Fine-Grained Position Helps Memorizing More, a Novel Music Compound Transformer Model with Feature Interaction Fusion.
AAAI(2023)
摘要
Due to the particularity of the simultaneous occurrence of multiple events in music sequences, compound Transformer is proposed to deal with the challenge of long sequences. However, there are two deficiencies in the compound Transformer. First, since the order of events is more important for music than natural language, the information provided by the original absolute position embedding is not precise enough. Second, there is an important correlation between the tokens in the compound word, which is ignored by the current compound Transformer. Therefore, in this work, we propose an improved compound Transformer model for music understanding. Specifically, we propose an attribute embedding fusion module and a novel position encoding scheme with absolute-relative consideration. In the attribute embedding fusion module, different attributes are fused through feature permutation by using a multi-head self-attention mechanism in order to capture rich interactions between attributes. In the novel position encoding scheme, we propose RoAR position encoding, which realizes rotational absolute position encoding, relative position encoding, and absolute-relative position interactive encoding, providing clear and rich orders for musical events. Empirical study on four typical music understanding tasks shows that our attribute fusion approach and RoAR position encoding brings large performance gains. In addition, we further investigate the impact of masked language modeling and casual language modeling pre-training on music understanding.
更多查看译文
关键词
feature interaction fusion,music,fine-grained
AI 理解论文
溯源树
样例
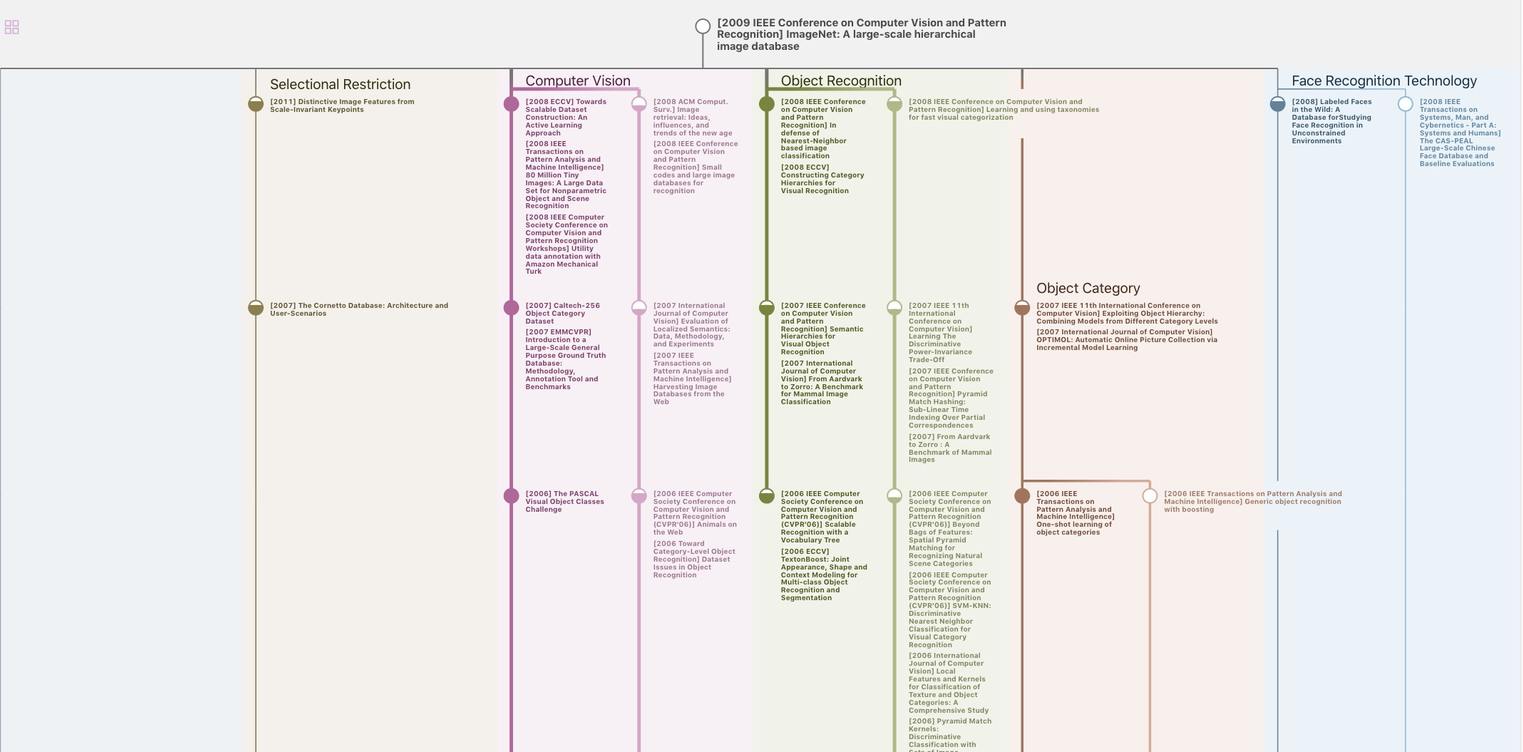
生成溯源树,研究论文发展脉络
Chat Paper
正在生成论文摘要