SparseEmbed: Learning Sparse Lexical Representations with Contextual Embeddings for Retrieval
PROCEEDINGS OF THE 46TH INTERNATIONAL ACM SIGIR CONFERENCE ON RESEARCH AND DEVELOPMENT IN INFORMATION RETRIEVAL, SIGIR 2023(2023)
摘要
In dense retrieval, prior work has largely improved retrieval effectiveness using multi-vector dense representations, exemplified by ColBERT. In sparse retrieval, more recent work, such as SPLADE, demonstrated that one can also learn sparse lexical representations to achieve comparable effectiveness while enjoying better interpretability. In this work, we combine the strengths of both the sparse and dense representations for first-stage retrieval. Specifically, we propose SparseEmbed - a novel retrieval model that learns sparse lexical representations with contextual embeddings. Compared with SPLADE, our model leverages the contextual embeddings to improve model expressiveness. Compared with ColBERT, our sparse representations are trained end-to-end to optimize both efficiency and effectiveness.
更多查看译文
关键词
Sparse Retrieval,Dense Retrieval,Contextual Embeddings
AI 理解论文
溯源树
样例
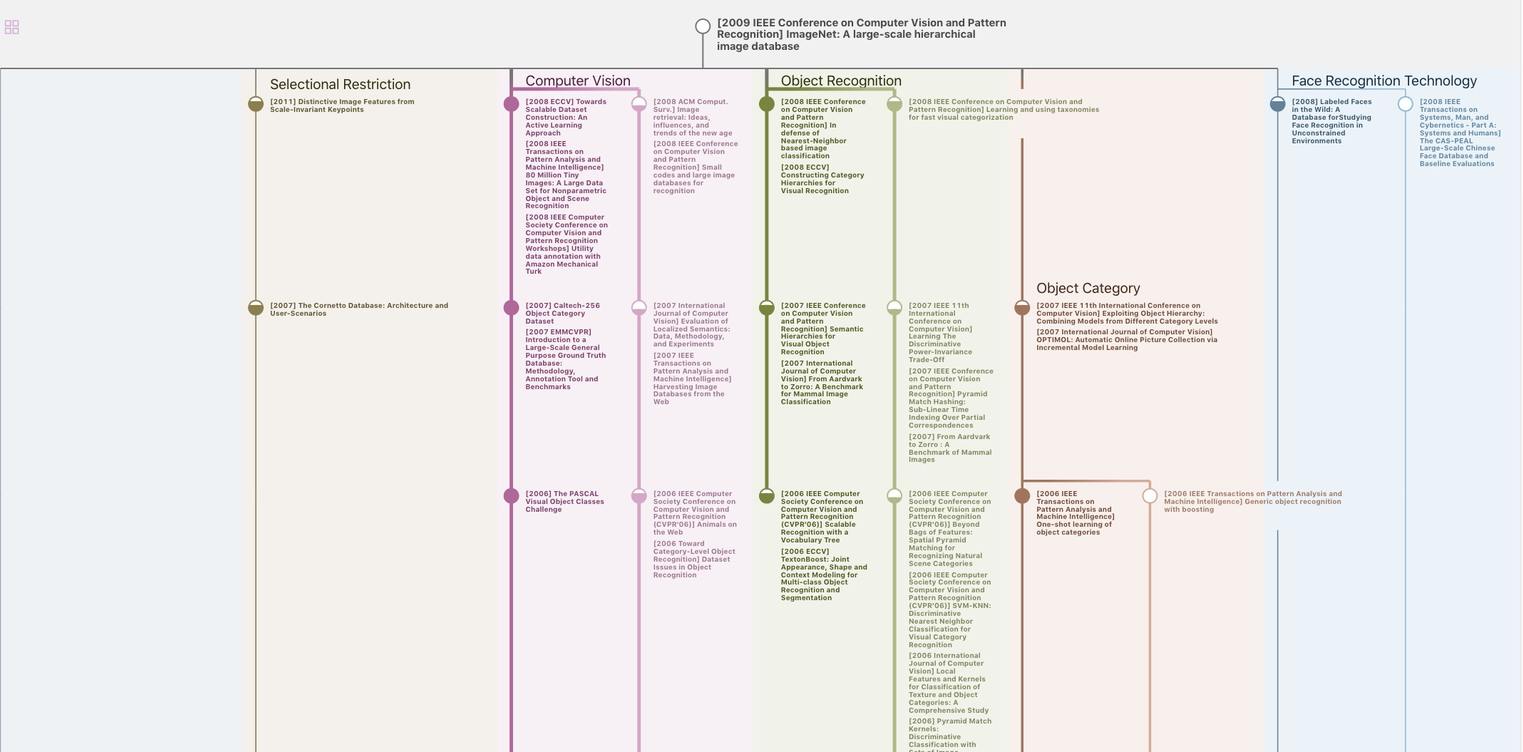
生成溯源树,研究论文发展脉络
Chat Paper
正在生成论文摘要