PointBoost: LiDAR-Enhanced Semantic Segmentation of Remote Sensing Imagery.
IEEE J. Sel. Top. Appl. Earth Obs. Remote. Sens.(2023)
摘要
Semantic segmentation of imagery is typically reliant on texture information from raster images, which limits its accuracy due to the inherently 2-D nature of the plane. To address the nonnegligible domain gap between different metric spaces, multimodal methods have been introduced that incorporate Light Detection and Ranging (LiDAR) derived feature maps. This converts multimodal joint semantic segmentation between 3-D point clouds and 2-D optical imagery into a feature extraction process for the 2.5-D product, which is achieved by concatenating LiDAR-derived feather maps, such as digital surface models, with the optical images. However, the information sources for these methods are still limited to 2-D, and certain properties of point clouds are lost as a result. In this study, we propose PointBoost, an effective sequential segmentation framework that can work directly with cross-modal data of LiDAR point clouds and imagery, which is able to extract richer semantic features from cross-dimensional and cross-modal information. Ablation experiments demonstrate that PointBoost can take full advantage of the 3-D topological structure between points and attribute information of point clouds, which is often discarded by other methods. Experiments on three multimodal datasets, namely N3C-California, ISPRS Vaihingen, and GRSS DFC 2018, show that our method achieves superior performance with good generalization.
更多查看译文
关键词
remote sensing imagery,remote sensing,segmentation,lidar-enhanced
AI 理解论文
溯源树
样例
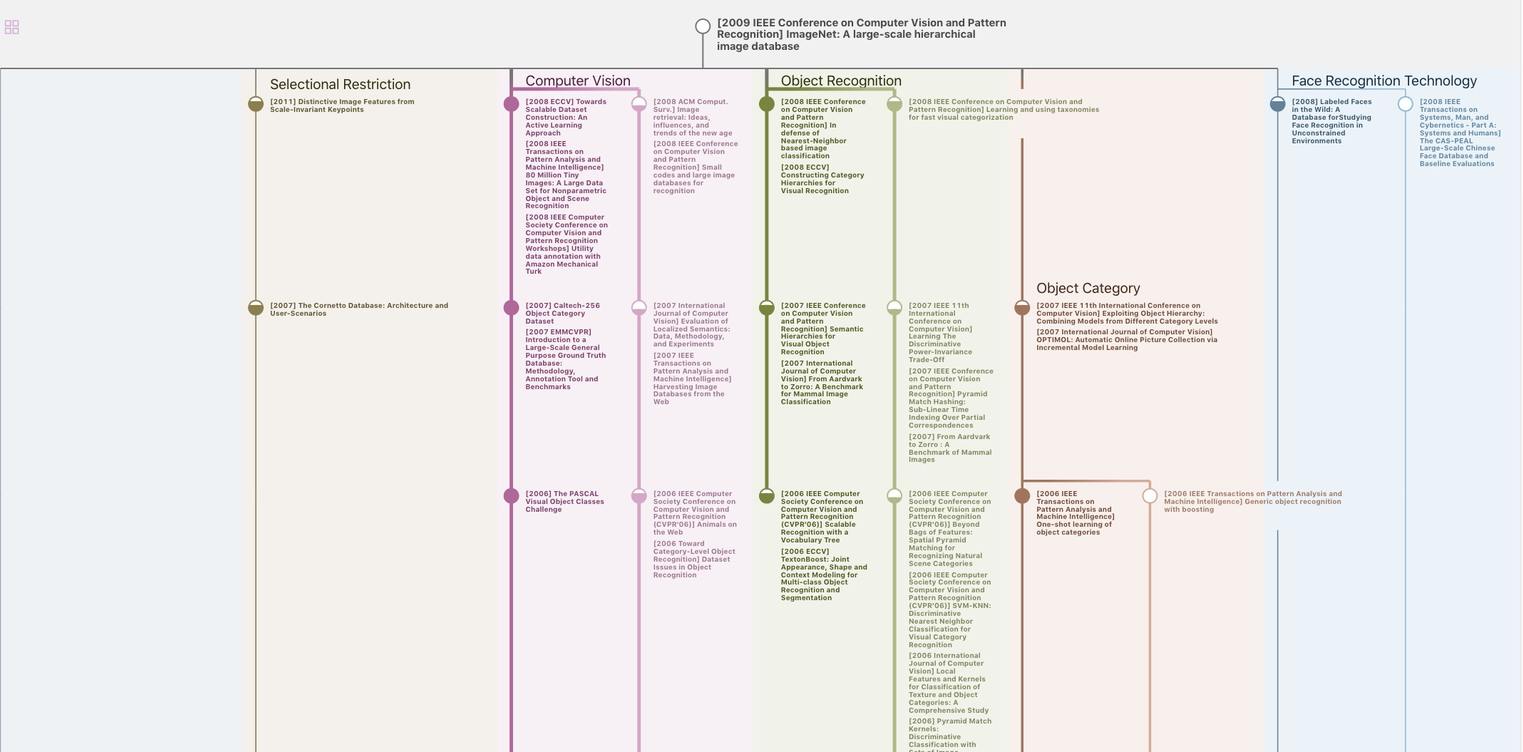
生成溯源树,研究论文发展脉络
Chat Paper
正在生成论文摘要