A Learnable Radial Basis Positional Embedding for Coordinate-MLPs.
AAAI(2023)
摘要
We propose a novel method to enhance the performance of coordinate-MLPs (also referred to as neural fields) by learning instance-specific positional embeddings. End-to-end optimization of positional embedding parameters along with network weights leads to poor generalization performance. Instead, we develop a generic framework to learn the positional embedding based on the classic graph-Laplacian regularization, which can implicitly balance the trade-off between memorization and generalization. This framework is then used to propose a novel positional embedding scheme, where the hyperparameters are learned per coordinate ( i.e instance) to deliver optimal performance. We show that the proposed embedding achieves better performance with higher stability compared to the well-established random Fourier features (RFF). Further, we demonstrate that the proposed embedding scheme yields stable gradients, enabling seamless integration into deep architectures as intermediate layers.
更多查看译文
关键词
learnable radial basis positional,coordinate-mlps
AI 理解论文
溯源树
样例
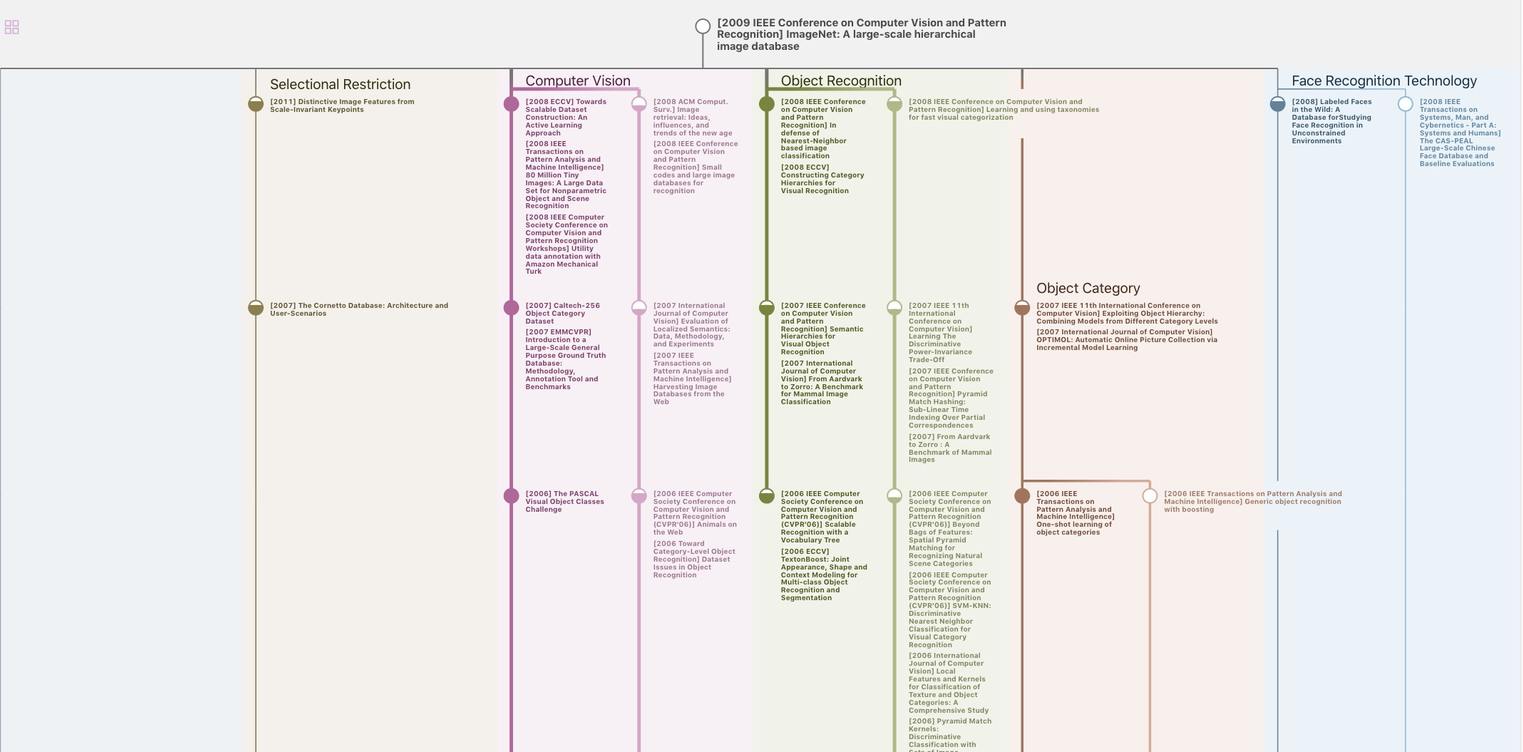
生成溯源树,研究论文发展脉络
Chat Paper
正在生成论文摘要