pre-train a model. Irregular time-series exhibits considerable variations in sampling density over time. Hence, our triplet generation strategy follows the density of the original data points, preserving its native irregularity. Moreover, the sampling density variation over time makes data reconstruction difficult for different regions. Therefore, we design a data masking technique that always masks a constant time duration to accommodate reconstruction for regions of different sampling density. We learn with these tasks using unlabeled data to build a pre-trained model and fine-tune on a downstream task with limited labeled data, in contrast with existing fully supervised approach for irregular time-series, requiring large amounts of labeled data. Experiment results show that PrimeNet significantly outperforms state-of-the-art methods on naturally irregular and asynchronous data from Healthcare and IoT applications for several downstream tasks, including classification, interpolation, and regression.
更多查看译文
关键词
series,pre-training
AI 理解论文
溯源树
样例
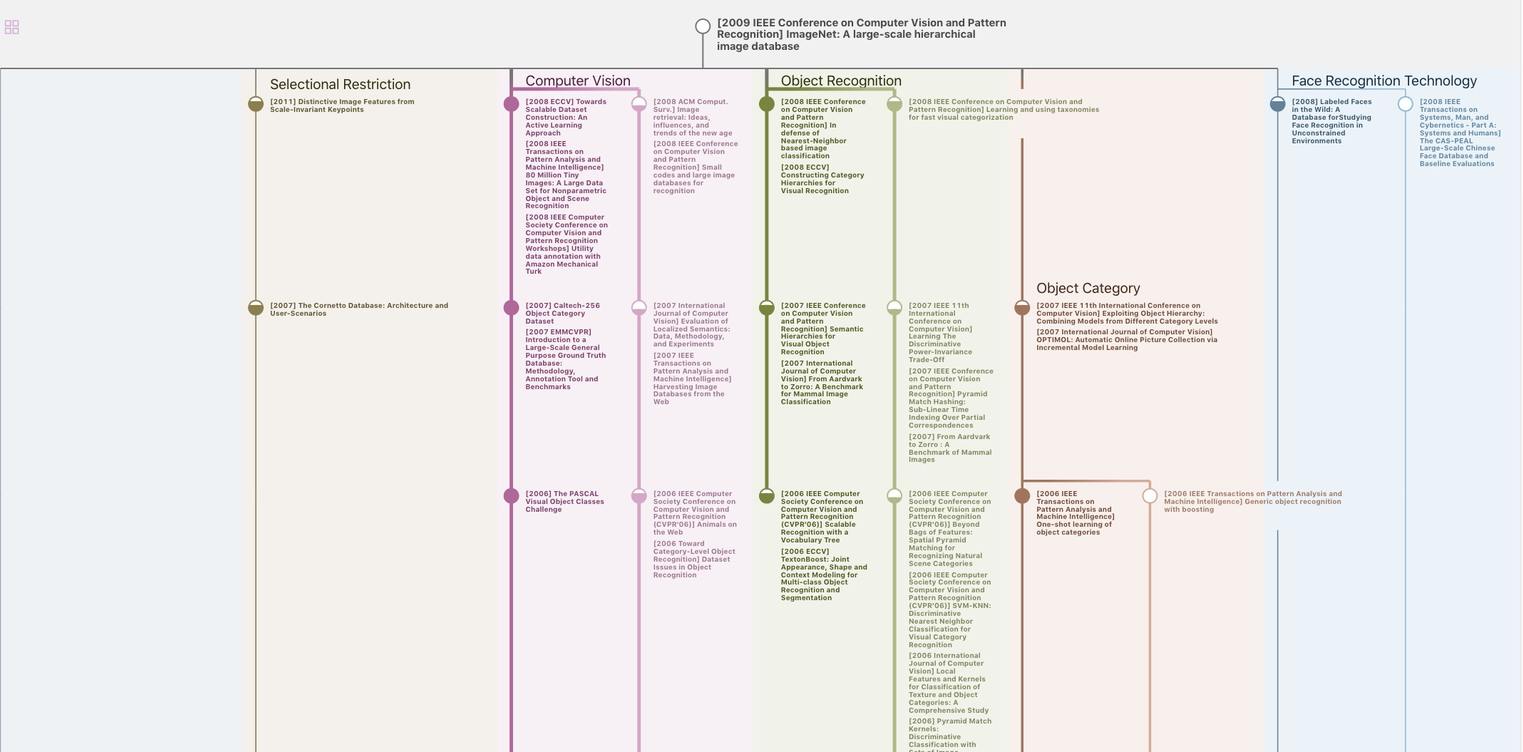
生成溯源树,研究论文发展脉络
Chat Paper
正在生成论文摘要