Lambertian Reflection Separation Under High Reflectiveness for Worn Surface Reconstruction With Insufficient Samples.
IEEE Trans. Instrum. Meas.(2023)
摘要
Compared with 2-D images, 3-D image-based worn inspection can provide more profound information regarding the in situ endoscope examination of mechanical parts. With photometric stereo vision (PSV), 3-D topography can be reconstructed from 2-D images with minimal cost. However, this promising technology is limited by the imprecision in calculating normal vector due to the high reflectiveness of worn surfaces. An innovative model is here developed to separate Lambertian reflection from photometric images. The Lambertian reflection variations are estimated by fusing multiple photometric images, while the constants are extracted from the general plane base of worn surfaces. Considering a small sample set of worn surfaces, hybrid samples, including the real and simulated images, are adopted for parameter optimization with feature-level alignment (FA). Moreover, a label-free prediction loss (LFL) function is constructed to constrain the optimization direction via real samples. The constructed model is obtained from training 20000 simulated samples and 1000 real samples. The results indicate that high reflectiveness can be addressed by separating the Lambertian reflections. Comparatively, the model can improve the normal vector calculation with lower average error than other existing algorithms.
更多查看译文
关键词
Lambertian reflection separation,photometric stereo vision (PSV),semi-supervised learning,worn surface reconstruction
AI 理解论文
溯源树
样例
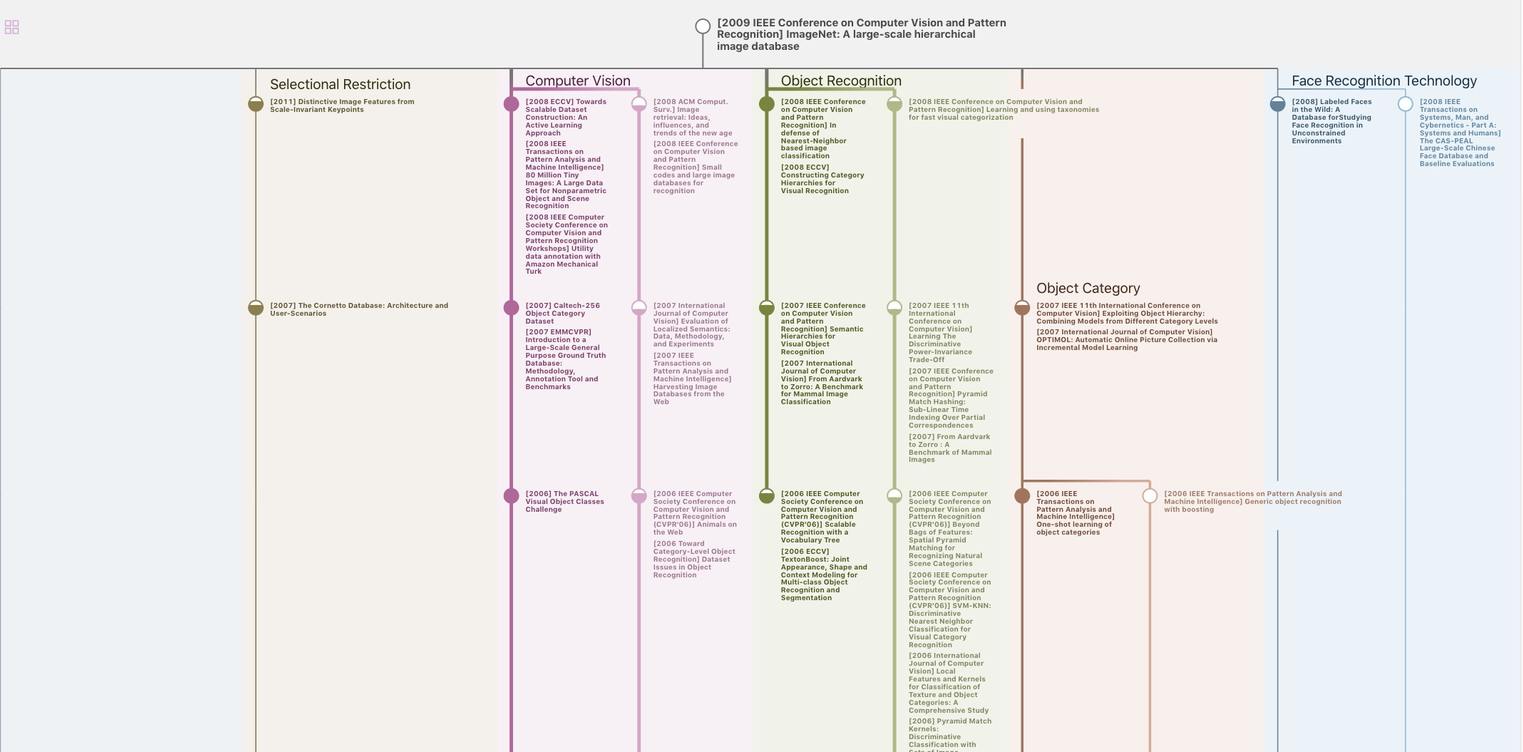
生成溯源树,研究论文发展脉络
Chat Paper
正在生成论文摘要