SimTDE: Simple Transformer Distillation for Sentence Embeddings
PROCEEDINGS OF THE 46TH INTERNATIONAL ACM SIGIR CONFERENCE ON RESEARCH AND DEVELOPMENT IN INFORMATION RETRIEVAL, SIGIR 2023(2023)
摘要
In this paper we introduce SimTDE, a simple knowledge distillation framework to compress sentence embeddings transformer models with minimal performance loss and significant size and latency reduction. SimTDE effectively distills large and small transformers via a compact token embedding block and a shallow encoding block, connected with a projection layer, relaxing dimension match requirement. SimTDE simplifies distillation loss to focus only on token embedding and sentence embedding. We evaluate on standard semantic textual similarity (STS) tasks and entity resolution (ER) tasks. It achieves 99.94% of the state-of-the-art (SOTA) SimCSE-Bert-Base performance with 3 times size reduction and 96.99% SOTA performance with 12 times size reduction on STS tasks. It also achieves 99.57% of teacher's performance on multi-lingual ER data with a tiny transformer student model of 1.4M parameters and 5.7MB size. Moreover, compared to other distilled transformers SimTDE is 2 times faster at inference given similar size and still 1.17 times faster than a model 33% smaller (e.g. MiniLM). The easy-to-adopt framework, strong accuracy and low latency of SimTDE can widely enable runtime deployment of SOTA sentence embeddings.
更多查看译文
关键词
Knowledge Distillation,Sentence Embeddings,Semantic Text Similarity,Entity Resolution
AI 理解论文
溯源树
样例
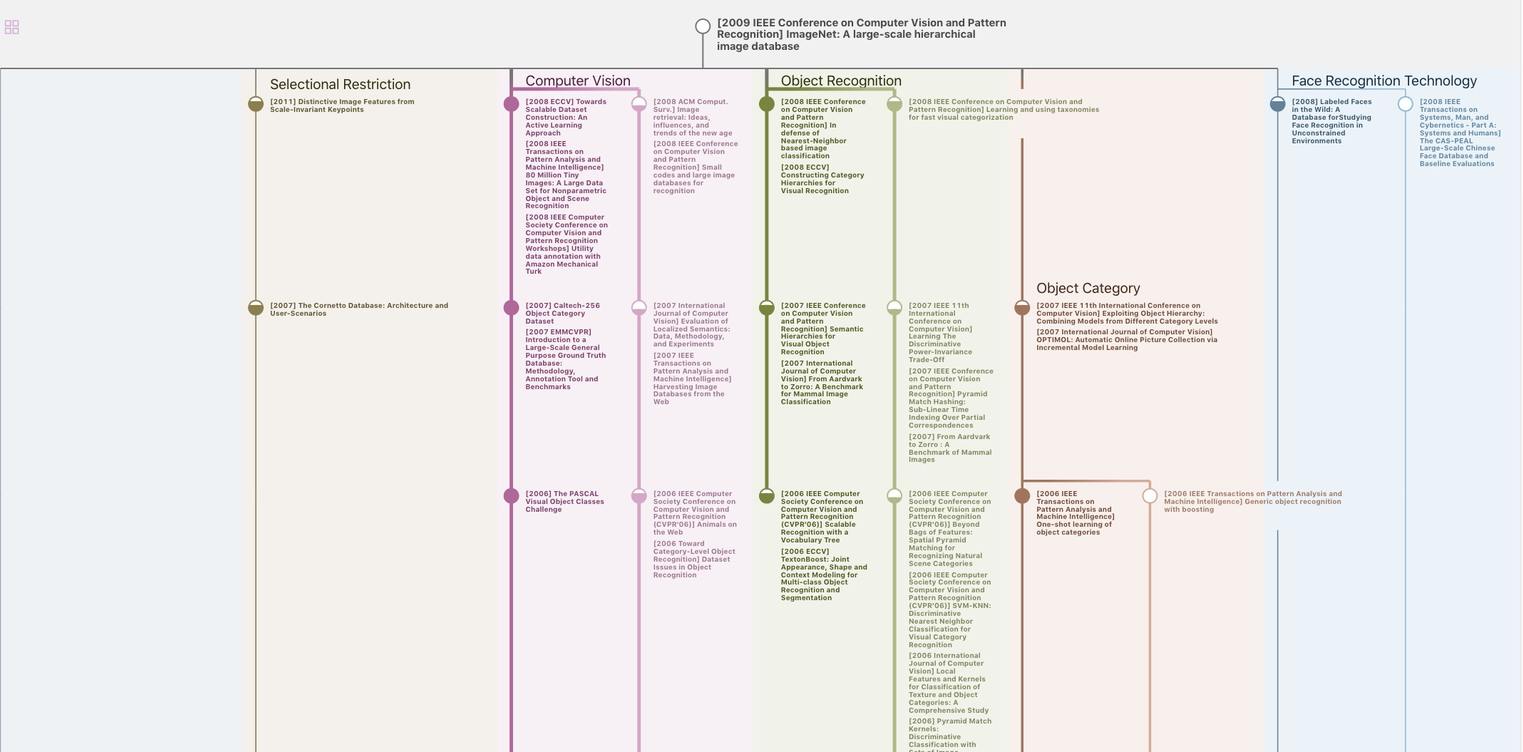
生成溯源树,研究论文发展脉络
Chat Paper
正在生成论文摘要