An Adaptive Deep Learning Neural Network Model to Enhance Machine-Learning-Based Classifiers for Intrusion Detection in Smart Grids.
Algorithms(2023)
摘要
Modern smart grids are built based on top of advanced computing and networking technologies, where condition monitoring relies on secure cyberphysical connectivity. Over the network infrastructure, transported data containing confidential information, must be protected as smart grids are vulnerable and subject to various cyberattacks. Various machine learning based classifiers were proposed for intrusion detection in smart grids. However, each of them has respective advantage and disadvantages. Aiming to improve the performance of existing machine learning based classifiers, this paper proposes an adaptive deep learning algorithm with a data pre-processing module, a neural network pre-training module and a classifier module, which work together classify intrusion data types using their high-dimensional data features. The proposed Adaptive Deep Learning (ADL) algorithm obtains the number of layers and the number of neurons per layer by determining the characteristic dimension of the network traffic. With transfer learning, the proposed ADL algorithm can extract the original data dimensions and obtain new abstract features. By combining deep learning models with traditional machine learning-based classification models, the performance of classification of network traffic data is significantly improved. By using the Network Security Laboratory-Knowledge Discovery in Databases (NSL-KDD) dataset, experimental results show that the proposed ADL algorithm improves the effectiveness of existing intrusion detection methods and reduces the training time, indicating a promising candidate to enhance network security in smart grids.
更多查看译文
关键词
deep learning, machine learning, intrusion detection, smart grid, neural networks
AI 理解论文
溯源树
样例
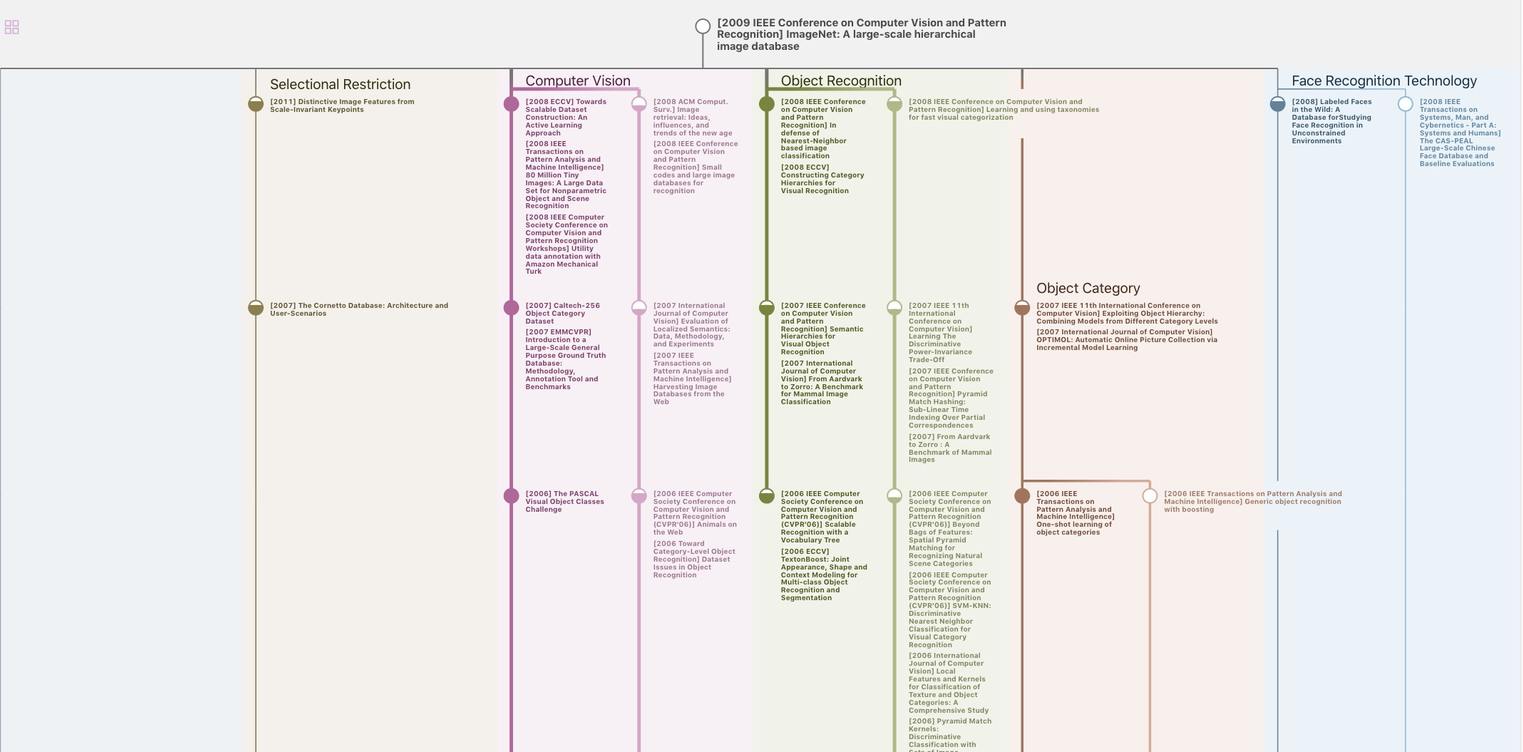
生成溯源树,研究论文发展脉络
Chat Paper
正在生成论文摘要