CLSR: Contrastive Learning for Semi-Supervised Remote Sensing Image Super-Resolution.
IEEE Geosci. Remote. Sens. Lett.(2023)
摘要
Real-world degradations diverge from ideal degradations, since most self-supervised and unsupervised learning scenarios generate low-resolution (LR) fake counterpart images from existing data using a common bicubic kernel. Additionally, conventional unsupervised learning techniques rely on a large number of training samples with excessive diversity as an inevitable requirement to reconstruct missing data based on their downsampled correlation. Practically, it is time-consuming to arrange large counts of samples along with the diversity for training. In this letter, we proposed a network CLSR: contrastive learning for remote sensing image super-resolution (SR) in a semi-supervised setting. Contrastive learning is the idea of comparing two samples to find shared features and attributes that set one data class apart, thus boosting visual task performance. Experiments demonstrate that it can super-resolve different modalities of data: single-band, multispectral band, RGB remote sensing images, and real-world natural images.
更多查看译文
关键词
Contrastive learning, remote-sensing image super-resolution (SR), semi-supervised image SR, unsupervised image SR
AI 理解论文
溯源树
样例
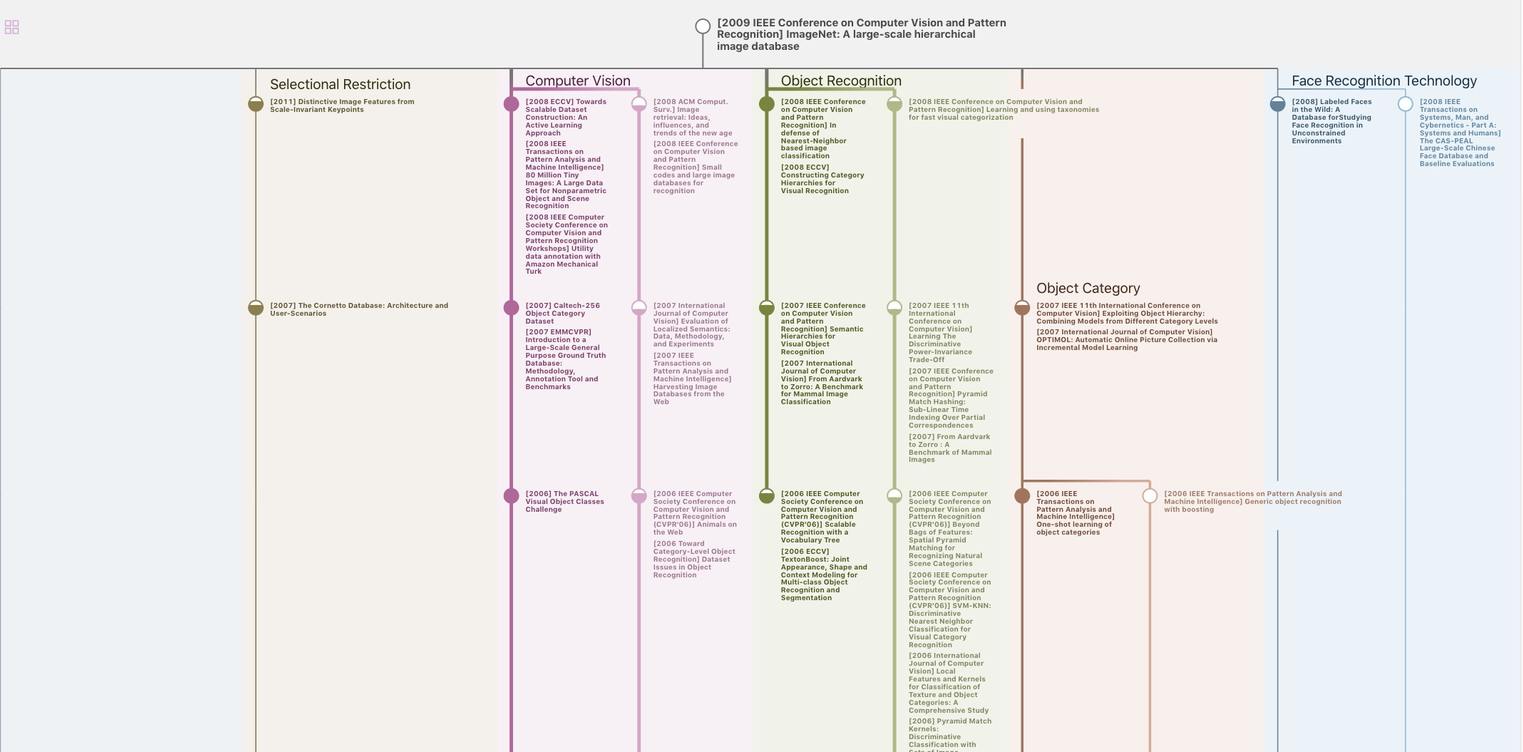
生成溯源树,研究论文发展脉络
Chat Paper
正在生成论文摘要