Unsupervised Palmprint Image Quality Assessment via Pseudo-Label Generation and Ranking Guidance.
IEEE Trans. Instrum. Meas.(2023)
摘要
Research on palmprint recognition has been progressing toward a contactless and unconstrained direction, where the imaging quality is easily disturbed by various factors. Determining the trustworthiness of palmprint image input to the system is crucial for stable and reliable recognition. However, there are few studies on palmprint image quality assessment (PIQA) for practical scenarios due to the lack of labeled image quality data. In this article, we propose a pseudo-label generation and ranking guidance (PGRG) framework for label-free PIQA. Two-stage preparation is performed before the training of quality model: 1) estimate the recognizability of palmprint images to generate quality pseudo-labels and 2) generate pseudoimages and guide their quality ranking to pretrain the model. In the final training phase, we propose a label-based ranking loss. It guides the model to determine quality relative relationships by emphasizing the ranking between pseudo-labels. This allows our framework to be applied to a variety of palmprint recognition models. Adequate experiments are conducted on three benchmark unconstrained palmprint databases. The results show that our PGRG framework can effectively improve the performance of the existing advanced palmprint recognition models. The equal error rate (EER) can be reduced by up to 2.41% by discarding a small amount of low-quality images compared with the baseline.
更多查看译文
关键词
quality,pseudo-label
AI 理解论文
溯源树
样例
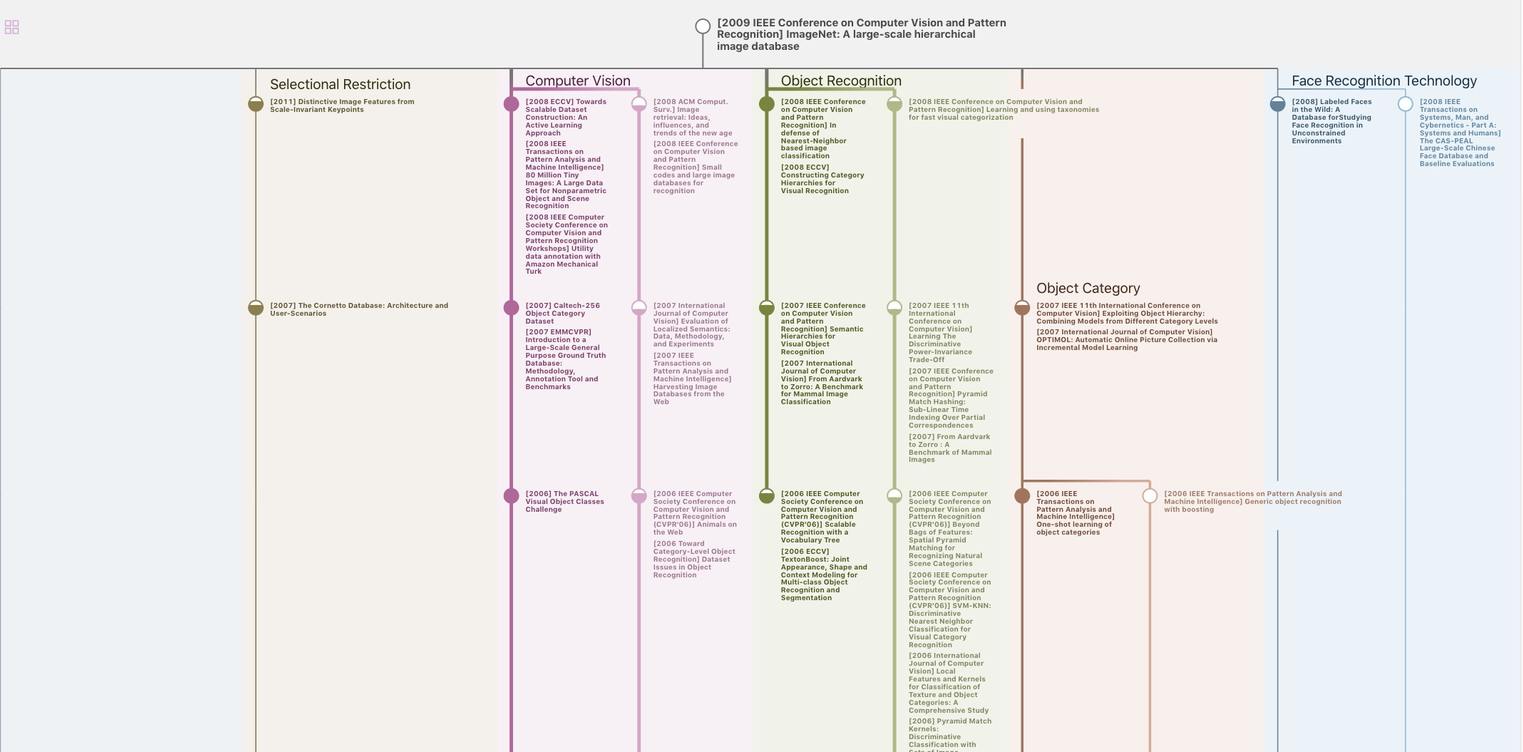
生成溯源树,研究论文发展脉络
Chat Paper
正在生成论文摘要