Boundary-Aware Supervoxel Segmentation for Indoor 3D Point Clouds.
IEEE Access(2023)
摘要
Supervoxels provide a natural and compact representation of 3D point clouds that enables operations to be performed on regions rather than on scattered points. However, most supervoxel segmentation methods generate supervoxels based on geometric dissimilarity among points and cannot preserve accurate structure boundaries when noise and outliers are present due to the uncertainty in the random sampling of representative points (RPs). This paper formulates indoor supervoxel segmentation as selecting RPs and matching each point with its RP simultaneously. The RPs are selected from nonboundary areas instead of randomly sampled points, guaranteeing the correctness of sampling from the same structure. The best point-to-RP matching is achieved through iterative refinement/clustering via an energy descent method, which ensures the optimal overall segmentation. Experimental tests on five publicly available datasets demonstrate that our method preserves indoor structure boundaries and small structures more effectively than other state-of-the-art methods, resulting in performance scores of approximately 0.65 for boundary recall.
更多查看译文
关键词
segmentation,3d,boundary-aware
AI 理解论文
溯源树
样例
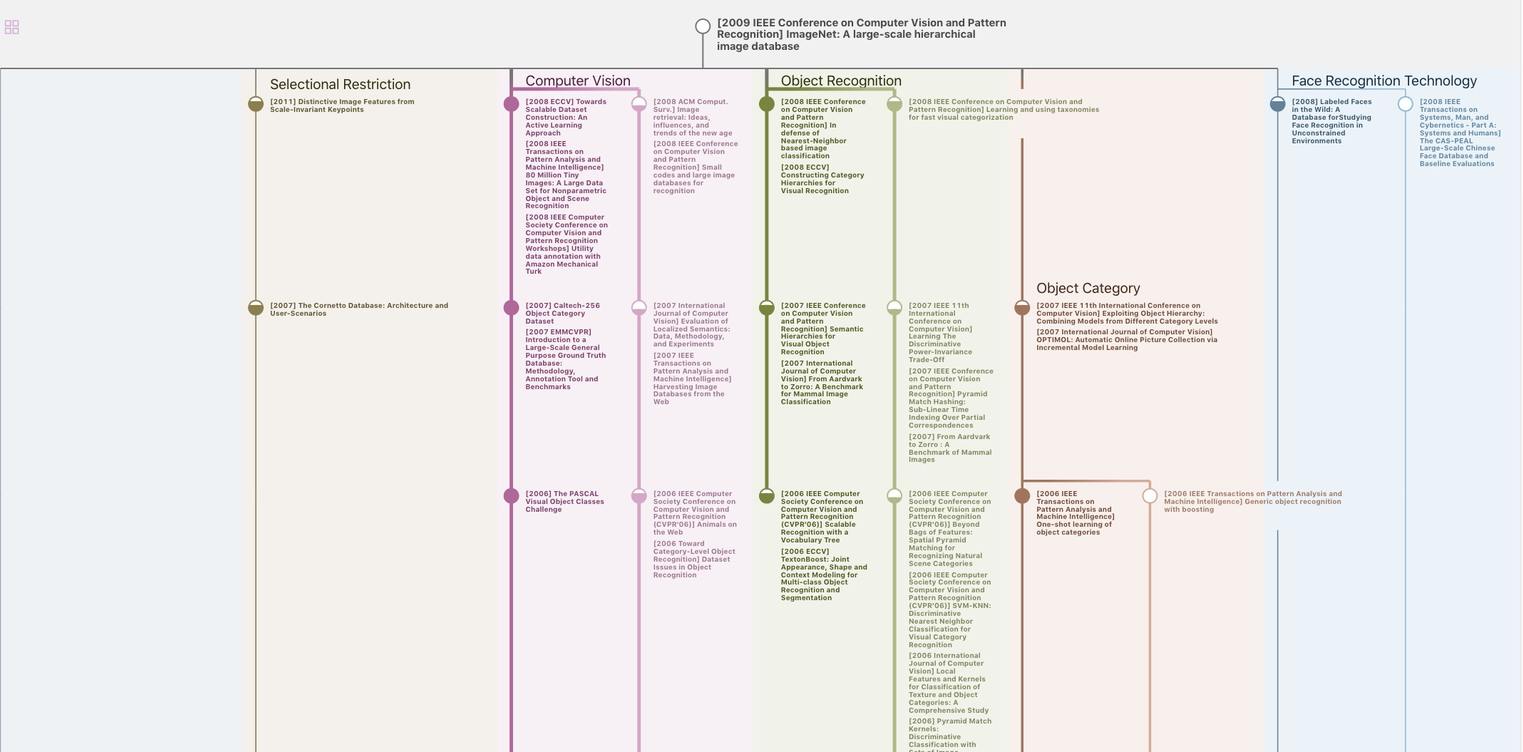
生成溯源树,研究论文发展脉络
Chat Paper
正在生成论文摘要