On the Effect of Log-Mel Spectrogram Parameter Tuning for Deep Learning-Based Speech Emotion Recognition.
IEEE Access(2023)
摘要
Speech emotion recognition (SER) has become a major area of investigation in human-computer interaction. Conventionally, SER is formulated as a classification problem that follows a common methodology: (i) extracting features from speech signals; and (ii) constructing an emotion classifier using extracted features. With the advent of deep learning, however, the former stage is integrated into the latter. That is to say, deep neural networks (DNNs), which are trained using log-Mel spectrograms (LMS) of audio waveforms, extract discriminative features from LMS. A critical issue, and one that is often overlooked, is that this procedure is done without relating the choice of LMS parameters to the performance of the trained DNN classifiers. It is commonplace in SER studies that practitioners assume some "usual" values for these parameters and devote major efforts to training and comparing various DNN architectures. In contrast with this common approach, in this work we choose a single lightweight pre-trained architecture, namely, SqueezeNet, and shift our main effort into tuning LMS parameters. Our empirical results using three publicly available SER datasets show that: (i) parameters of LMS can considerably affect the performance of DNNs; and (ii) by tuning LMS parameters, highly competitive classification performance can be achieved. In particular, treating LMS parameters as hyperparameters and tuning them led to similar to 23%, similar to 10%, and similar to 11% improvement in contrast with the use of "usual" values of LMS parameters in EmoDB, IEMOCAP, and SAVEE datasets, respectively.
更多查看译文
关键词
speech emotion recognition,log-mel,learning-based
AI 理解论文
溯源树
样例
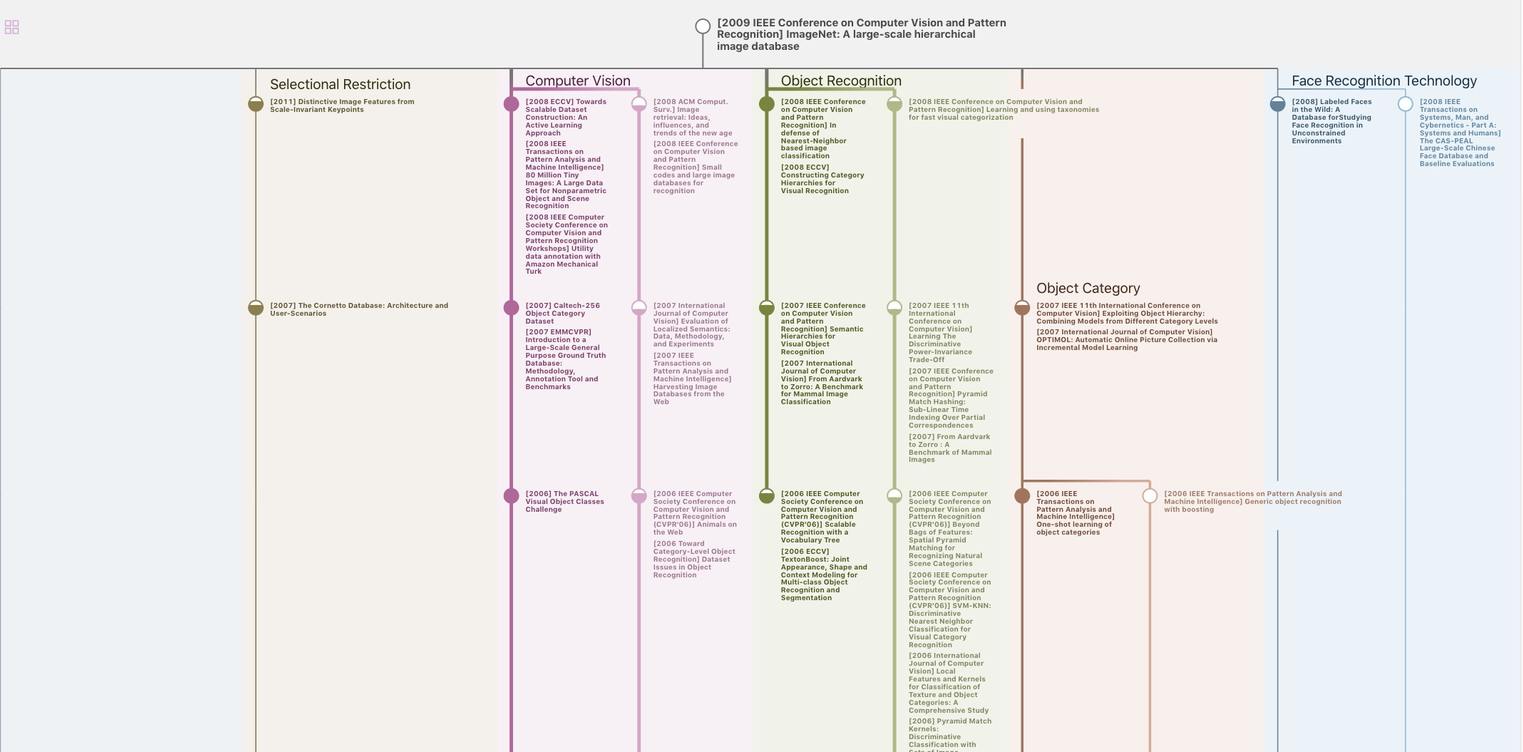
生成溯源树,研究论文发展脉络
Chat Paper
正在生成论文摘要