T-Rex: Optimizing Pattern Search on Time Series.
Proc. ACM Manag. Data(2023)
摘要
Pattern search is an important class of queries for time series data. Time series patterns often match variable-length segments with a large search space, thereby posing a significant performance challenge. The existing pattern search systems, for example, SQL query engines supporting MATCH_RECOGNIZE, are ineffective in pruning the large search space of variable-length segments. In many cases, the issue is due to the use of a restrictive query language modeled on time series points and a computational model that limits search space pruning. We built T-ReX to address this problem using two main building blocks: first, a MATCH_RECOGNIZE language extension that exposes the notion of segment variable and adds new operators, lending itself to better optimization; second, an executor capable of pruning the search space of matches and minimizing total query time using an optimizer. We conducted experiments using 5 real-world datasets and 11 query templates, including those from existing works. T-ReX outperformed an optimized NFA-based pattern search executor by 6x in median query time and an optimized tree-based executor by 19X.
更多查看译文
关键词
operator,pattern search,query optimizer,query processing,time series
AI 理解论文
溯源树
样例
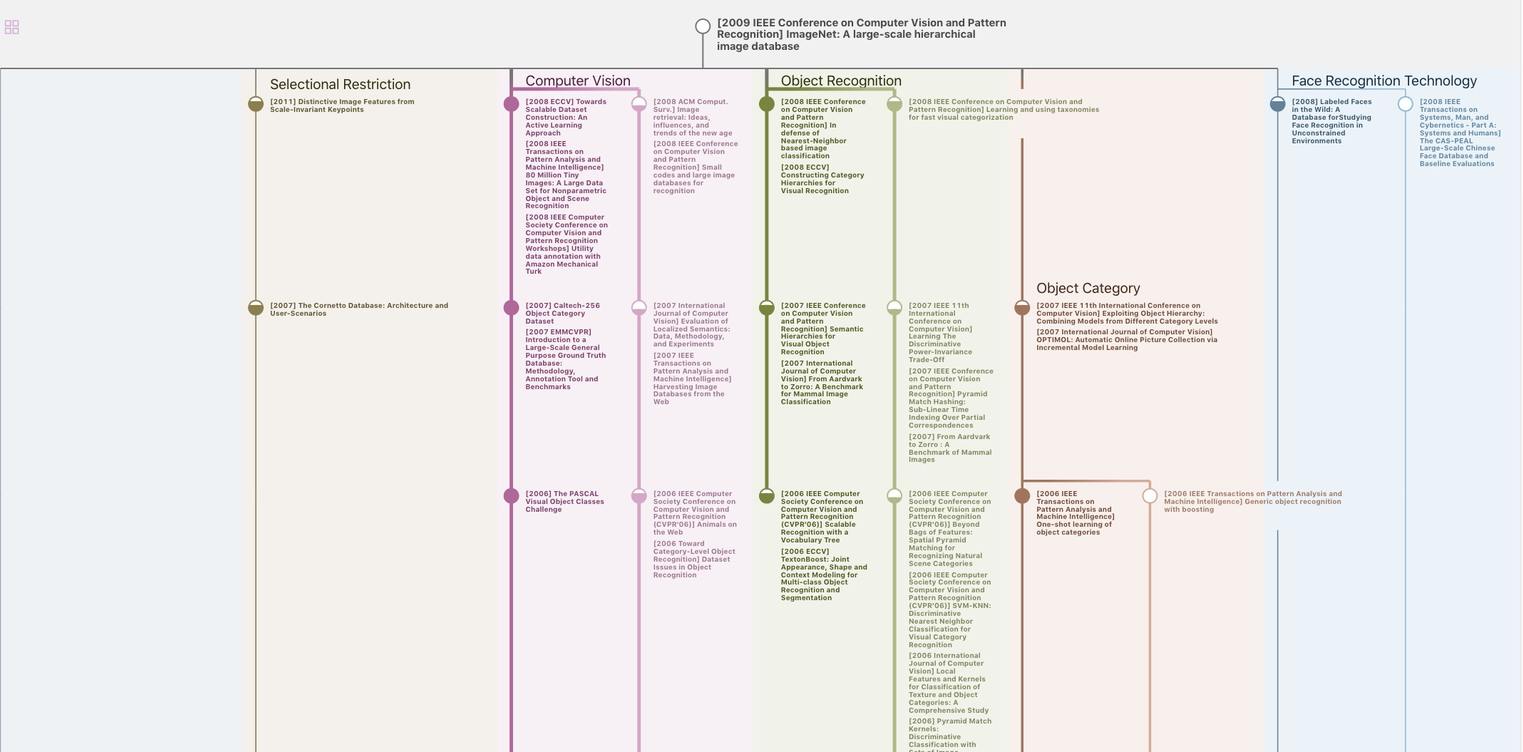
生成溯源树,研究论文发展脉络
Chat Paper
正在生成论文摘要