Multimodal Counterfactual Learning Network for Multimedia-based Recommendation
PROCEEDINGS OF THE 46TH INTERNATIONAL ACM SIGIR CONFERENCE ON RESEARCH AND DEVELOPMENT IN INFORMATION RETRIEVAL, SIGIR 2023(2023)
摘要
Multimedia-based recommendation (MMRec) utilizes multimodal content (images, textual descriptions, etc.) as auxiliary information on historical interactions to determine user preferences. Most MM-Rec approaches predict user interests by exploiting a large amount of multimodal contents of user-interacted items, ignoring the potential effect of multimodal content of user-uninteracted items. As a matter of fact, there is a small portion of user preference-irrelevant features in the multimodal content of user-interacted items, which may be a kind of spurious correlation with user preferences, thereby degrading the recommendation performance. In this work, we argue that the multimodal content of user-uninteracted items can be further exploited to identify and eliminate the user preferenceirrelevant portion inside user-interacted multimodal content, for example by counterfactual inference of causal theory. Going beyond multimodal user preference modeling only using interacted items, we propose a novel model called Multimodal Counterfactual Learning Network (MCLN), in which user-uninteracted items' multimodal content is additionally exploited to further purify the representation of user preference-relevant multimodal content that better matches the user's interests, yielding state-of-the-art performance. Extensive experiments are conducted to validate the effectiveness and rationality of MCLN. We release the complete codes of MCLN at https://github.com/hfutmars/MCLN.
更多查看译文
关键词
Recommender Systems,Multimodal User Preference,Counterfactual Learning,Spurious Correlation
AI 理解论文
溯源树
样例
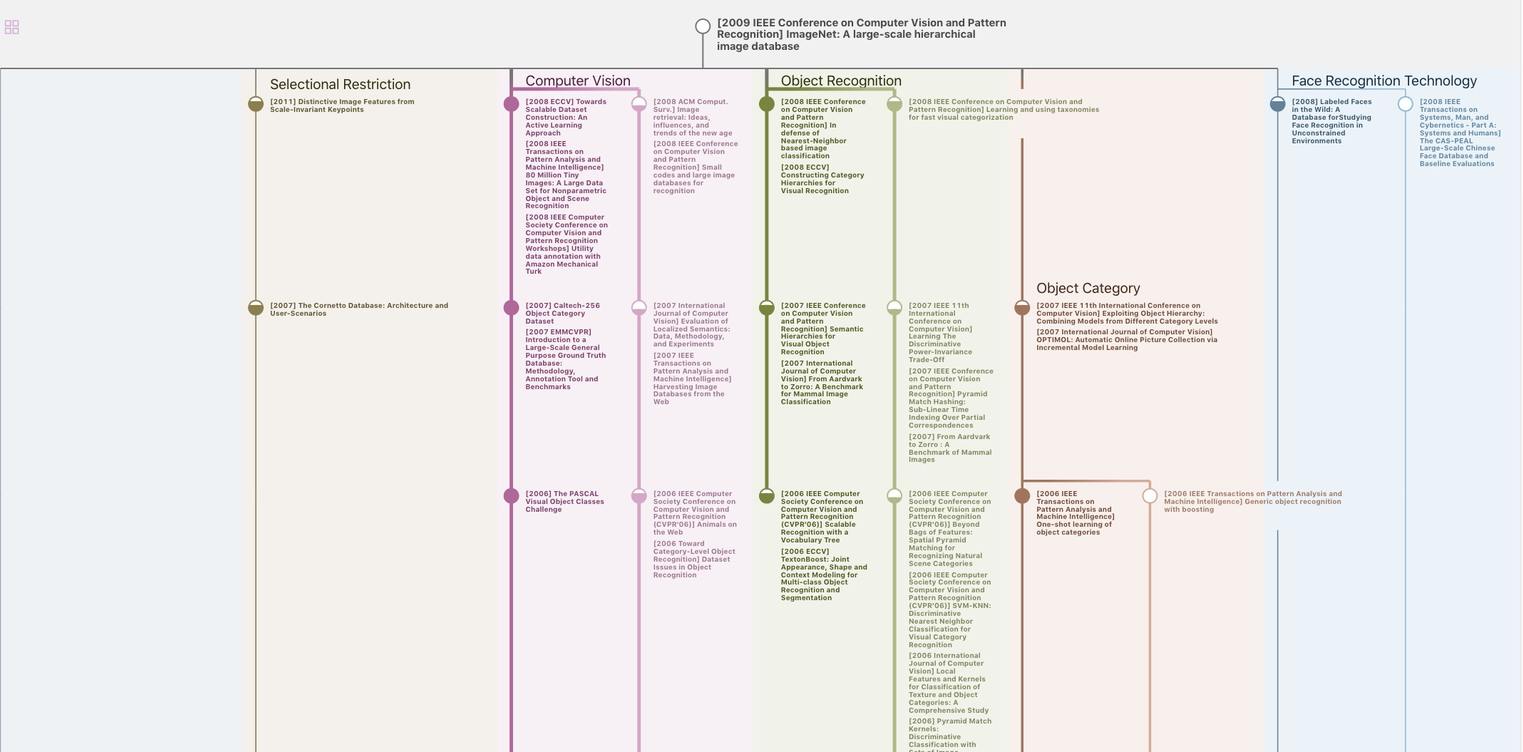
生成溯源树,研究论文发展脉络
Chat Paper
正在生成论文摘要