Limitations of Open-Domain Question Answering Benchmarks for Document-level Reasoning
PROCEEDINGS OF THE 46TH INTERNATIONAL ACM SIGIR CONFERENCE ON RESEARCH AND DEVELOPMENT IN INFORMATION RETRIEVAL, SIGIR 2023(2023)
摘要
Many recent QA models retrieve answers from passages, rather than whole documents, due to the limitations of deep learning models with limited context size. However, this approach ignores important document-level cues that can be crucial in answering questions. This paper reviews three open-domain QA benchmarks from a document-level perspective and finds that they are biased towards passage-level information. Out of 17,000 assessed questions, 82 were identified as requiring document-level reasoning and could not be answered by passage-based models. Document-level retrieval (BM25) outperformed both dense and sparse passage-level retrieval on these questions, highlighting the need for more evaluation of models' ability to understand documents, an often-overlooked challenge in open-domain QA.
更多查看译文
关键词
open-domain question answering,document-level reasoning,long context question answering
AI 理解论文
溯源树
样例
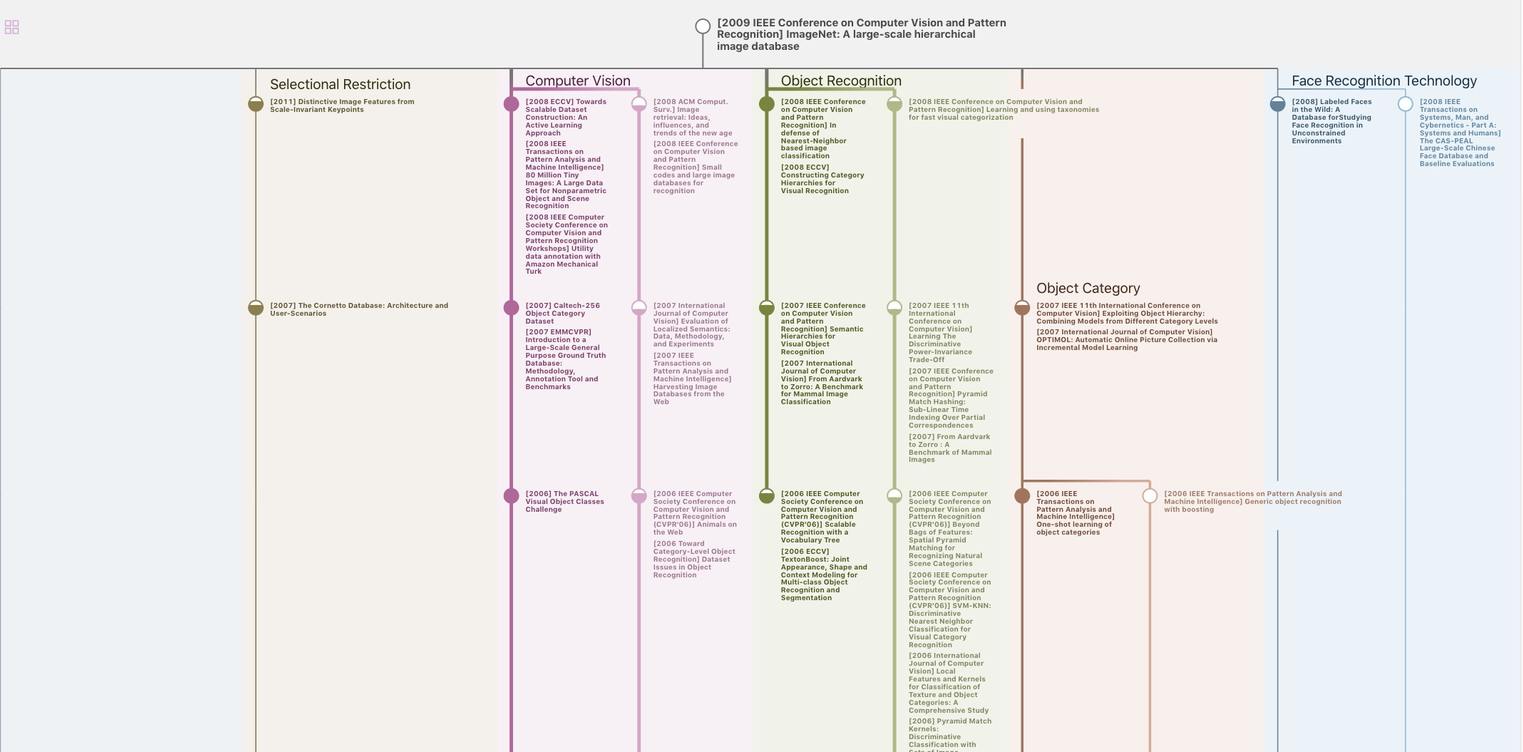
生成溯源树,研究论文发展脉络
Chat Paper
正在生成论文摘要