Local Enhancing Transformer With Temporal Convolutional Attention Mechanism for Bearings Remaining Useful Life Prediction.
IEEE Trans. Instrum. Meas.(2023)
摘要
Deep-learning (DL)-based remaining useful life (RUL) prognostics have achieved prominent advancements to maintain the reliability and safety of industrial equipment. The run-to-failure condition monitoring data of machinery generally takes the form of a long life-cycle sequence containing long and short-term latent degradation patterns, which requires DL models possessing both global and local modeling abilities for RUL prediction. Nevertheless, most existing DL approaches are still inadequate for capturing precise long-time dependencies and grasping local information synchronously. To address this challenge, this article proposes a novel multiscale temporal convolutional transformer (MTCT) to simultaneously extract long-term degradation features and local contextual associations directly from raw monitoring data. It has two distinctive characteristics. First, a convolutional self-attention (CsA) mechanism is developed to insert dilated causal convolution (DCC) into the self-attention mechanism so that the modeling of local context can be incorporated into global modeling to capture more accurate long-term dependency coupling and mitigate the influences of stochastic noises. Second, a temporal convolutional network (TCN) attention module combing TCN and squeeze-and-excitation (SE) attention is designed to select more important degradation-relation features and improve the local representation learning ability. Afterward, an end-to-end RUL prognostic framework based on the MTCT is established for bearings. Comparative studies and ablation experiments are carried out on a real-world dataset of bearings to demonstrate the effectiveness and superiority of the proposed method.
更多查看译文
关键词
Convolutional self-attention (CsA) mechanism,health assessment,remaining useful life (RUL),temporal convolutional network (TCN),transformer
AI 理解论文
溯源树
样例
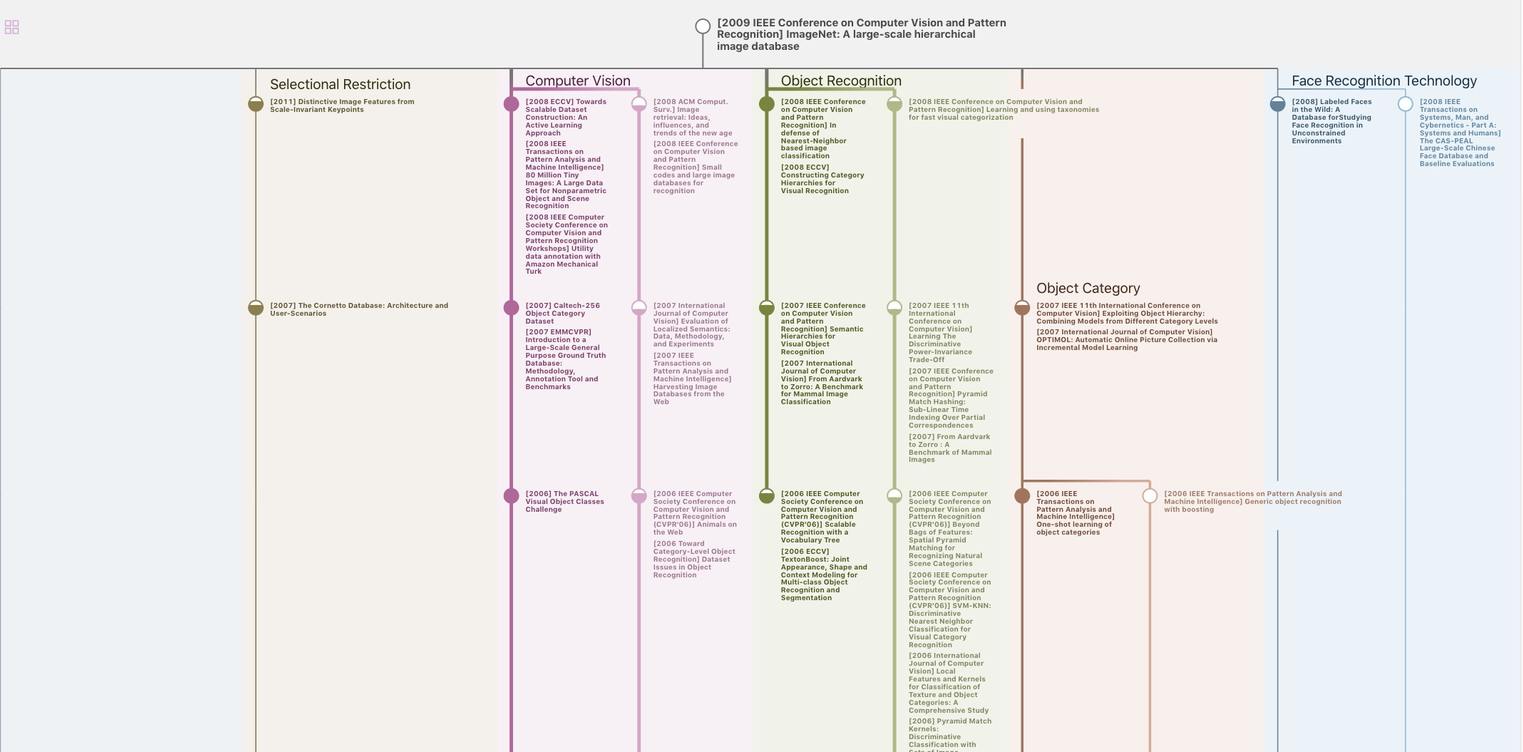
生成溯源树,研究论文发展脉络
Chat Paper
正在生成论文摘要