User-Controllable Arbitrary Style Transfer via Entropy Regularization.
AAAI(2023)
摘要
Ensuring the overall end-user experience is a challenging task in arbitrary style transfer (AST) due to the subjective nature of style transfer quality. A good practice is to provide users many instead of one AST result. However, existing approaches require to run multiple AST models or inference a diversified AST (DAST) solution multiple times, and thus they are either slow in speed or limited in diversity. In this paper, we propose a novel solution ensuring both efficiency and diversity for generating multiple user-controllable AST results by systematically modulating AST behavior at run-time. We begin with reformulating three prominent AST methods into a unified assign-and-mix problem and discover that the entropies of their assignment matrices exhibit a large variance. We then solve the unified problem in an optimal transport framework using the Sinkhorn-Knopp algorithm with a user input e to control the said entropy and thus modulate stylization. Empirical results demonstrate the superiority of the proposed solution, with speed and stylization quality comparable to or better than existing AST and significantly more diverse than previous DAST works.
更多查看译文
关键词
entropy,user-controllable
AI 理解论文
溯源树
样例
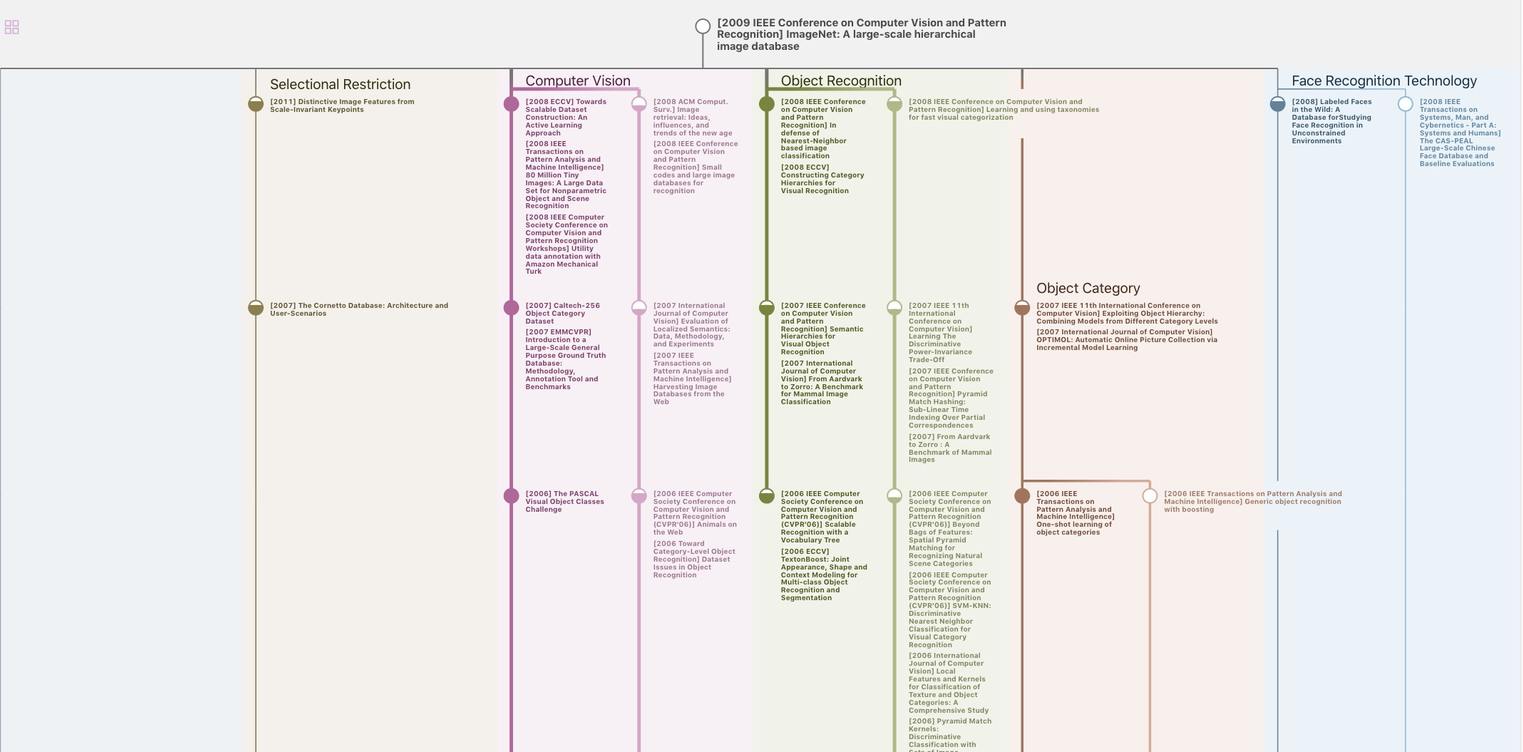
生成溯源树,研究论文发展脉络
Chat Paper
正在生成论文摘要