GAN-in-GAN for Monaural Speech Enhancement.
IEEE Signal Process. Lett.(2023)
摘要
Some generative adversarial networks (GANs) have been developed to remove background noise in real-world audio recordings. MetricGAN and its variants focus on generating a clean spectrogram from a noisy one, but the final audio quality can't be guaranteed. SEGAN and its variants directly generate an enhanced audio from a noisy one, but their over-long input representations make it less effective in identifying and removing audio noise. In this letter, a novel GAN-in-GAN framework is proposed, where the inner GAN conducts spectrogram-to-spectrogram recovery under the supervision of metric discriminators to effectively clean the audio noise, and the outer GAN conducts an audio-to-audio recovery under the supervision of multi-resolution discriminators to optimize the final audio quality. To tackle the challenges of utilizing multiple adversarial losses for training the proposed GAN-in-GAN simultaneously, a novel gradient balancing scheme is proposed to facilitate a coherent training. The proposed method is compared with state-of-the-art methods on the VoiceBank+DEMAND dataset for audio denoising. It outperforms all the compared methods.
更多查看译文
关键词
enhancement,gan-in-gan
AI 理解论文
溯源树
样例
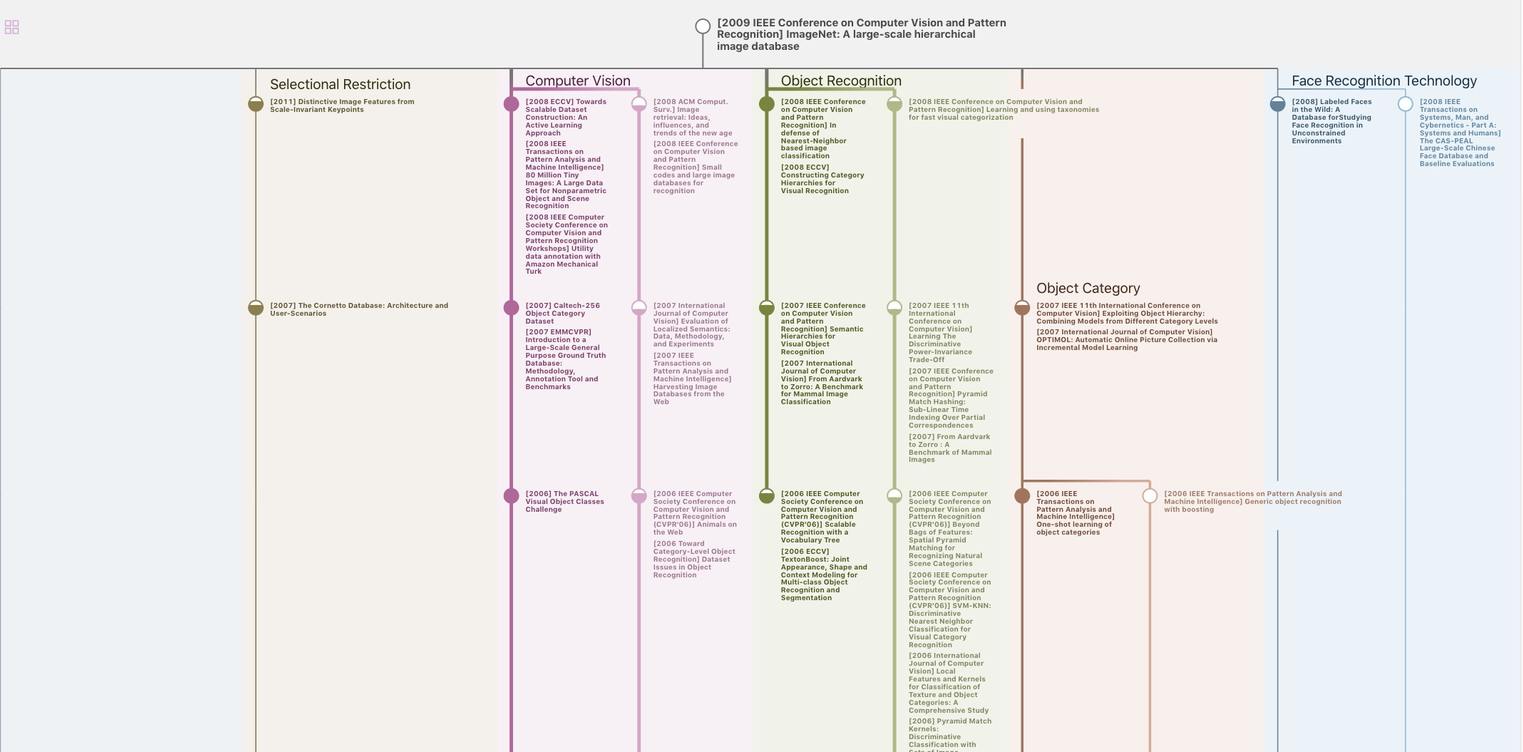
生成溯源树,研究论文发展脉络
Chat Paper
正在生成论文摘要