Demonstration Learning and Generalization of Robotic Motor Skills Based on Wearable Motion Tracking Sensors.
IEEE Trans. Instrum. Meas.(2023)
摘要
Learning from demonstration (LfD) is an important way for robots to learn new skills. Its principle is to obtain an optimized trajectory through an instructor's action teaching or action coding regression. By learning the teaching trajectory, the robot can master the movement skills. However, adapting the learned motor skills to meet additional constraints that arise during the task can be challenging. This article proposed a noise exploration method policy improvement through black-box optimization with an adaptive covariance matrix (PIBB-CMA), which improves the PIBB algorithm by using an adaptive covariance matrix. In our method, the teaching trajectory is collected with our designed inertial motion capture system. After that, a more reasonable trajectory is synthesized as the input to dynamic movement primitives (DMPs) using the Gaussian mixture model (GMM) and Gaussian mixture regression (GMR). Finally, the improved algorithm PIBB-CMA is used to perform noise exploration to ensure that the robot arm can pass the designated points in the path planning. Furthermore, three baseline methods are chosen for comparison to validate the robustness of our proposed method. The experimental results demonstrated that our proposed method outperforms the baseline algorithms and is feasible for improving robot intelligence. We believe that our method has a certain potential in the aspect of skill learning for robots.
更多查看译文
关键词
~Adaptive covariance matrix, dynamic movement primitives (DMPs), learning from demonstration (LfD), path planning, policy improvement through black-box optimization (PIBB)
AI 理解论文
溯源树
样例
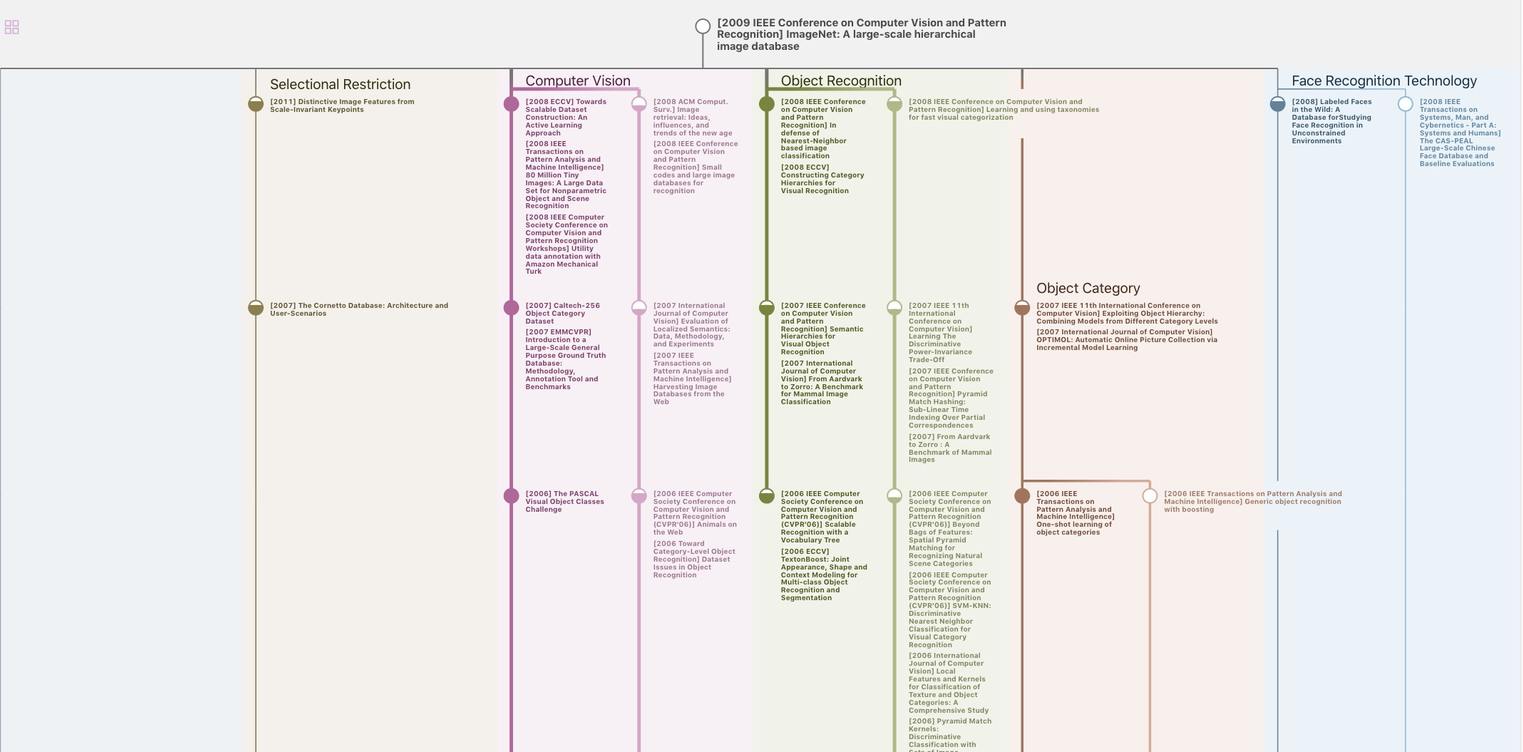
生成溯源树,研究论文发展脉络
Chat Paper
正在生成论文摘要