Multilevel Context Feature Fusion for Semantic Segmentation of ALS Point Cloud.
IEEE Geosci. Remote. Sens. Lett.(2023)
摘要
Semantic segmentation of airborne laser scanning (ALS) point clouds using deep learning is a hot research in remote sensing and photogrammetry. A current trend is to aggregate contextual features from different scales for boosting network generalization and diversity discrimination capabilities. One main challenge is how to achieve effective fusion with multi-scale information. In this letter, we propose a multilevel context feature fusion network (MCFN) for semantic segmentation of ALS point cloud based on an encoder-decoder structure. More specifically, we design the squeeze-expansion shared multilayer perceptron (SE-MLP) module following kernel point convolution (KPConv) in the encoding stage, which can extend the receptive field of KPConv. To aggregate low-level features and highlevel representations, we establish channel self-attention between skip connections. In the decoding stage, we develop a crosslayer attention fusion (CAF) module to generate additional discriminative channel features by fusing multiscale features at different upsampling layers. Experiments on the ISPRS and LASDU datasets demonstrate the superiority of the proposed method. Code: https://github.com/SC-shendazt/MCFN.
更多查看译文
关键词
Attention mechanism, encoder-decoder structure, kernel point convolution (KPConv), multilevel fusion, point cloud semantic segmentation
AI 理解论文
溯源树
样例
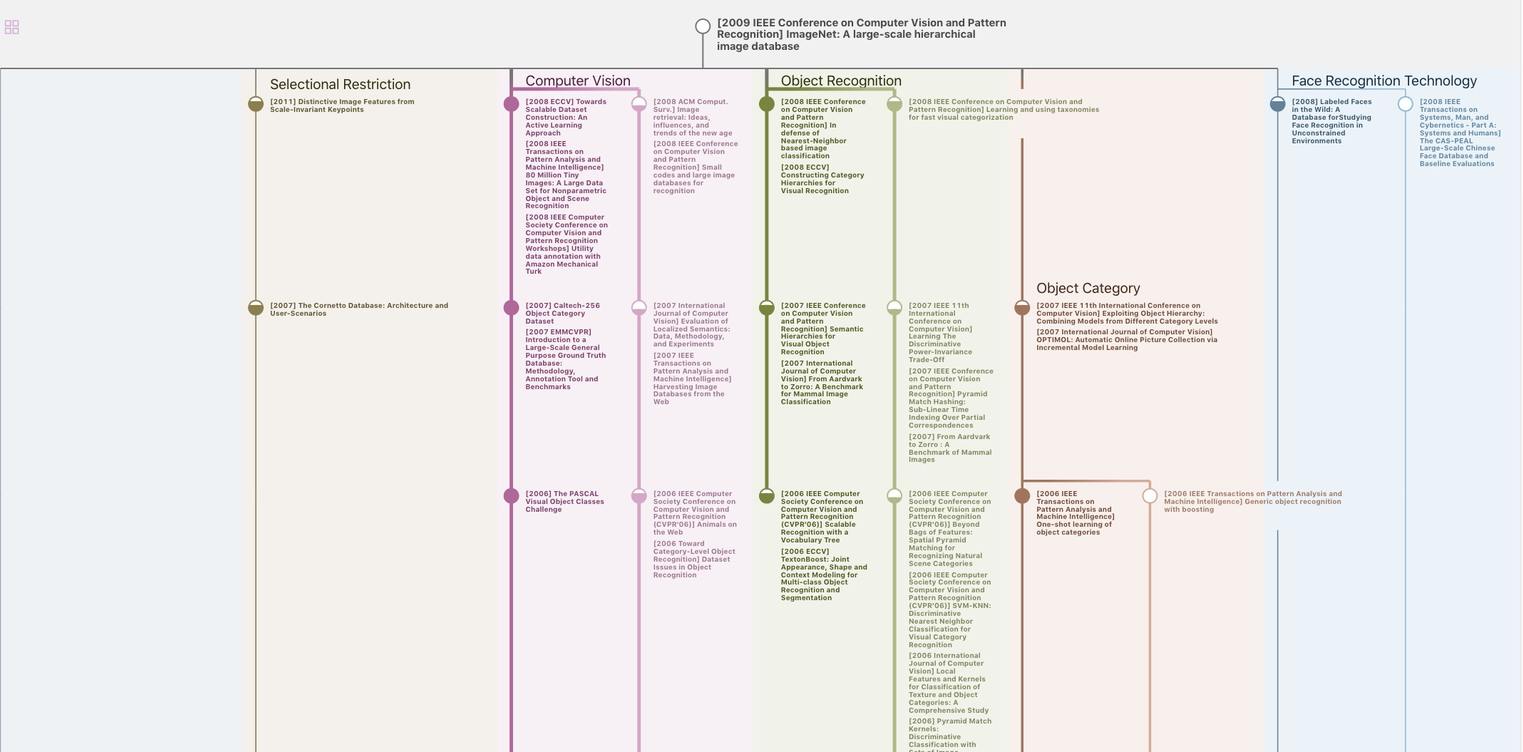
生成溯源树,研究论文发展脉络
Chat Paper
正在生成论文摘要