Spatial Downscaling of Lunar Surface Temperature Based on Geographically Weighted Regression.
IEEE Geosci. Remote. Sens. Lett.(2023)
摘要
Lunar exploration has put further demands on the high spatial resolution of the lunar surface temperature (LuST) data. However, available LuST data with coarse resolution provided by current remote sensing platforms (e.g., Lunar Reconnaissance Orbiter (LRO) Diviner) are unable to meet the requirement. Therefore, it is necessary to develop new methods to obtain LuST data with high spatial resolution. Inspired by the study of land surface temperature (LST) downscaling, here, we perform the exploratory study of the LuST downscaling. The core of the exploration work consists of two parts: 1) the 16 pixels per degree (ppd) Diviner gridded data records (GDRs) images are downscaled to 128 ppd using a downscaling model based on geographically weighted regression (GWR), in order to explore the feasibility of downscaling LuST and 2) two sets of experiments with scales of 4 and 8 are conducted and the growth characteristics of the errors are observed to determine whether it is feasible to obtain higher-spatial-resolution data. The evaluation metrics for the experiments in two specific craters with scale of 8 are 4.03/5.42 K for mean absolute error (MAE), 5.89/8.05 K for root mean square error (RMSE), and 0.037/0.048 for normalized RMSE (NRMSE), respectively. The LuST downscaling study is feasible, and it provides an effective way for obtaining high-spatial-resolution LuST data, which has positive implications for lunar exploration.
更多查看译文
关键词
lunar surface temperature,geographically weighted regression
AI 理解论文
溯源树
样例
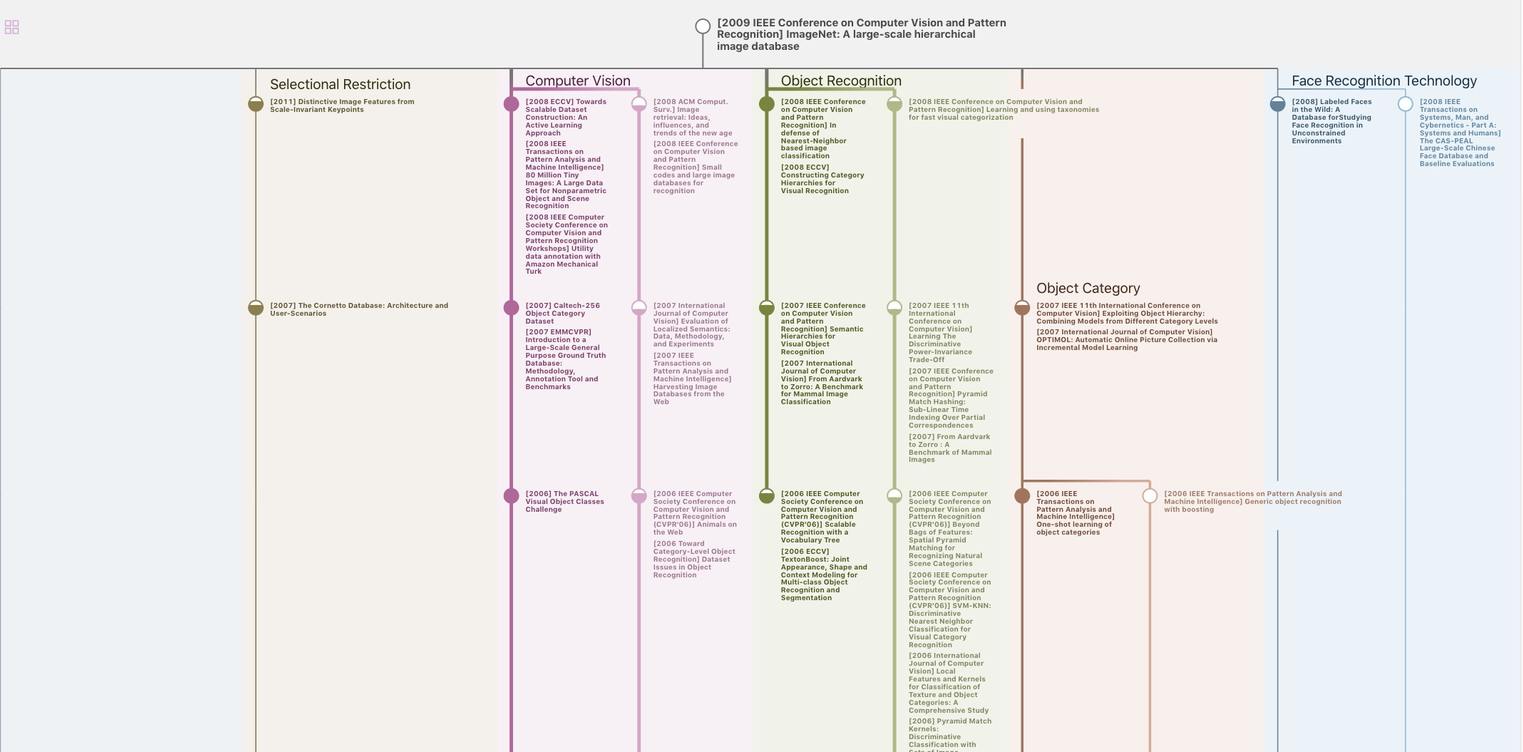
生成溯源树,研究论文发展脉络
Chat Paper
正在生成论文摘要