Explainable Machine Learning in Human Gait Analysis: A Study on Children With Cerebral Palsy.
IEEE Access(2023)
摘要
This work investigates the effectiveness of various machine learning (ML) methods in classifying human gait patterns associated with cerebral palsy (CP) and examines the clinical relevance of the learned features using explainability approaches. We trained different ML models, including convolutional neural networks, self-normalizing neural networks, random forests, and decision trees, and generated explanations for the trained models. For the deep neural networks, Grad-CAM explanations were aggregated on different levels to obtain explanations at the decision, class and model level. We investigate which subsets of 3D gait analysis data are particularly suitable for the classification of CP-related gait patterns. The results demonstrate the superiority of kinematic over ground reaction force data for this classification task and show that traditional ML approaches such as random forests and decision trees achieve better results and focus more on clinically relevant regions compared to deep neural networks. The best configuration, using sagittal knee and ankle angles with a random forest, achieved a classification accuracy of 93.4 % over all four CP classes (crouch gait, apparent equinus, jump gait, and true equinus). Deep neural networks utilized not only clinically relevant features but also additional ones for their predictions, which may provide novel insights into the data and raise new research questions. Overall, the article provides insights into the application of ML in clinical practice and highlights the importance of explainability to promote trust and understanding of ML models.
更多查看译文
关键词
human gait analysis,explainable machine learning,machine learning,cerebral palsy
AI 理解论文
溯源树
样例
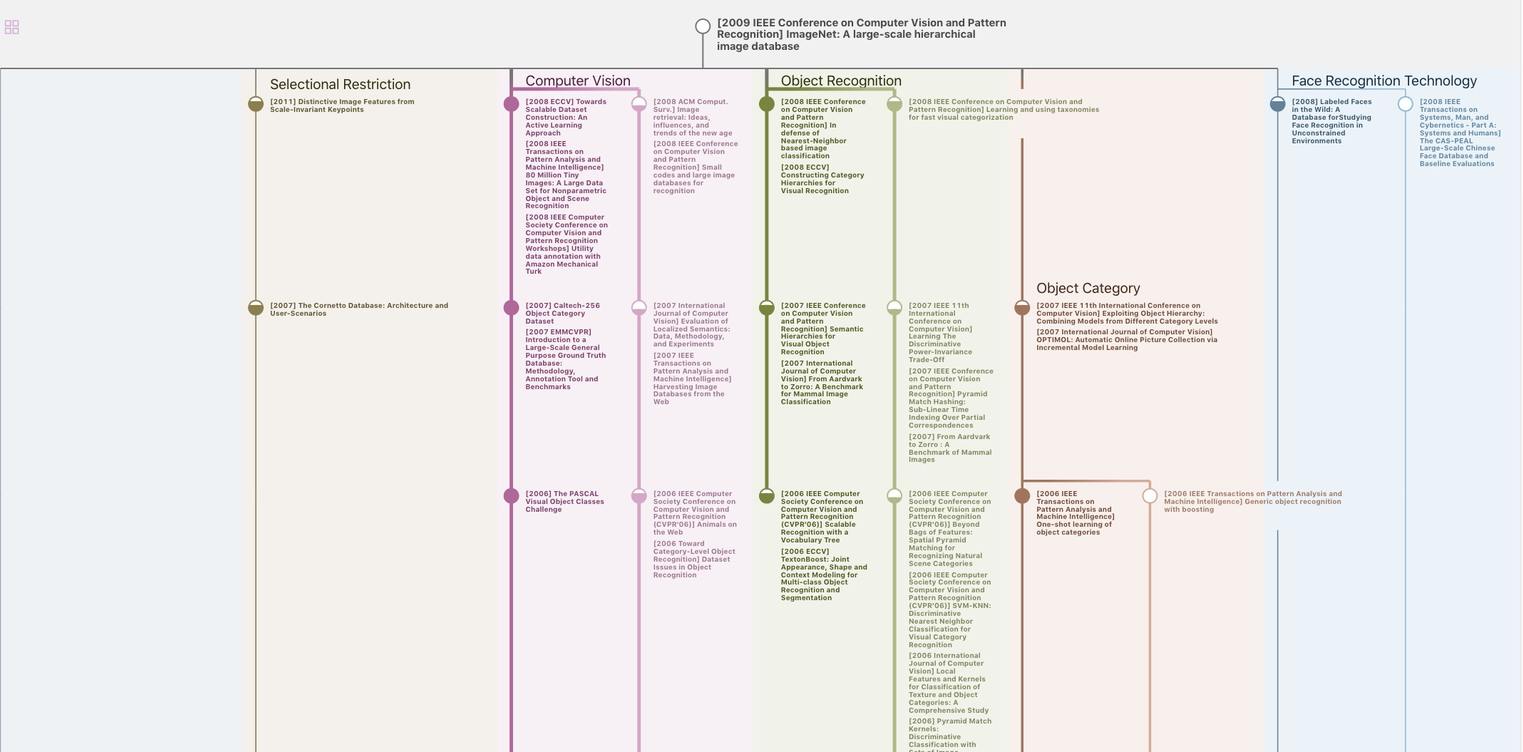
生成溯源树,研究论文发展脉络
Chat Paper
正在生成论文摘要