CoRe: Contrastive and Restorative Self-Supervised Learning for Surface Defect Inspection.
IEEE Trans. Instrum. Meas.(2023)
摘要
Visual inspection technology based on deep learning has achieved great success in surface defect inspection tasks. Most existing works transfer the learned knowledge from the natural dataset (e.g., ImageNet dataset) into the target tasks. However, the paradigm is suboptimal for defect inspection tasks due to: 1) the inherent dataset gap between natural and defect images and 2) the misalignment of task objectives. The limitations make the model cannot learn a generalized visual representation. To address the above issues, a contrastive and restorative self-supervised learning framework (CoRe) is proposed to learn general representation for the defect inspection task. Specifically, to bridge the dataset gap, we pretrain the model on the related dataset with a similar feature distribution instead of the natural dataset, which facilitates the representation learning of defect inspection tasks. Subsequently, to mitigate the task misalignment, the proposed method combines contrastive and restorative learning to excavate features required for the defect inspection task. The experimental results on five surface defect datasets demonstrate that our method outperforms the existing self-supervised learning (SSL) works, typical supervised pretraining paradigm, and some specific defect inspection methods.
更多查看译文
关键词
inspection,learning,surface,self-supervised
AI 理解论文
溯源树
样例
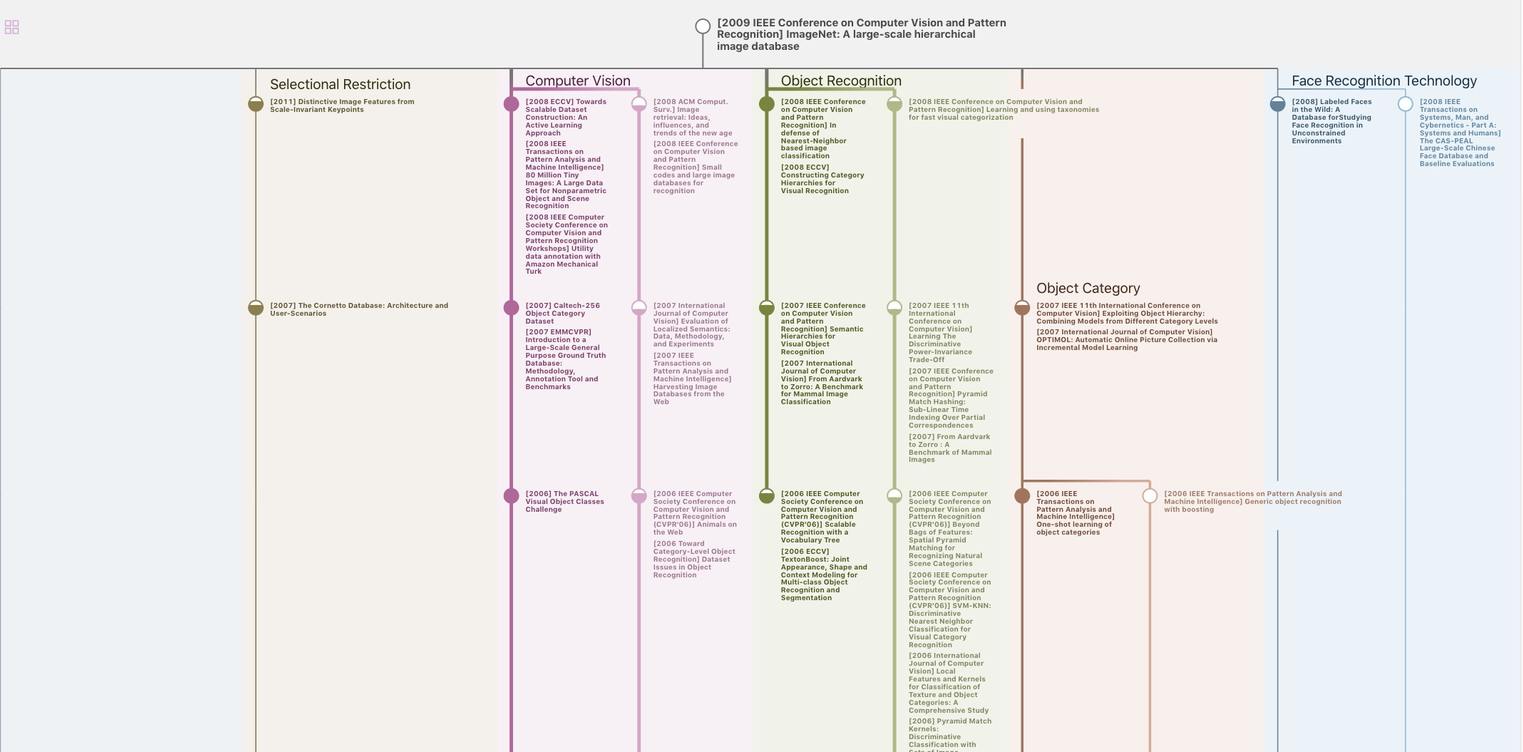
生成溯源树,研究论文发展脉络
Chat Paper
正在生成论文摘要