Multi-Camera 3D Object Detection for Autonomous Driving Using Deep Learning and Self-Attention Mechanism.
IEEE Access(2023)
摘要
In the absence of depth-centric sensors, 3D object detection using only conventional cameras becomes ill-posed and inaccurate due to the lack of depth information in the RGB image. We propose a multi-camera perception solution to predict the 3D properties of the vehicle obtained from the aggregated information from multiple static infrastructure-installed cameras. While a multi-bin regression loss has been adopted to predict the orientation of a 3D bounding box using a convolutional neural network, combining it with the geometrical constraints of a 2D bounding box to form a 3D bounding box is not accurate enough for all the driving scenarios and orientations. This paper leverages a vision transformer that overcomes the drawbacks of convolutional neural networks when there are no external LiDAR or pseudo-LiDAR pre-trained datasets available for depth map estimation, particularly in occluded regions. By combining the predicted 3D boxes from various cameras using an average weighted score algorithm, we determine the best bounding box with the highest confidence score. Comprehensive simulations for performance analysis are shown from the results obtained by utilizing the KITTI standard data generated from the CARLA simulator.
更多查看译文
关键词
Autonomous vehicles, deep learning, object detection, vision transformer
AI 理解论文
溯源树
样例
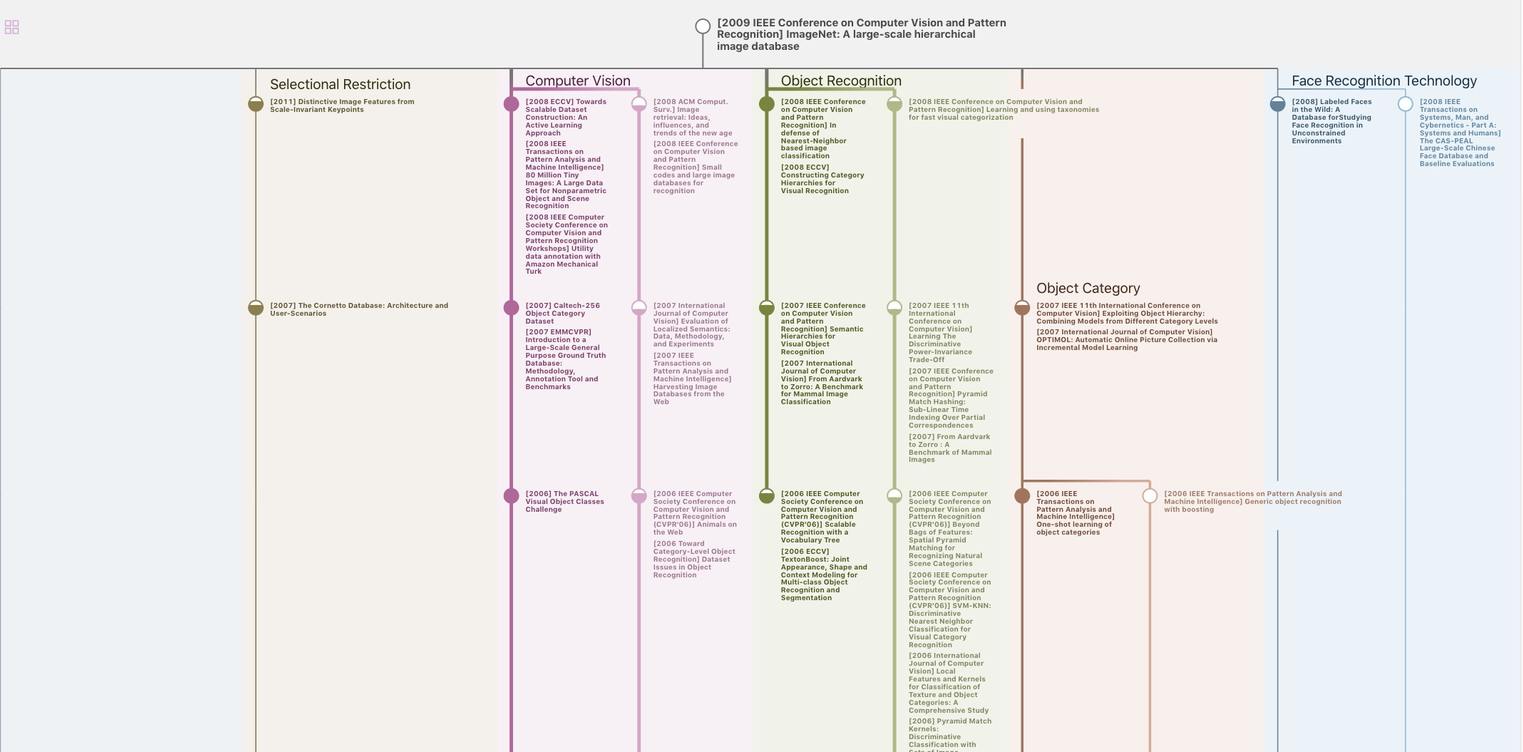
生成溯源树,研究论文发展脉络
Chat Paper
正在生成论文摘要