Quantifying and Leveraging User Fatigue for Interventions in Recommender Systems
PROCEEDINGS OF THE 46TH INTERNATIONAL ACM SIGIR CONFERENCE ON RESEARCH AND DEVELOPMENT IN INFORMATION RETRIEVAL, SIGIR 2023(2023)
摘要
Predicting churn and designing intervention strategies are crucial for online platforms to maintain user engagement. We hypothesize that predicting churn, i.e. users leaving from the system without further return, is often a delayed act, and it might get too late for the system to intervene. We propose detecting early signs of users losing interest, allowing time for intervention, and introduce a new formulation of user fatigue as short-term dissatisfaction, providing early signals to predict long-term churn. We identify behavioral signals predicting fatigue and develop models for fatigue prediction. Furthermore, we leverage the predicted fatigue estimates to develop fatigue-aware ad-load balancing intervention strategy that reduces churn, improving short- and long-term user retention. Results from deployed recommendation system and multiple live A/B tests across over 80 million users generating over 200 million sessions highlight gains for user engagement and platform strategic metrics.
更多查看译文
关键词
user churn,user fatigue,recommendation system,social media
AI 理解论文
溯源树
样例
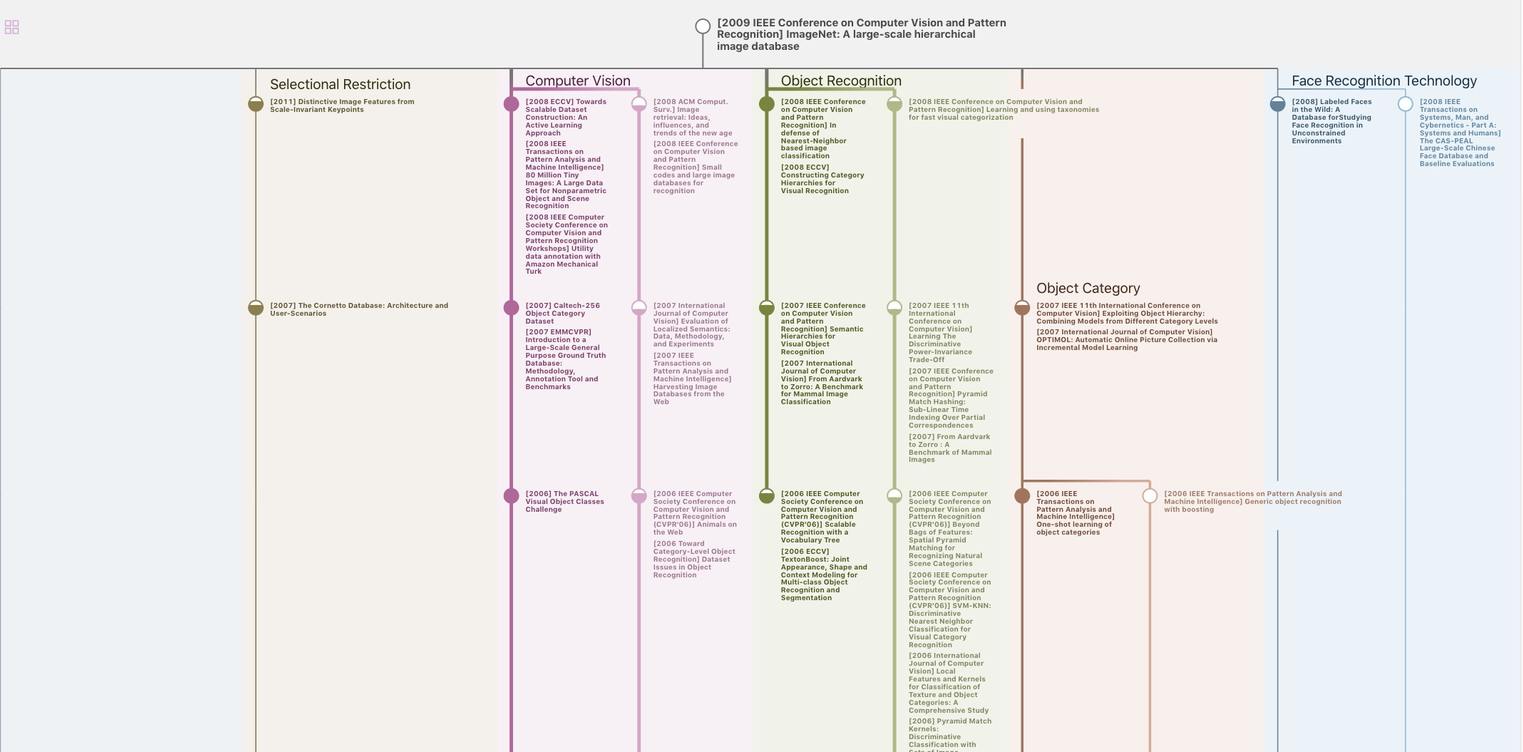
生成溯源树,研究论文发展脉络
Chat Paper
正在生成论文摘要