Lane-Level and Full-Cycle Multivehicle Tracking Using Low-Channel Roadside LiDAR.
IEEE Trans. Instrum. Meas.(2023)
摘要
The ability to track multiple objects is crucial for roadside units to provide high-precision, trajectory-based traffic data, especially for connected vehicles that require complementary and long-range traffic information to improve road safety. Occlusion and continuous tracking are major challenges that have hindered the achievement of obtaining accurate, uninterrupted, and consistent multiobject tracking using roadside light detection and range (LiDAR) technology. This article presents a lane-level and full-cycle multivehicle tracking (MVT) method that uses low-channel roadside LiDAR (LRLT). First, a lane-level map was created by analyzing multiple frames of traffic object detection results. Then, we introduced an association method based on a search process and a microscopic motion model, while considering the lane-level map as a constraint. The search process aims to identify all the previous tracks that might be linked to the target vehicle, while the microscopic motion model ensures finding a correct historical track for association. In addition, a detection optimization approach using the lane-level map was also proposed to enhance the tracking performance. Finally, we created a new roadside tracking dataset for experimental studies using Velodyne VLP-16 LiDAR sensors. The results showed that our algorithm achieved the highest accuracy and the strongest anti-occlusion performance compared with other popular algorithms such as simple online and realtime tracking (SORT), observation-centric SORT (OC-SORT), and ByteTrack. It is therefore a very suitable method for obtaining complete vehicle tracks using roadside LiDAR technology. The dataset and source code are available at https://github.com/moxigua/LMAT.
更多查看译文
关键词
lane-level,full-cycle,low-channel
AI 理解论文
溯源树
样例
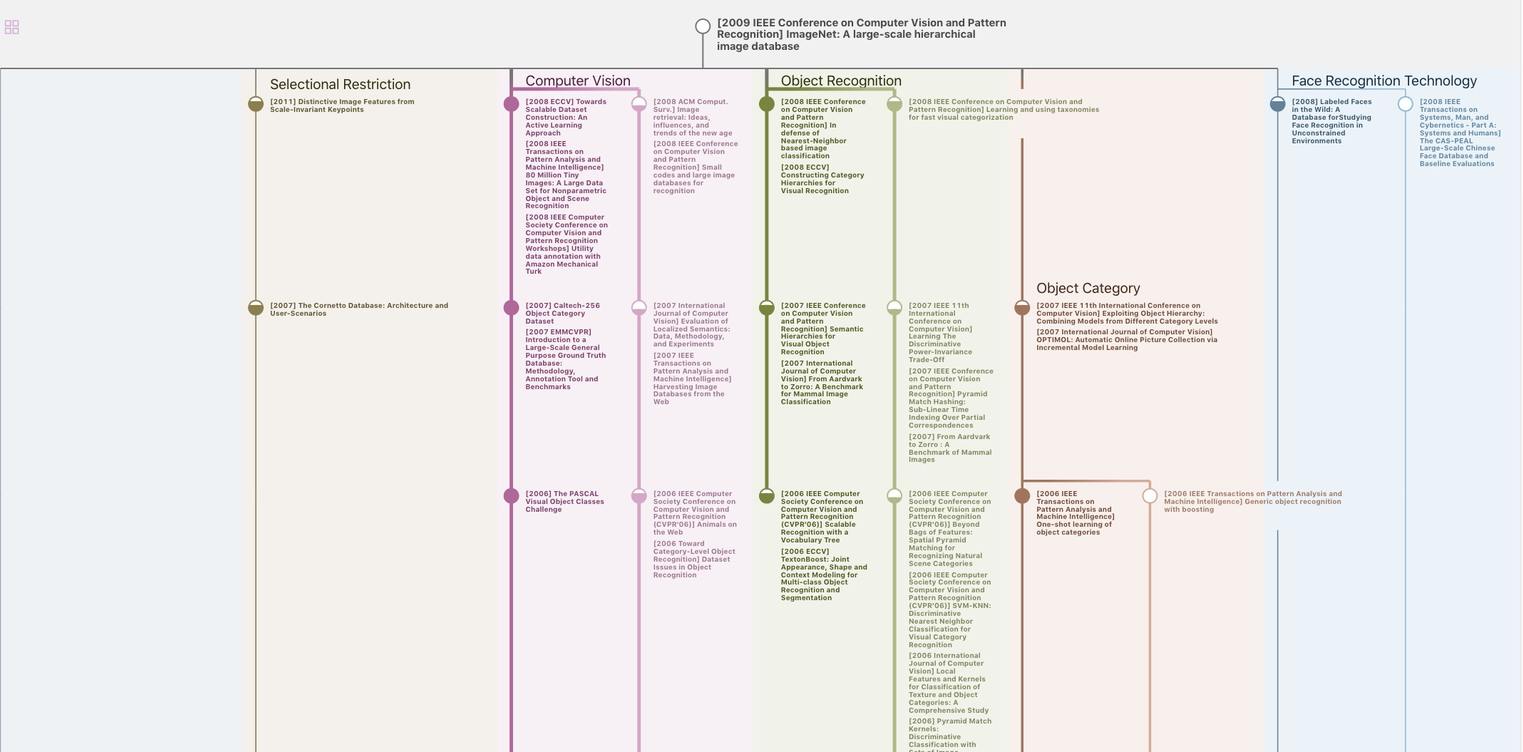
生成溯源树,研究论文发展脉络
Chat Paper
正在生成论文摘要