TAML: Time-Aware Meta Learning for Cold-Start Problem in News Recommendation
PROCEEDINGS OF THE 46TH INTERNATIONAL ACM SIGIR CONFERENCE ON RESEARCH AND DEVELOPMENT IN INFORMATION RETRIEVAL, SIGIR 2023(2023)
摘要
Meta-learning has become a widely used method for the user cold-start problem in recommendation systems, as it allows the model to learn from similar learning tasks and transfer the knowledge to new tasks. However, most existing meta-learning methods do not consider the temporal factor of users' preferences, which is crucial for news recommendation scenarios where news streams change dynamically over time. In this paper, we propose Time-Aware Meta-Learning (TAML), a novel framework that focuses on cold-start users in news recommendation systems. TAML factorizes user preferences into time-specific and time-shift representations that jointly affect users' news preferences. These temporal factors are further incorporated into the meta-learning framework to achieve accurate and timely cold-start recommendations. Extensive experiments are conducted on two real-world datasets, demonstrating the superior performance of TAML over state-of-the-art methods.
更多查看译文
关键词
News recommendation,cold-start problem,meta-learning,time-aware
AI 理解论文
溯源树
样例
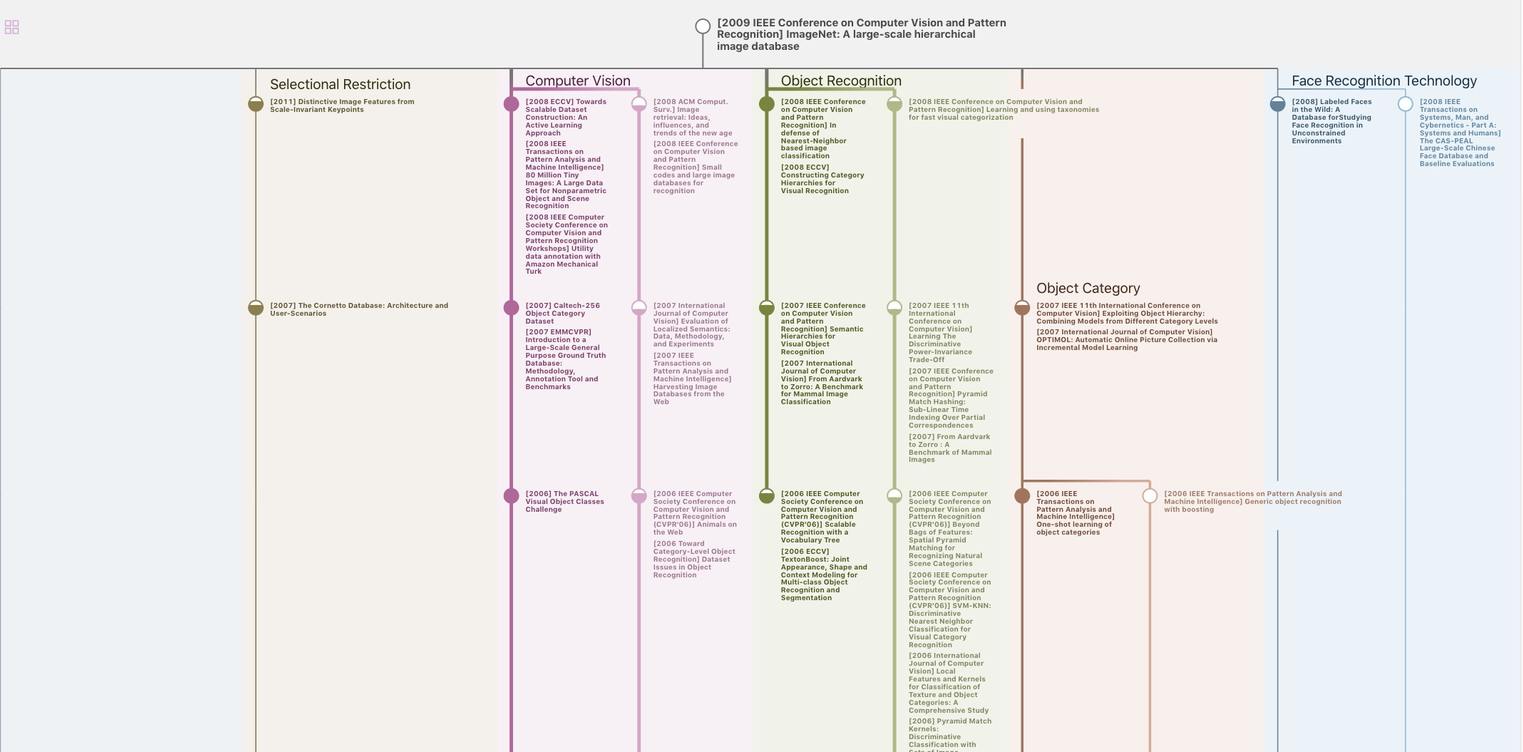
生成溯源树,研究论文发展脉络
Chat Paper
正在生成论文摘要