Jointly Imputing Multi-View Data with Optimal Transport.
AAAI(2023)
摘要
The multi-view data with incomplete information hinder the effective data analysis. Existing multi-view imputation methods that learn the mapping between complete view and completely missing view are not able to deal with the common multi-view data with missing feature information. In this paper, we propose a generative imputation model named Git with optimal transport theory to jointly impute the missing features/values, conditional on all observed values from the multi-view data. Git consists of two modules, i.e., a multiview joint generator (MJG) and a masking energy discriminator (MED). The generator MJG incorporates a joint autoencoder with the multiple imputation rule to learn the data distribution from all observed multi-view data. The discriminator MED leverages a new masking energy divergence function to make Git differentiable for imputation enhancement. Extensive experiments on several real-world multi-view data sets demonstrate that, Git yields over 35% accuracy gain, compared to the state-of-the-art approaches.
更多查看译文
关键词
optimal transport,data,multi-view
AI 理解论文
溯源树
样例
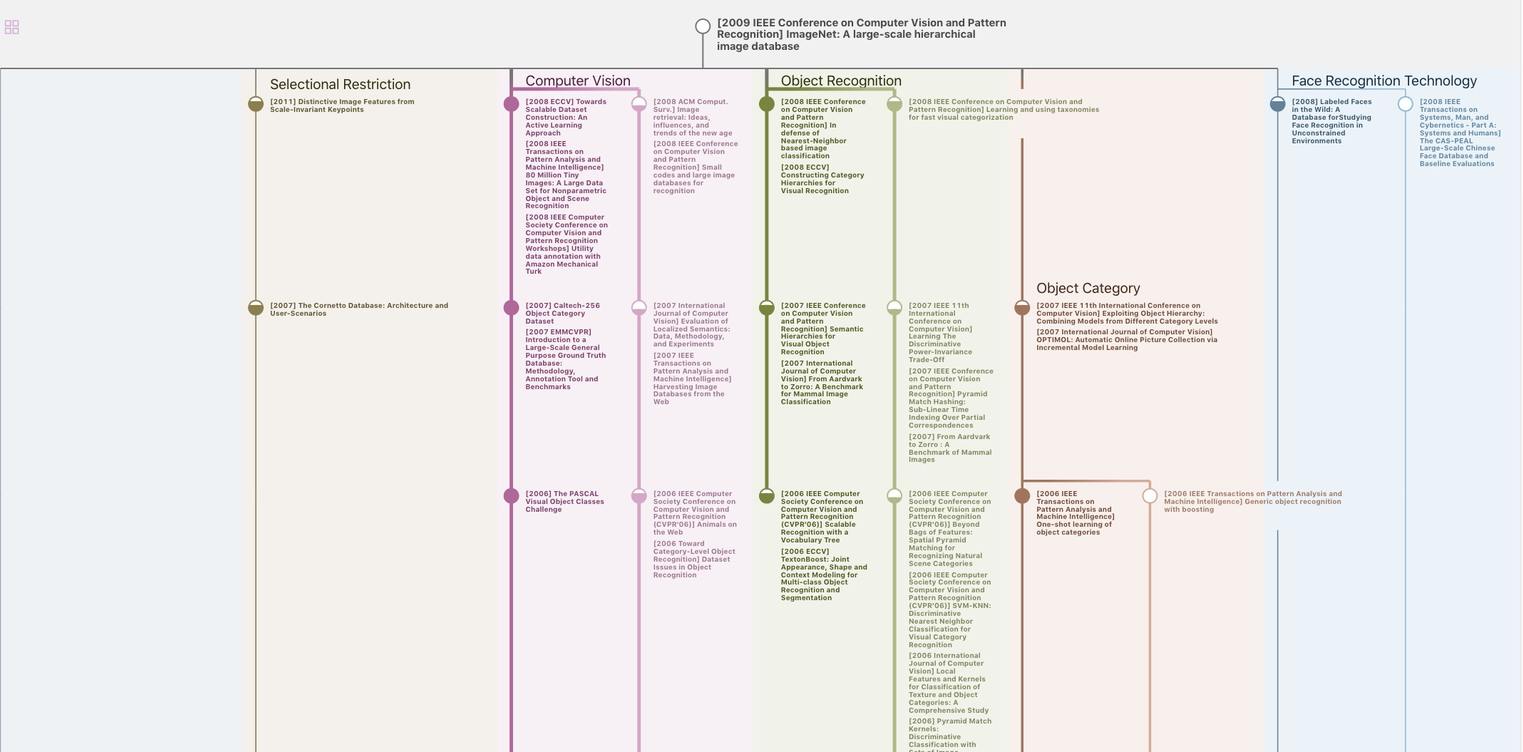
生成溯源树,研究论文发展脉络
Chat Paper
正在生成论文摘要