Crack growth degradation-based diagnosis and design of high pressure liquefied natural gas pipe via designable data-augmented anomaly detection.
J. Comput. Des. Eng.(2023)
摘要
A new approach to anomaly detection termed "anomaly detection with designable generative adversarial network (Ano-DGAN)" is proposed, which is a series connection of a designable generative adversarial network and anomaly detection with a generative adversarial network. The proposed Ano-DGAN, based on a deep neural network, overcomes the limitations of abnormal data collection when performing anomaly detection. In addition, it can perform statistical diagnosis by identifying the healthy range of each design variable without a massive amount of initial data. A model was constructed to simulate a high-pressure liquefied natural gas pipeline for data collection and the determination of the critical design variables. The simulation model was validated and compared with the failure mode and effect analysis of a real pipeline, which showed that stress was concentrated in the weld joints of the branch pipe. A crack-growth degradation factor was applied to the weld, and anomaly detection was performed. The performance of the proposed model was highly accurate compared with that of other anomaly detection models, such as support vector machine, 1D convolutional neural network, and long short-term memory. The results provided a statistical estimate of the design variable ranges and were validated statistically, indicating that the diagnosis was acceptable.
更多查看译文
关键词
liquefied natural gas pipeline, failure mode and effect analysis (FMEA), anomaly detection-designable generative adversarial network (Ano-DGAN), diagnosis-based design, statistical estimation
AI 理解论文
溯源树
样例
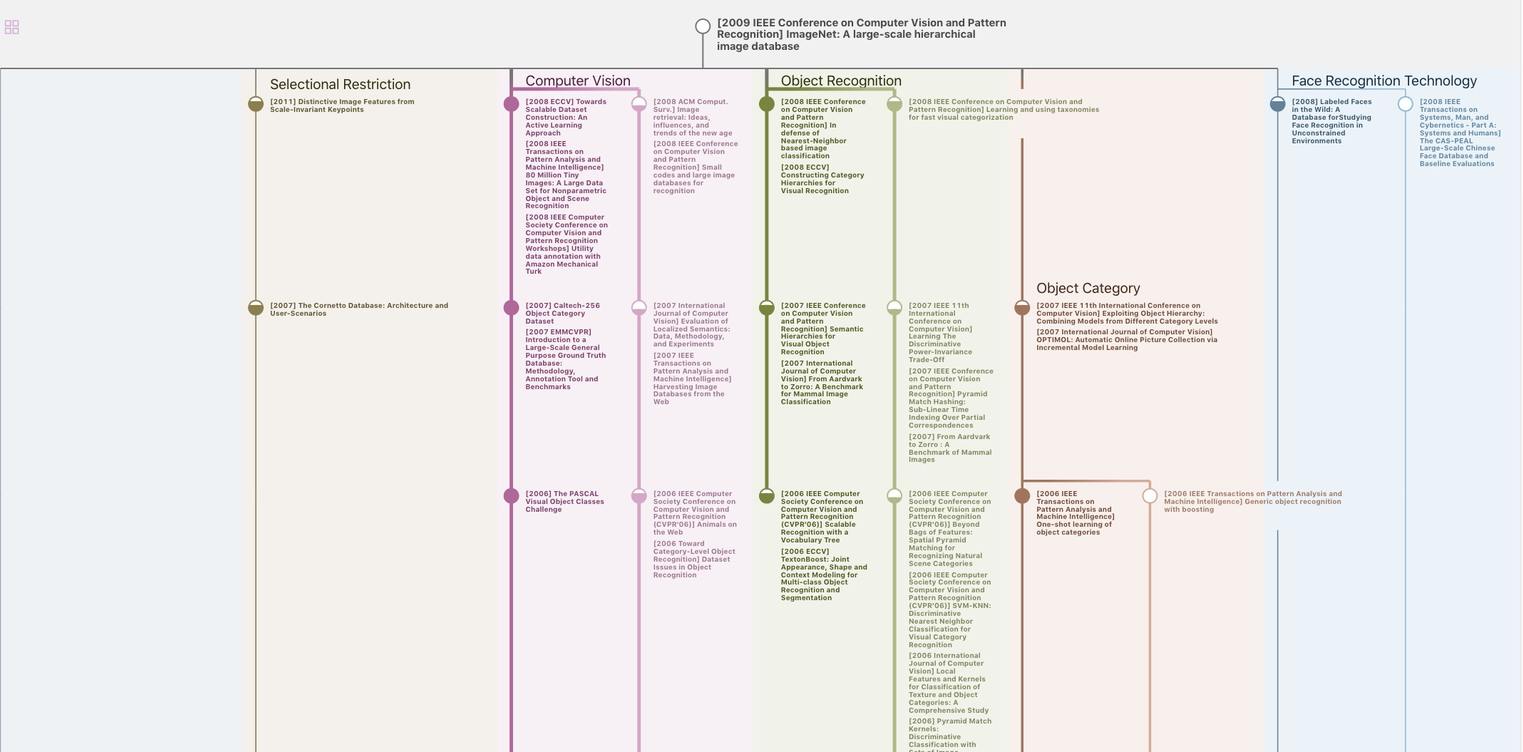
生成溯源树,研究论文发展脉络
Chat Paper
正在生成论文摘要