Low-Cost Adaptive LS-DEKF for SOC Estimation and RDT Prediction With SFP Model.
IEEE Trans. Instrum. Meas.(2023)
摘要
Simplified first principle (SFP) lithium-ion battery model has high accuracy in state-of-charge (SOC) estimation and remaining-dischargeable-time (RDT) prediction. However, SFP model also has high computation and puts challenges for real-time applications. To this end, a Lebesgue sampling (LS)-based method was proposed to reduce the computation of the SFP model without sacrificing the accuracy. The LS-based methods are event-based, in which algorithms are executed only when necessary. The main challenge of the LS-based method is the low convergence speed of SOC estimation and RDT prediction, especially when the batteries are under dynamic loading profiles. This article, therefore, proposes a low-cost solution that integrates adaptation of the Lebesgue length and dichotomy with the LS-based method, which is implemented with extended Kalman filter (EKF). The novelty of the proposed adaptive LS-based dichotomy with EKF (ALS-DEKF) is twofold: 1) it fuses the dichotomy and EKF in the LS framework to improve the accuracy and convergence speed and 2) it optimizes the Lebesgue length according to the battery load to accommodate the dynamic loading profiles. The proposed ALS-DEKF is then verified with a series of experiments under dynamic operating conditions. Experimental results and comparison studies show that ALS-DEKF can achieve fast convergence speed and high accuracy under dynamic loading profiles for SOC estimation and RDT prediction.
更多查看译文
关键词
Dichotomy, extended Kalman filter (EKF), Lebesgue sampling (LS), remaining-dischargeable-time (RDT), simplified first principle (SFP), state-of-charge (SOC)
AI 理解论文
溯源树
样例
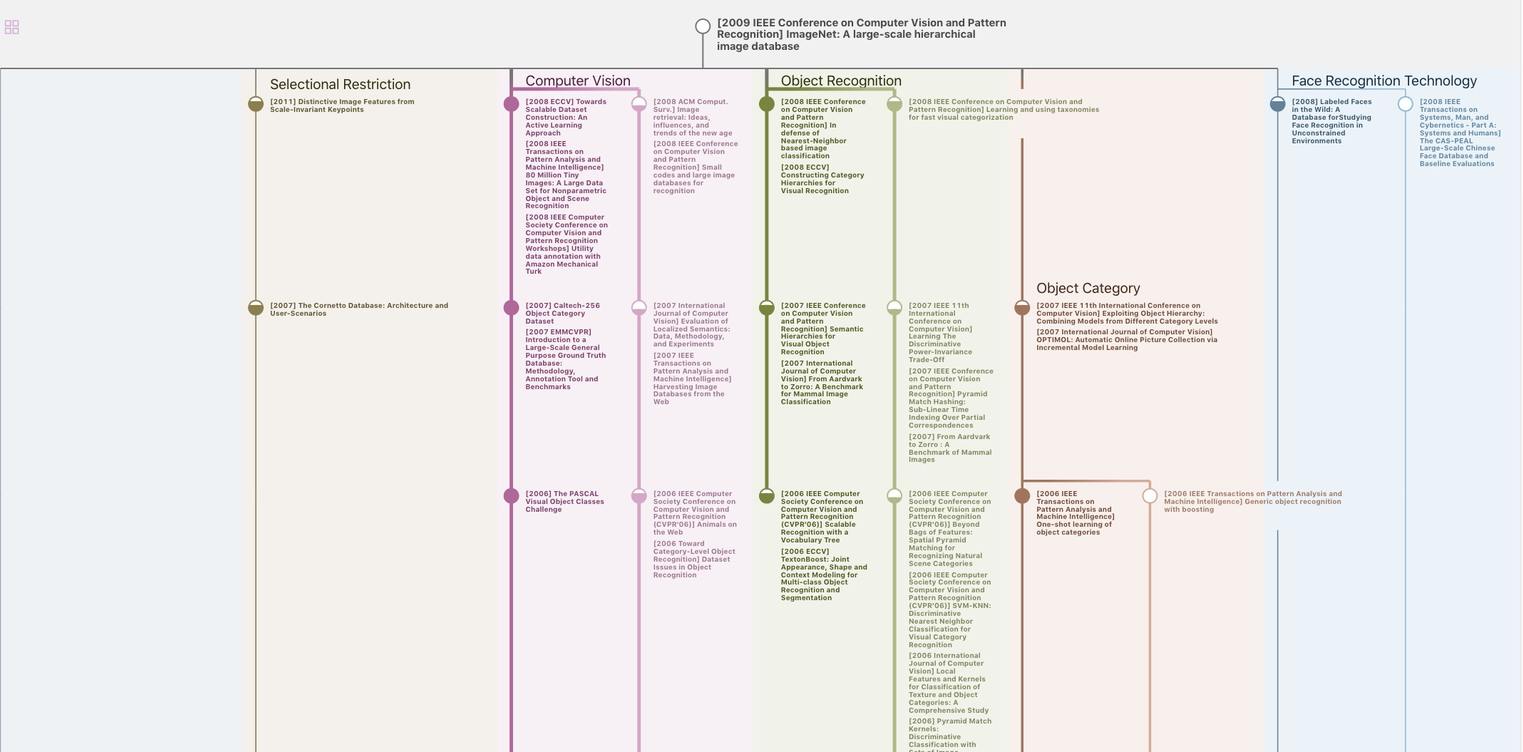
生成溯源树,研究论文发展脉络
Chat Paper
正在生成论文摘要