TMML: Text-Guided MuliModal Product Location For Alleviating Retrieval Inconsistency in E-Commerce
PROCEEDINGS OF THE 46TH INTERNATIONAL ACM SIGIR CONFERENCE ON RESEARCH AND DEVELOPMENT IN INFORMATION RETRIEVAL, SIGIR 2023(2023)
摘要
Image retrieval system (IRS) is commonly used in E-Commerce platforms for a wide range of applications such as price comparison and commodity recommendation. However, customers may experience inconsistent retrieval problems. Although the retrieved image contains the query object, the main product of the retrieved image is not associated with the query product. This is caused by the wrong product instance location when building the product image retrieval library. We can easily determine which product is on sale through the hint of the title, so we propose Text-Guided MuliModal Product Location (TMML) to use additional product titles to assist in locating the actual selling product instance. We design a weakly-aligned region-text data collection method to generate region-text pseudo-label by utilizing the IRS and user behavior from the E-commerce platform. To mitigate the impact of data noise, we propose a Mutual-Aware Contrastive Loss. Our results show that the proposed TMML outperforms the state-of-the-art method GLIP [11] by 3.95% in top-1 precision on our multi-objects test set, and 2.53% error located images in AliExpress has been corrected, which greatly alleviates the retrieval inconsistencies in IRS.
更多查看译文
关键词
Image Retrieval,Product Location,MultiModal Learning
AI 理解论文
溯源树
样例
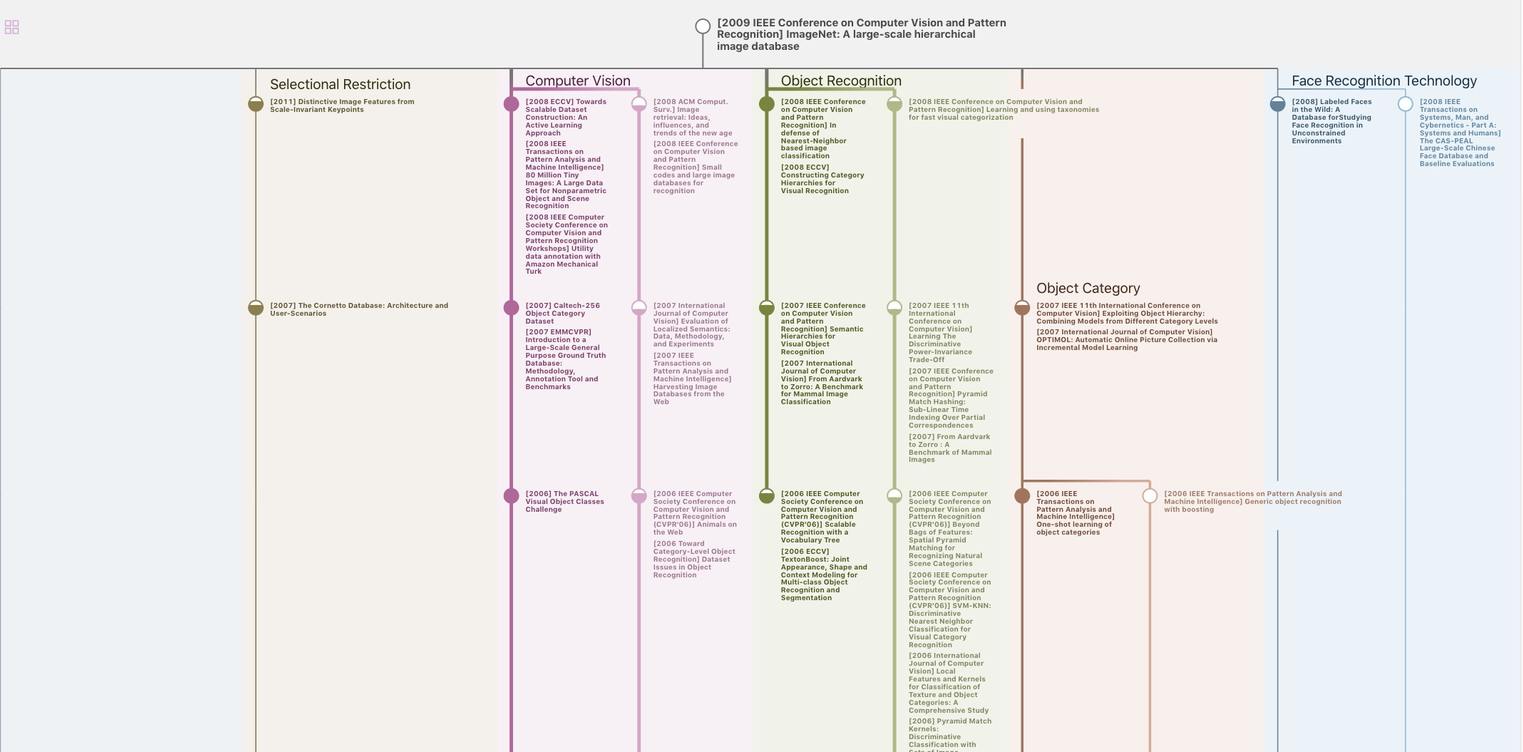
生成溯源树,研究论文发展脉络
Chat Paper
正在生成论文摘要