Attention Guided Policy Optimization for 3D Medical Image Registration.
IEEE Access(2023)
摘要
Learning-based image registration approaches typically learn to map from input images to a transformation matrix. Regarding the current deep-learning-based image rigid registration approaches learn a transformation matrix in a one-shot way. Our purpose is to present a deep reinforcement learning (DRL) based method for image registration to explicitly model the step-wise nature of the human registration process. We cast an image registration process as a Markov Decision Process (MDP) where actions are defined as global image adjustment operations. Then we train our proxy to learn the optimal action sequences to achieve a good registration. More specifically, we propose a DRL proxy incorporating an attention mechanism to address the challenge of large differences in appearance between images from different modalities. Registration experiments on 3D CT-MR image pairs of patients with nasopharyngeal carcinoma and on publicly available 3D PET-MR image pairs show that our approach significantly outperforms other methods, and achieves state-of-the-art performance in multi-m-modal medical image registration.
更多查看译文
关键词
INDEX TERMS Deep reinforcement learning, medical image, multi-m-modal registration, attention mechanism
AI 理解论文
溯源树
样例
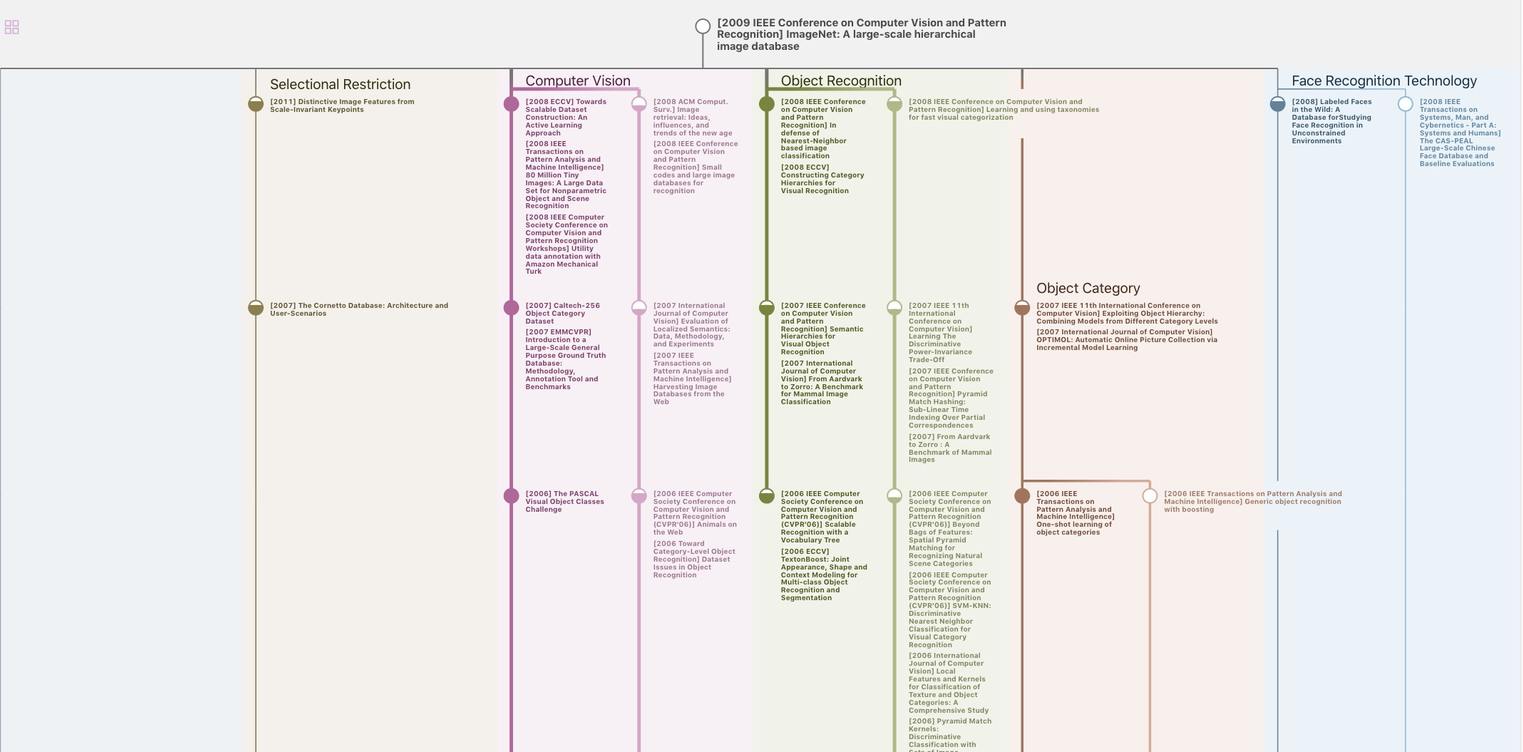
生成溯源树,研究论文发展脉络
Chat Paper
正在生成论文摘要