5G/B5G Network Slice Management via Staged Reinforcement Learning.
IEEE Access(2023)
摘要
The use of network slices to support different services in 5G/B5G networks is a key technology of 5G/B5G networks. Many flexible control methods have been used in admission control and resource management. However, resource management for network slicing is becoming increasingly complex due to the use of virtual technology to share physical resources. In this paper, we propose a deep reinforcement learning algorithm and a deep neural network control system to estimate future resource usage, select appropriate slice requests using a deep neural network strategy, and maximize rewards by interacting with the environment to address the aforementioned issue. Experimental results show that the proposed method achieves an average slice response ratio of 2.21, which is better than that of the Shortest Job First (SJF) algorithm and the random method. Additionally, the proposed method achieves an average slice response ratio that is 14.01% and 58.92% lower than SJF and random, respectively.
更多查看译文
关键词
5G, network slicing, reinforcement learning
AI 理解论文
溯源树
样例
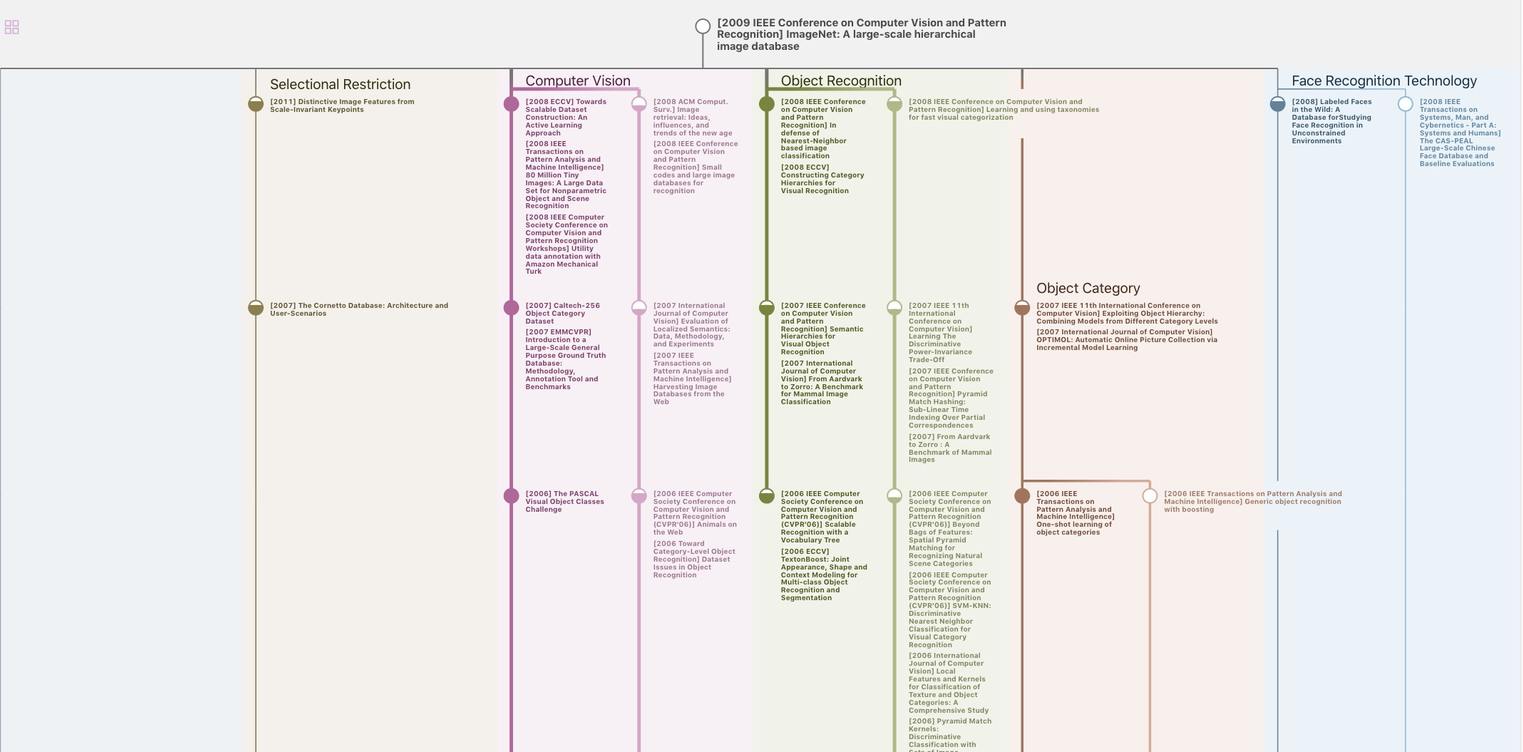
生成溯源树,研究论文发展脉络
Chat Paper
正在生成论文摘要