Tensor Singular Spectrum Analysis for 3-D Feature Extraction in Hyperspectral Images.
IEEE Trans. Geosci. Remote. Sens.(2023)
摘要
Due to the cubic structure of a hyperspectral image (HSI), how to characterize its spectral and spatial properties in 3-D is challenging. Conventional spectral-spatial methods usually extract spectral and spatial information separately, ignoring their intrinsic correlations. Recently, some 3-D feature extraction methods are developed for the extraction of spectral and spatial features simultaneously, although they rely on local spatial-spectral regions and thus ignore the global spectral similarity and spatial consistency. Meanwhile, some of these methods contain huge model parameters which require a large number of training samples. In this article, a novel tensor singular spectrum analysis method is proposed to extract global and low-rank features of HSI. In TensorSSA, an adaptive embedding operation is first proposed to construct a trajectory tensor corresponding to the entire HSI, which takes full advantage of the spatial similarity and improves the adequate representation of the global low-rank properties of the HSI. Moreover, the obtained trajectory tensor, which contains the global and local spatial and spectral information of the HSI, is decomposed by the tensor singular value decomposition (t-SVD) to explore its low-rank intrinsic features. Finally, the efficacy of the extracted features is evaluated using the accuracy of image classification with a support vector machine (SVM) classifier. Experimental results on three publicly available datasets have fully demonstrated the superiority of the proposed TensorSSA over a few state-of-the-art 2-D/3-D feature extraction and deep learning algorithms, even with a limited number of training samples.
更多查看译文
关键词
hyperspectral images,3d feature extraction,tensor
AI 理解论文
溯源树
样例
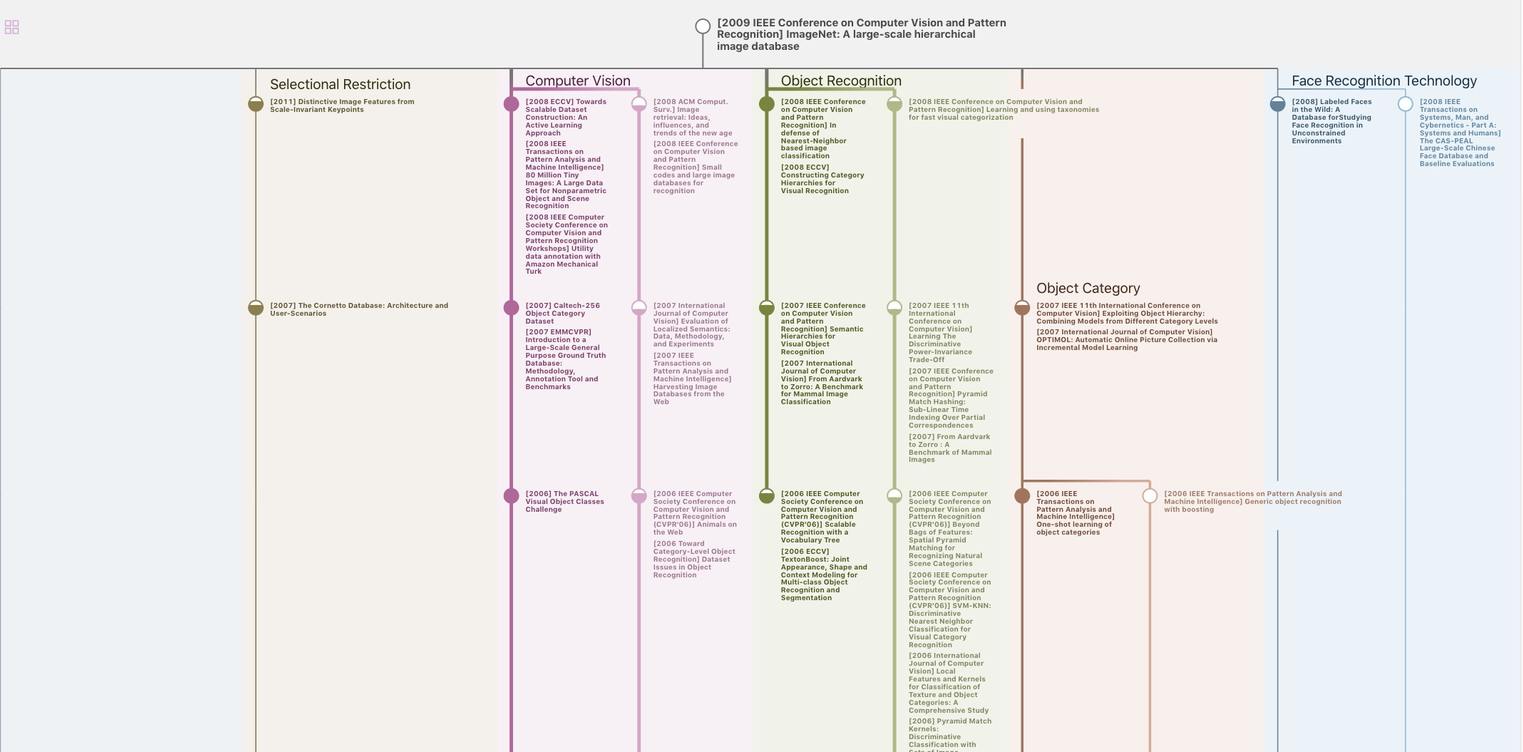
生成溯源树,研究论文发展脉络
Chat Paper
正在生成论文摘要