Deep Reinforcement Learning Based Task-Oriented Communication in Multi-Agent Systems
IEEE Wirel. Commun.(2023)
摘要
Driven by the increasing demand for executing intelligent tasks in various fields, multi-agent system (MAS) has drawn significant attention recently. An MAS relies on efficient communication between agents to exchange task-relevant information, so as support cooperative operation. Meanwhile, traditional communication systems are bit-oriented, which neglect the content and task relevance of the transmitted data. Thus, if bit-oriented communication patterns are applied in a MAS, a significant amount of task-irrelevant data would be transmitted, leading to communication resource waste and low operational efficiency. Considering that many emerging MASs are data-intensive and delay-sensitive, traditional ways of communication are unfit for these MASs. Task-oriented communication is a promising solution to deal with this issue, but its application in MAS still faces various challenges. In this article, we propose a task-oriented communication based framework for MAS, aiming to support efficient cooperation among agents. This framework specifies the collection, transmission, and processing of task-relevant information, in which task relevance is fully utilized to enhance communication efficiency. Based on the proposed framework, we then apply deep reinforcement learning (DRL) to implement task-oriented communication, in which a modular design and an end-to-end design for information extraction, data transmission, and task execution are proposed. Finally, the open problems for future research are discussed.
更多查看译文
关键词
Deep learning, Reinforcement learning, Cooperative systems, Information retrieval, Data communication, Task analysis, Multi-agent systems
AI 理解论文
溯源树
样例
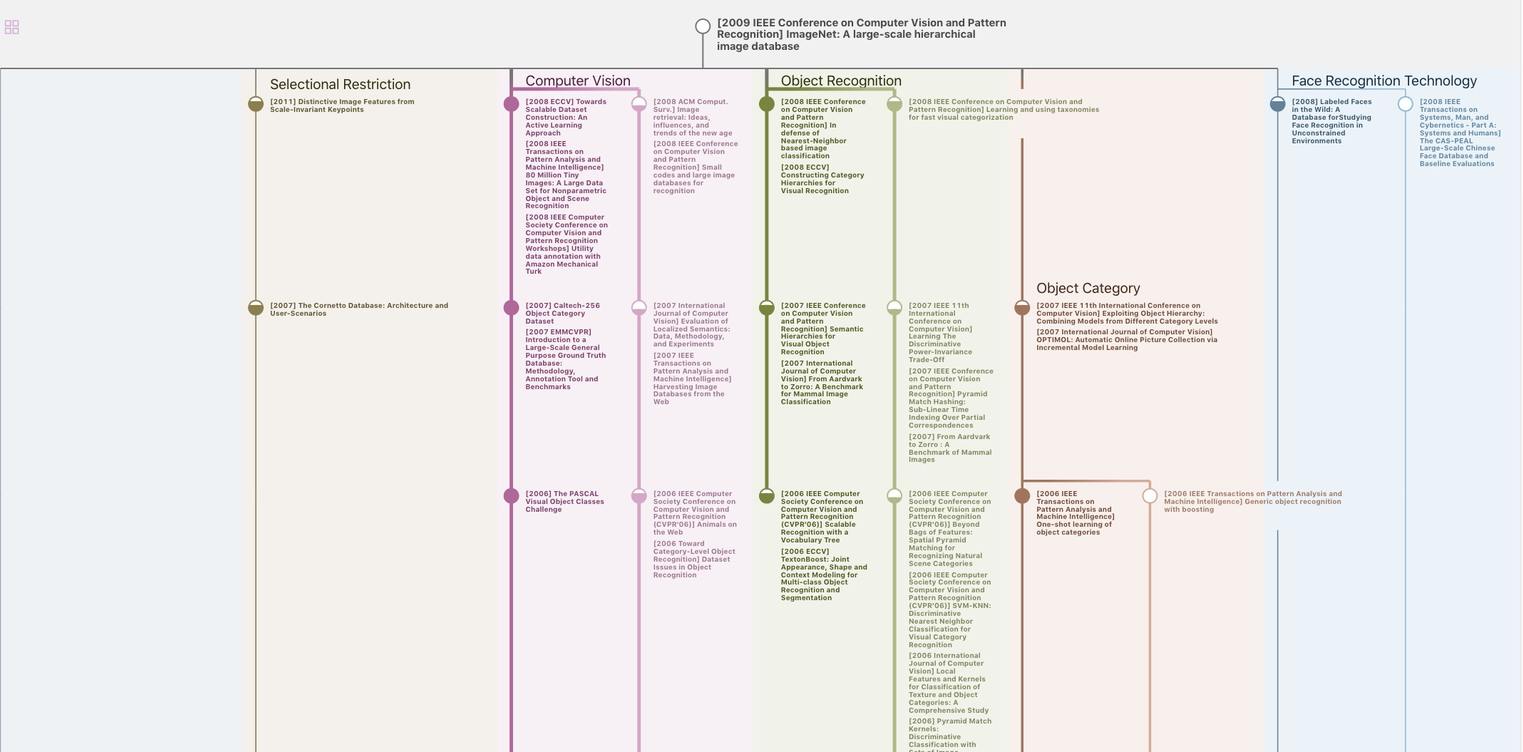
生成溯源树,研究论文发展脉络
Chat Paper
正在生成论文摘要