Intelligent Resource Management for eMBB and URLLC in 5G and Beyond Wireless Networks.
IEEE Access(2023)
摘要
In the era of 5G and beyond wireless networks, the simultaneous support of enhanced Mobile Broadband (eMBB) and Ultra-Reliable Low Latency Communications (URLLC) poses significant challenges in managing radio resources efficiently. By leveraging the puncturing technique, we propose an intelligent resource management framework for meeting the strict latency and reliability requirement of URLLC services and the high data rate for eMBB services. In particular, a semi-supervised learning and deep reinforcement learning (DRL) based architecture is proposed to manage the resources intelligently. We decompose the optimization problem into two subproblems: 1) resource block allocation (RBA) strategy for eMBB slice, and 2) URLLC scheduling. Through extensive simulations and performance evaluations, we demonstrate the effectiveness of the proposed technique in optimizing resource utilization, minimizing latency for URLLC users, and maximizing the throughput for eMBB services. Simulation findings demonstrate that the proposed methodology can ensure the URLLC reliability requirements while maintaining higher average sum rate for eMBB and higher convergence rate. The proposed framework paves the way for the efficient coexistence of diverse services, enabling wireless network operators to optimize resource allocation, improve user experience, and meet the specific requirements of eMBB and URLLC applications.
更多查看译文
关键词
5G,DNN,DRL,RAN slicing,eMBB,URLLC
AI 理解论文
溯源树
样例
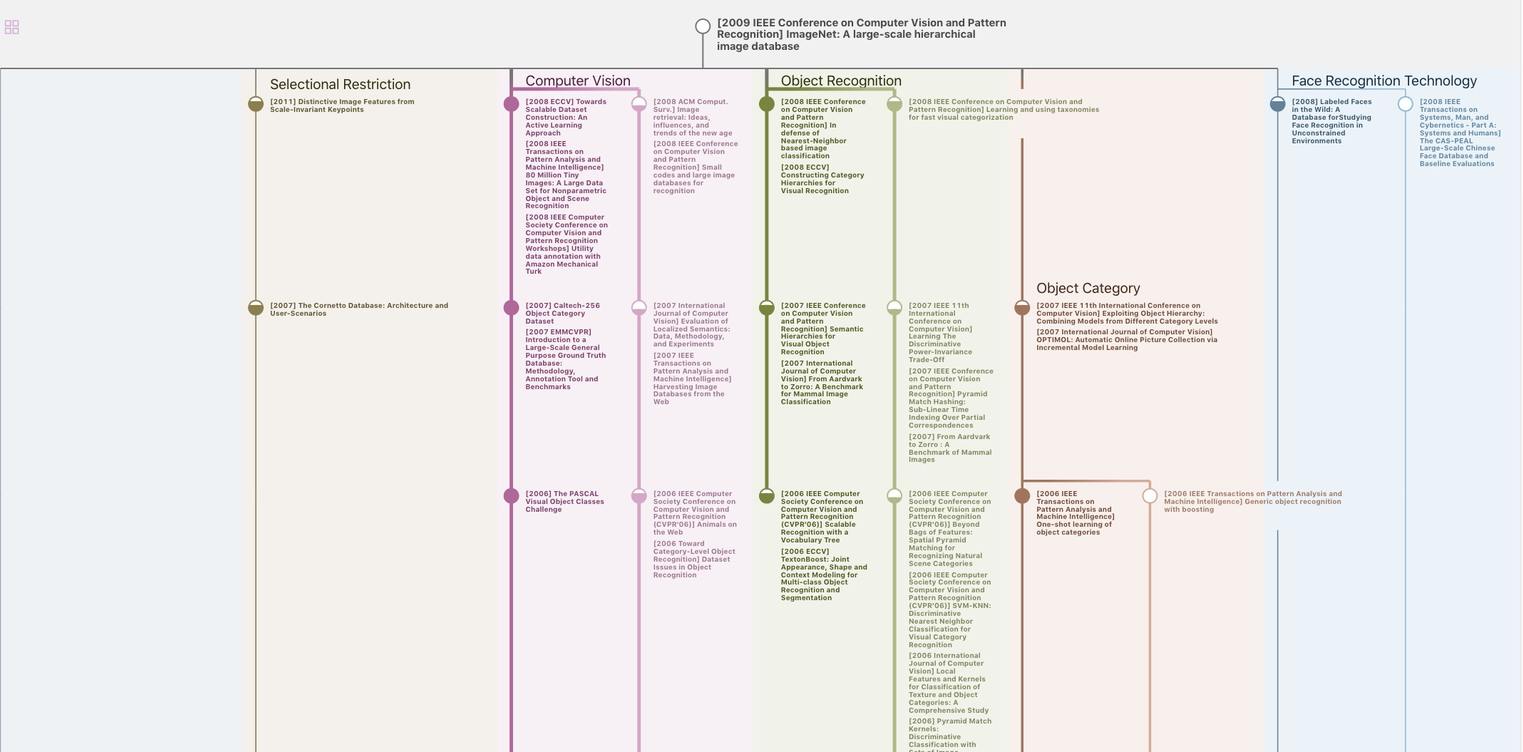
生成溯源树,研究论文发展脉络
Chat Paper
正在生成论文摘要