Any-Shot Learning From Multimodal Observations (ALMO).
IEEE Access(2023)
摘要
In this paper, we propose a framework (ALMO) for any-shot learning from multi-modal observations. Using training data containing both objects (inputs) and class attributes (side information) from multiple modalities, ALMO embeds the high-dimensional data into a common stochastic latent space using modality-specific encoders. Subsequently, a non-parametric classifier is trained to predict the class labels of the objects. We perform probabilistic data fusion to combine the modalities in the stochastic latent space and learn class conditional distributions for improved generalization and scalability. We formulate ALMO for both few-shot and zero-shot classification tasks, demonstrating significant improvement in recognition performance on the Omniglot and CUB-200 datasets as compared to state-of-the-art baselines.
更多查看译文
关键词
Prototypes, Task analysis, Machine learning, Data integration, Stochastic processes, Probabilistic logic, Transforms, Few-shot learning, zero-shot learning, multi-modal machine learning, probabilistic modeling
AI 理解论文
溯源树
样例
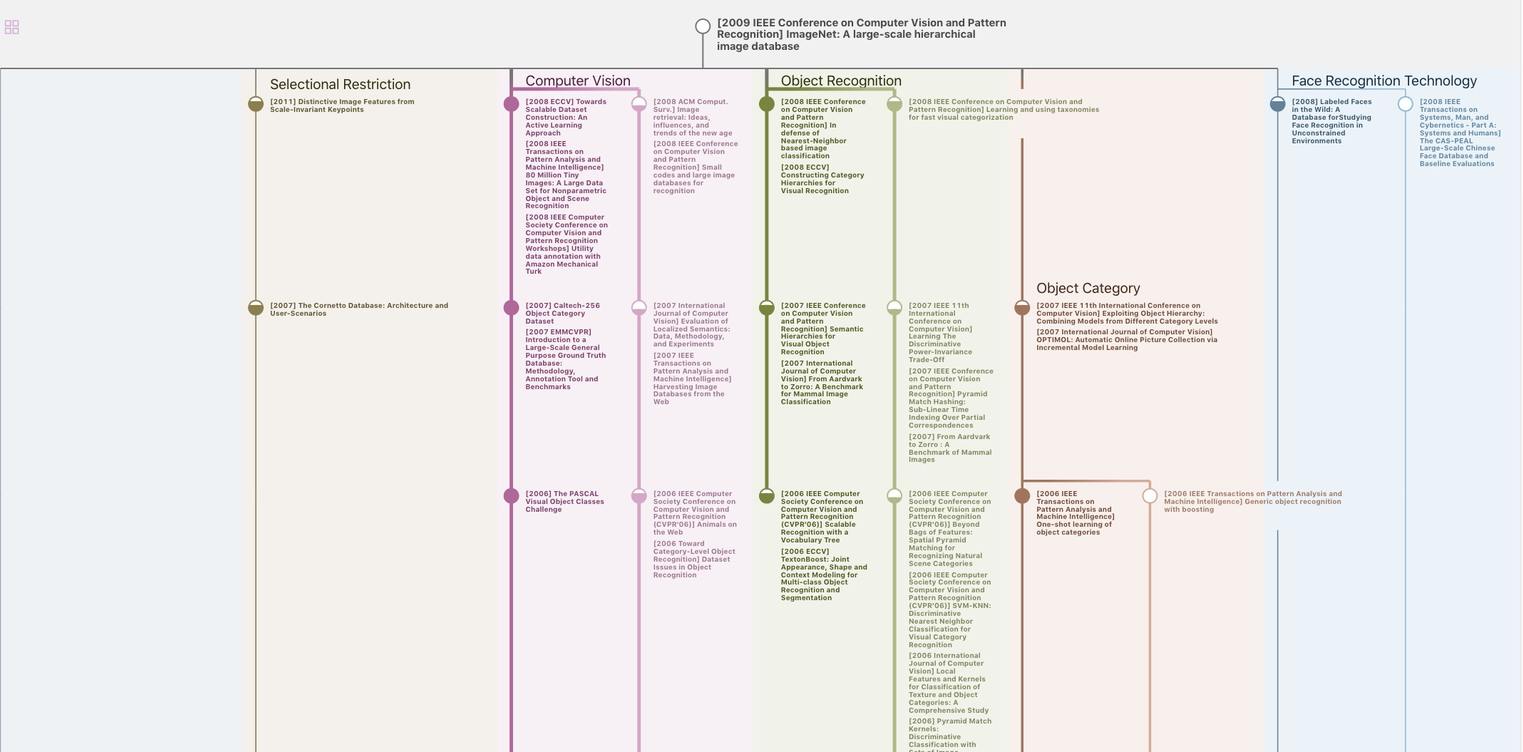
生成溯源树,研究论文发展脉络
Chat Paper
正在生成论文摘要