Efficient Neuromorphic Reservoir Computing Using Optoelectronic Memristors for Multivariate Time Series Classification.
Int. J. Bifurc. Chaos(2023)
摘要
Reservoir computing (RC) has attracted much attention as a brain-like neuromorphic computing algorithm for time series processing. In addition, the hardware implementation of the RC system can significantly reduce the computing time and effectively apply it to edge computing, showing a wide range of applications. However, many hardware implementations of RC use different hardware to implement standard RC without further expanding the RC architecture, which makes it challenging to deal with relatively complex time series tasks. Therefore, we propose a bidirectional hierarchical light reservoir computing method using optoelectronic memristors as the basis for the hardware implementation. The approach improves the performance of hardware-implemented RC by allowing the memristor to capture multilevel temporal information and generate a variety of reservoir states. Ag|GQDs|TiOx|FTO memristors with negative photoconductivity effects can map temporal inputs nonlinearly to reservoir states and are used to build physical reservoirs to accomplish higher-speed operations. The method's effectiveness is demonstrated in multivariate time series classification tasks: a predicted accuracy of 98.44% is achieved in voiceprint recognition and 99.70% in the mobile state recognition task. Our study offers a strategy for dealing with multivariate time series classification issues and paves the way to developing efficient neuromorphic computing.
更多查看译文
关键词
efficient neuromorphic reservoir computing,optoelectronic memristors,time series classification
AI 理解论文
溯源树
样例
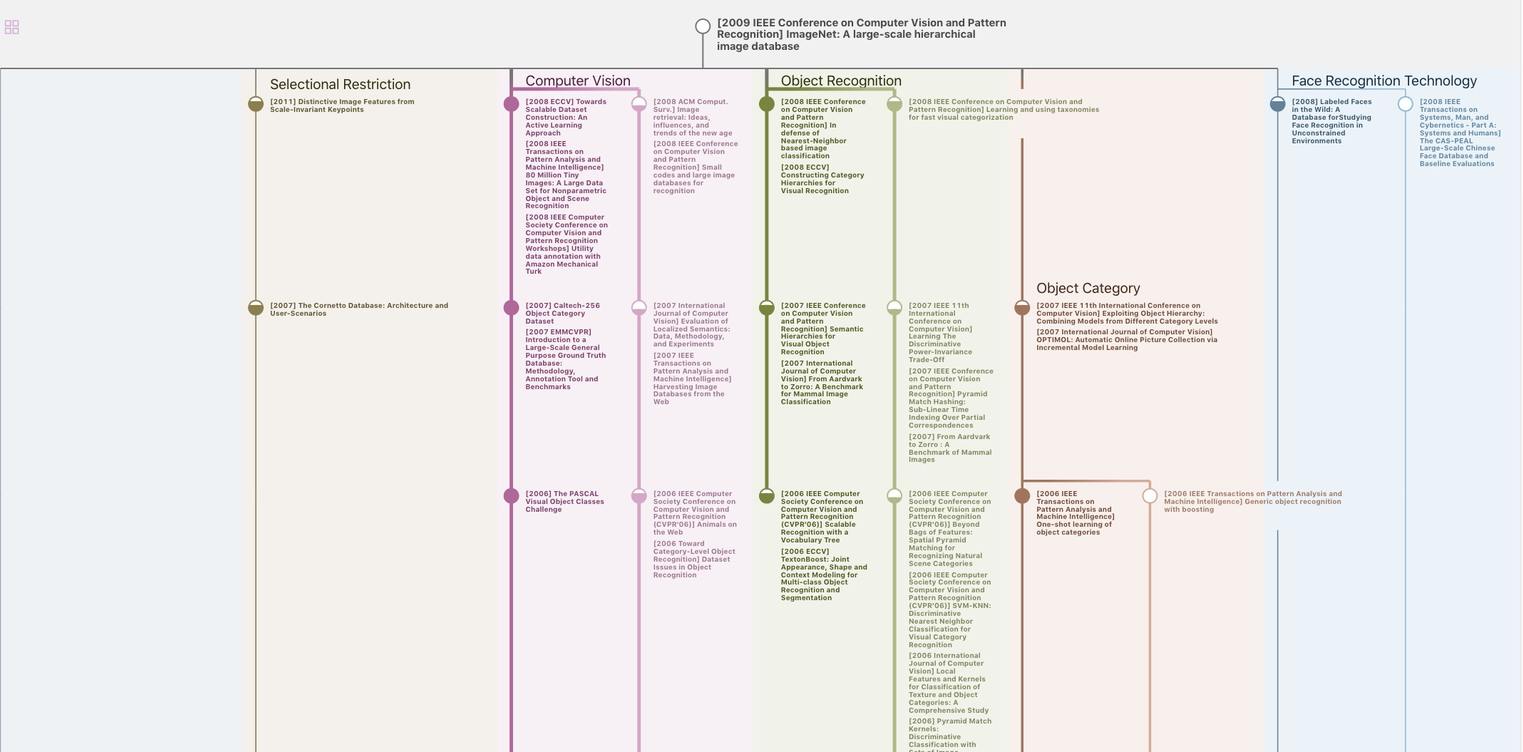
生成溯源树,研究论文发展脉络
Chat Paper
正在生成论文摘要