BLADE: Combining Vocabulary Pruning and Intermediate Pretraining for Scaleable Neural CLIR
PROCEEDINGS OF THE 46TH INTERNATIONAL ACM SIGIR CONFERENCE ON RESEARCH AND DEVELOPMENT IN INFORMATION RETRIEVAL, SIGIR 2023(2023)
摘要
Learning sparse representations using pretrained language models enhances the monolingual ranking effectiveness. Such representations are sparse vectors in the vocabulary of a language model projected from document terms. Extending such approaches to Cross-Language Information Retrieval (CLIR) using multilingual pretrained language models poses two challenges. First, the larger vocabularies of multilingual models affect both training and inference efficiency. Second, the representations of terms from different languages with similar meanings might not be sufficiently similar. To address these issues, we propose a learned sparse representation model, BLADE, combining vocabulary pruning with intermediate pre-training based on cross-language supervision. Our experiments reveal BLADE significantly reduces indexing time compared to its monolingual counterpart, SPLADE, on machine-translated documents, and it generates rankings with strengths complementary to those of other efficient CLIR methods.
更多查看译文
关键词
Sparse representation learning,neural CLIR,multilingual LM
AI 理解论文
溯源树
样例
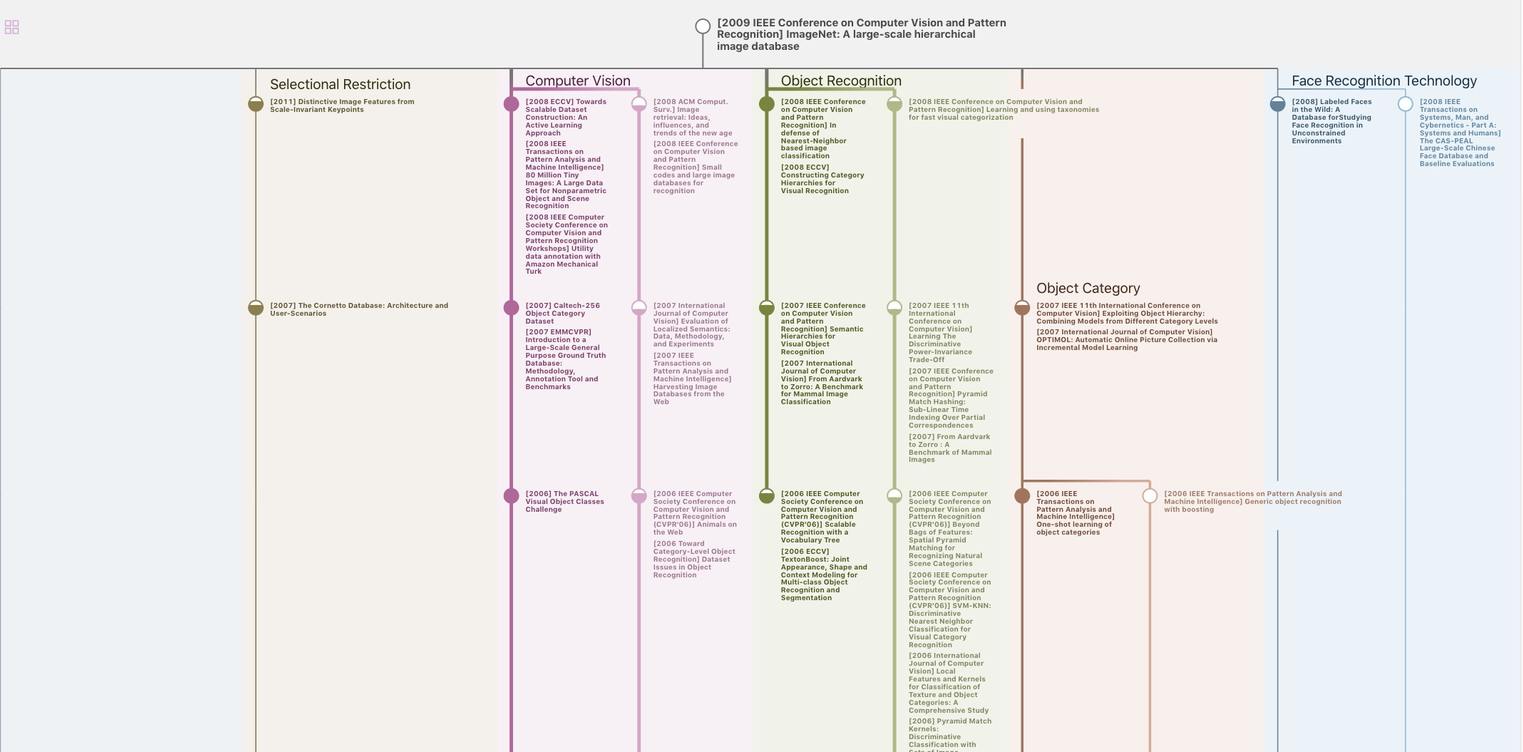
生成溯源树,研究论文发展脉络
Chat Paper
正在生成论文摘要